The Geneva FP&A Board convened on November 5, 2024, at the prestigious SPACES Quai de l’Ile, a charming venue in the heart of the city centre.
34 senior FP&A practitioners from leading global companies such as P&G, Cargill, Richemont, Novartis, Eaton, MSC, EA and many others attended the event to discuss a critical theme: Dynamic Shift – Mastering Predictive and Planning Forecasting.
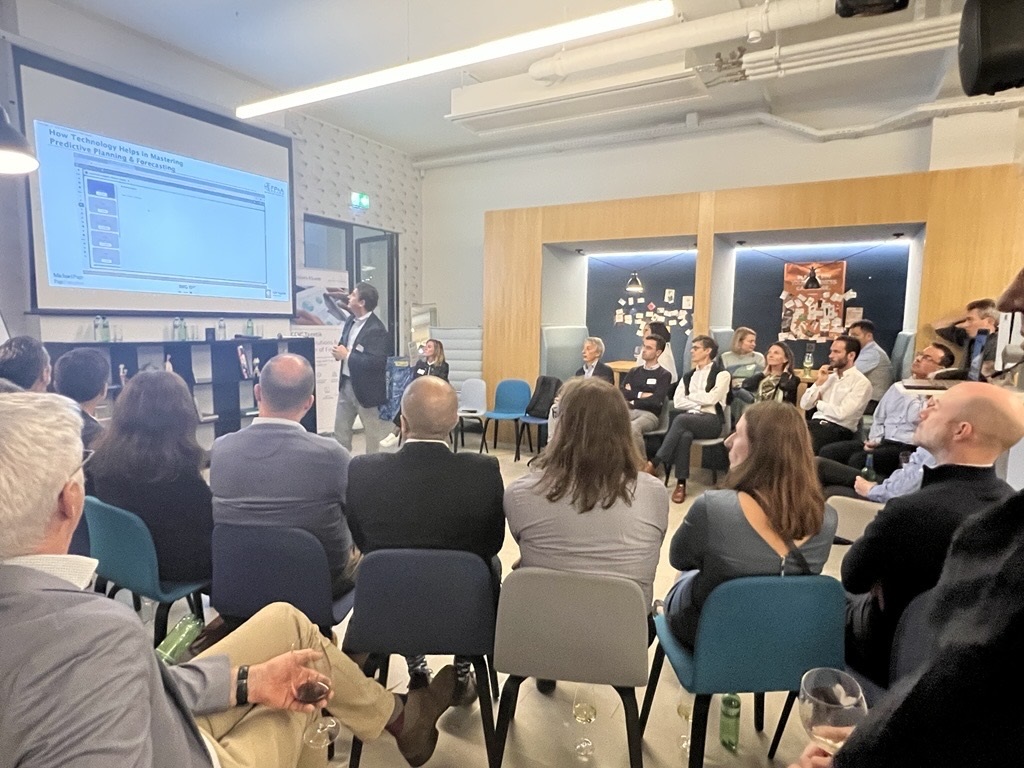
Figure 1: Geneva FP&A Board №17, November 2024
Larysa Melnychuk, CEO of FP&A Trends Group and Founder of the International FP&A Board, chaired the meeting, which was sponsored by Wolters Kluwer/CCH Tagetik in partnership with Michael Page and Page Executive, and International Workplace Group (IWG). This marked a key milestone for both PageGroup and IWG: the 150th global event with PageGroup and the 100th with IWG.
The event’s agenda covered a range of topics, from a theoretical discussion on the concept of Predictive Planning and Forecasting (PPF) to practical insights from field and small group discussions.
1. Evolution from Traditional to Predictive Planning and Forecasting (PPF)
Participants acknowledged that the FP&A community has been undergoing a paradigm shift from traditional to predictive forecasting.
To kick off the session, a brief ice-breaker was held, during which senior FP&A professionals shared the two key words they associate with the potential impact of PPF. Key insights focused on transparency, efficiency, flexibility, clarity, enhanced decision-making, resource deployment and simplification.
The group then proceeded to review the definition of PPF, which is shown in Figure 2 below.
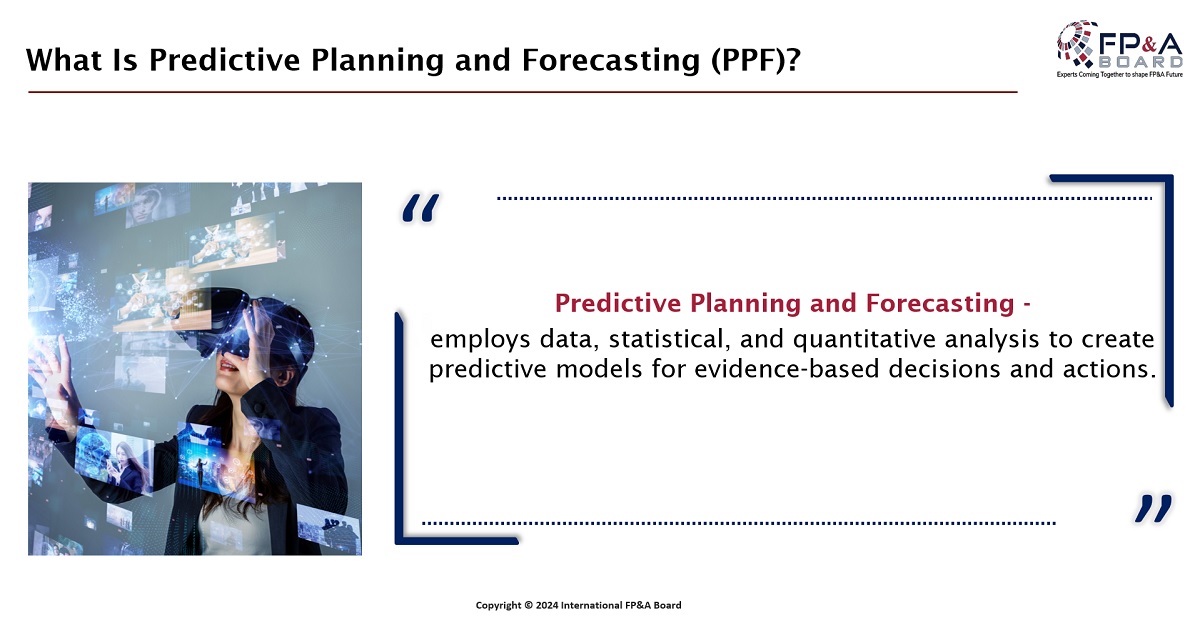
Figure 2
Despite the early stage of the discussion, Geneva FP&A Board members have already identified and agreed on two non-negotiable conditions for successful PPF implementation: data availability and driver identification.
Additionally, when considering the overall planning horizon, the forum recognised that traditional forecasting is limited to the Predictability Span (PS), while PPF, powered by Artificial Intelligence/Machine Learning (ML), currently stands as one of the solutions to manage uncertainty beyond the Predictability Span. Figure 3 illustrates this dichotomy, represented by the Uncertainty Cone.
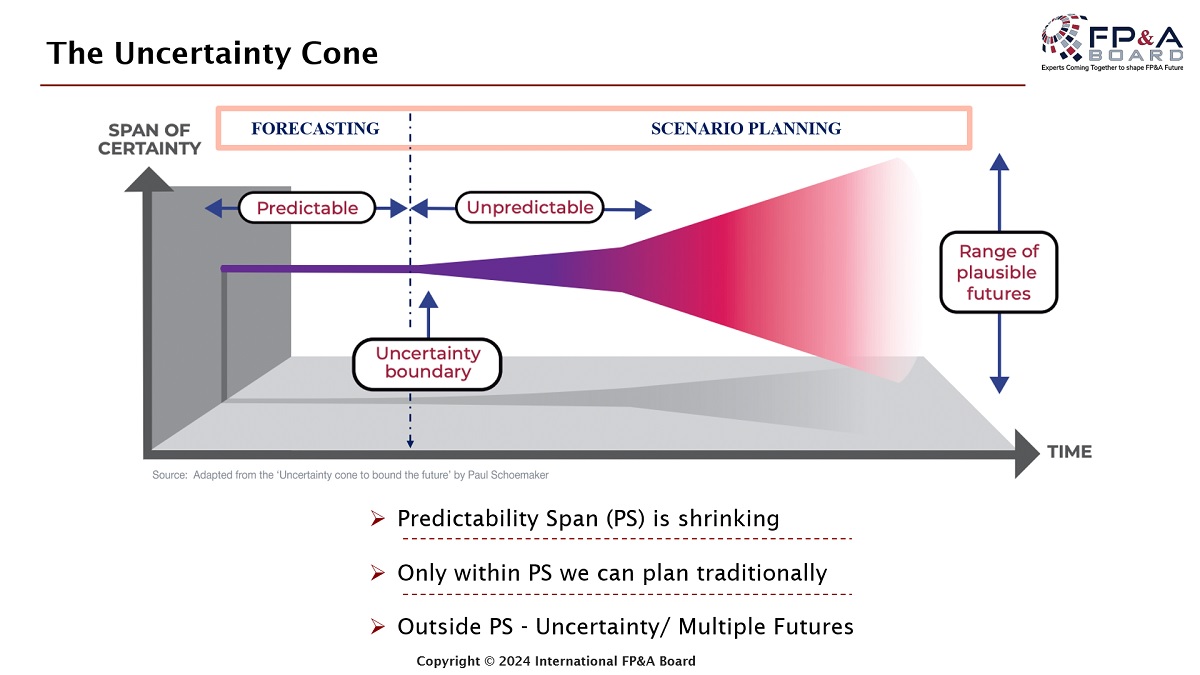
Figure 3
The group then reviewed the key structural differences between traditional forecasting and PPF, as clearly summarised in Figure 4 below.
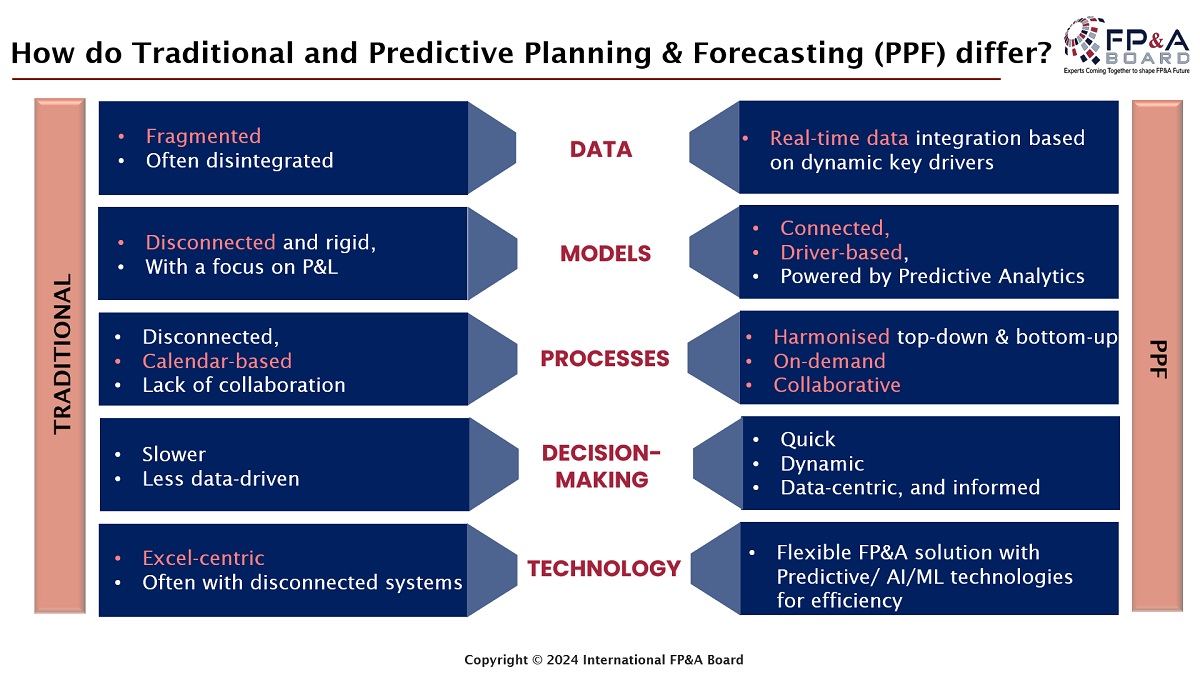
Figure 4
Armed with these theoretical but essential building blocks, we moved on to examine the key findings from the latest 2024 FP&A Trends Survey to assess progress on the journey toward PPF.
The survey analysis, complemented by insights from a recent McKinsey presentation, uncovered intriguing findings about agility, automation potential, time and resource allocation by activities, and driver and technology adoption.
- Agility: Measuring the time FP&A organisations take to run scenarios, the survey revealed that only 22% of respondents can run scenarios within a day.
- Automatability: According to a recent McKinsey study, nearly 50% of typical FP&A tasks are only partially or difficult to automate, compared to just 25% for accounting and financial reporting activities.
- Time and resource allocation: The 2024 FP&A Trends Survey unveiled that FP&A teams spend 45% of their time on data collection and validation, while 20% of their time is dedicated to information generation. This leaves only one-third of their time resources for higher-value activities, such as insight generation and driving actions.
- Drivers and technology adoption: Based on four key dimensions, such as the time spent on high-value activities, percentage of data-driven decisions, FP&A team performance, and forecast accuracy, the survey showed that FP&A organisations deploying driver-based models, AI/ML techniques, and cloud platforms in forecasting consistently outperform those that do not do so across all dimensions.
As a result, the Geneva FP&A Board members concluded that we should focus on these key six success factors to advance a more harmonised adoption of PPF (see Figure 5 below). These six pillars must be integrated both vertically and horizontally, with people as the driving force.
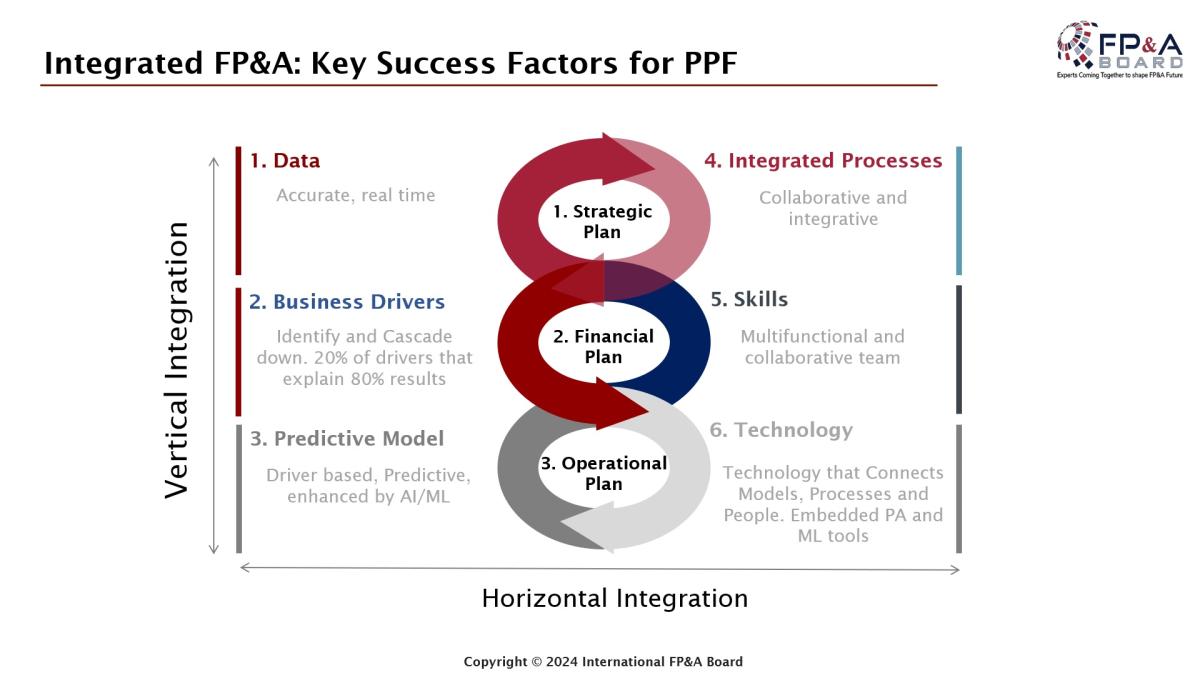
Figure 5
2. Balancing Act: Risks and Rewards of Predictive Planning and Forecasting
The Geneva FP&A Board members also briefly addressed the benefits and drawbacks of PPF to holistically assess both elements, as outlined in Figure 6 below.
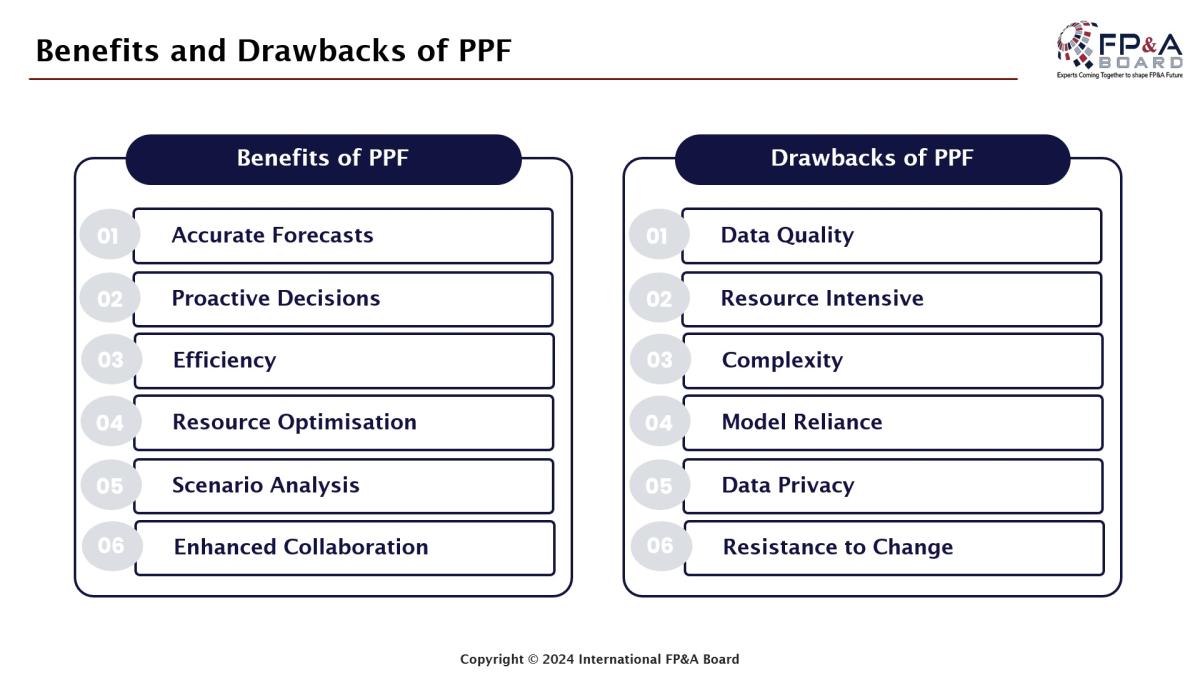
Figure 6
3. Key Elements of Predictive Planning and Forecasting Solution Framework
We also examined the Gartner Analytics Maturity Model, depicted in Figure 7 below. After that, we addressed how Predictive Analytics (PA) and Machine Learning (ML) could practically enhance PPF.
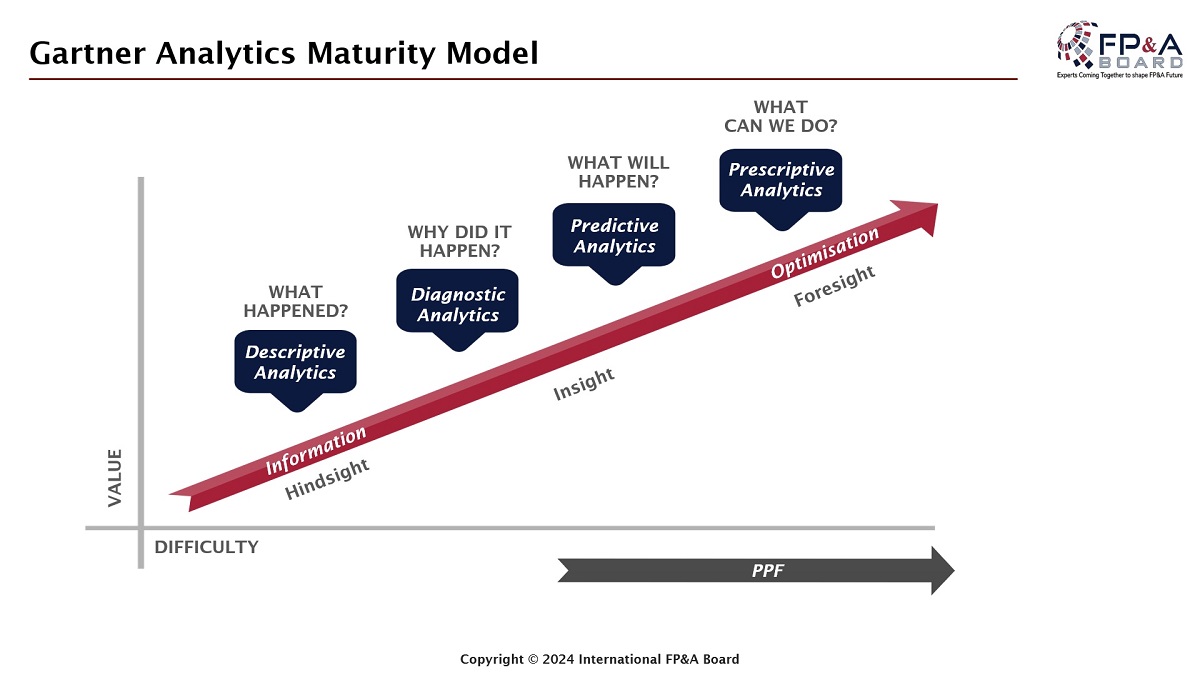
Figure 7
At first, we clarified the distinction between PA and ML, which lies in their scope, learning processes, and applications. PA relies on historical data to forecast future events or outcomes. Conversely, ML, a subset of Artificial Intelligence (AI), enables computer systems to learn from data and make predictions without explicit programming. However, both approaches depend on historical data, as portrayed in Figure 8 below.
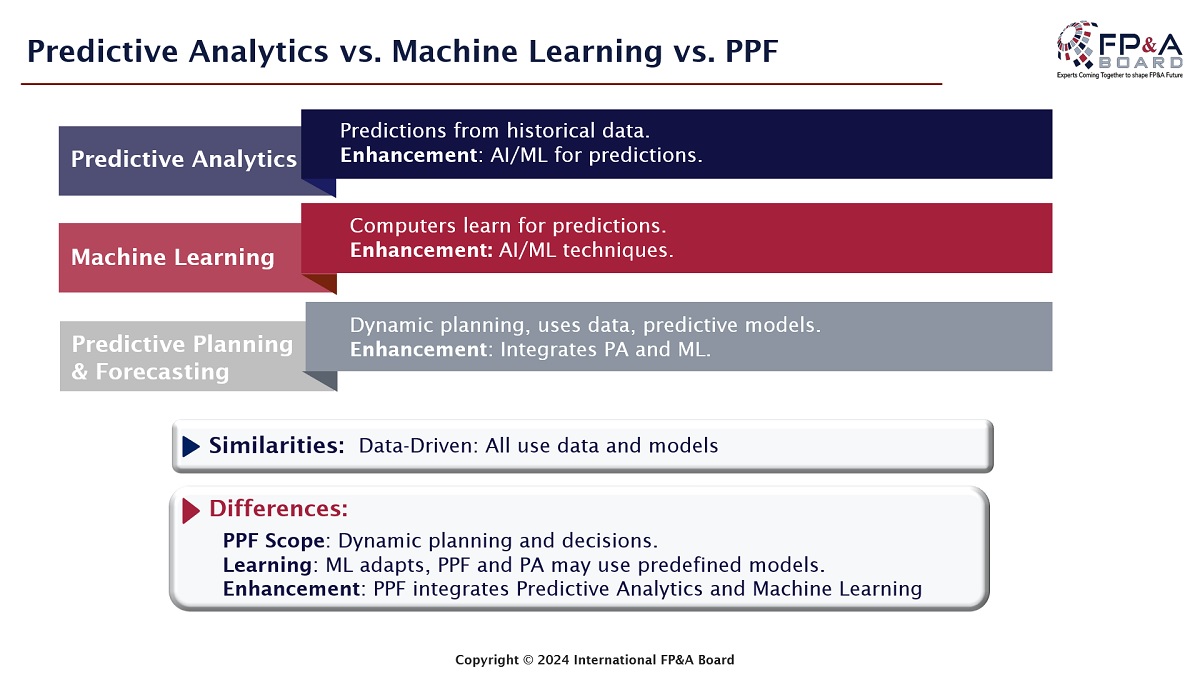
Figure 8
Geneva FP&A Board members subsequently wrapped up this section by concluding that an ideal FP&A team should be skilled in Prescriptive Analytics. It’s the area of PPF that not only forecasts future financial outcomes but also offers specific recommendations for optimising financial decisions. Furthermore, implementing ML would enhance the power of Predictive Analytics. ML can enable real-time recommendations and strengthen PPF through improved forecast accuracy and more efficient, data-driven decision-making.
4. Roadmap to Implementation: Navigating through the FP&A Trends PPF Maturity Model
Ahead of transitioning to the case study, we finalised the first part of the event with the FP&A Trends PPF Maturity Model, illustrated in Figure 9 below.
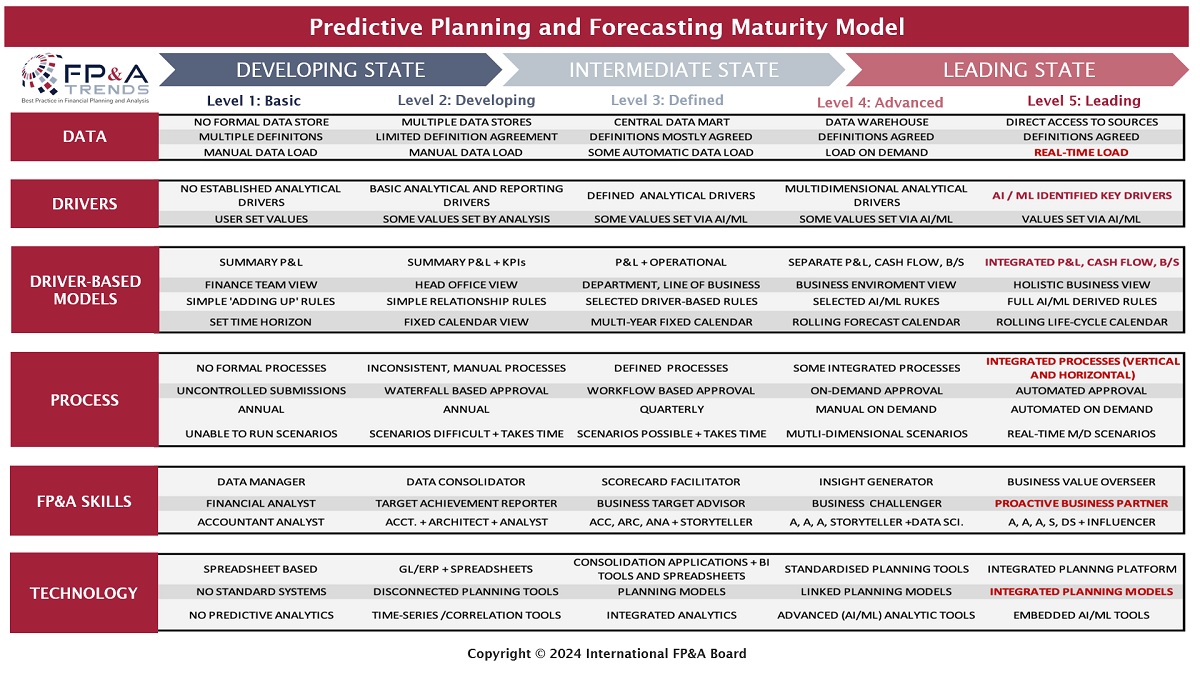
Figure 9
The FP&A Trends Predictive Planning and Forecasting Maturity Model illustrates the progression from a basic PPF to an advanced state, evaluated across six dimensions with five levels in each. The leading state signifies an organisation where planning and analysis contribute significant value, enabling the business to navigate an unpredictable future.
5. Practical Insights from the Field
Christine Fromont, CFO at Hôpital de la Tour, took the stage to present to the Geneva FP&A Board the key aspects of a successful AI/ML transformation project she had led at a major pharmaceutical corporation.
She introduced three core principles:
- AI Integration: Revolutionising traditional financial processes.
- Change Management: Crucial for successful adoption. Full alignment across the organisation is key.
- Tangible Results: The ability to deliver concrete improvements is essential for building trust, securing buy-in, ensuring alignment, and ultimately driving adoption.
The project focused on optimising the annual budgeting process. Before the initiative began, this process was cumbersome and inefficient, taking about nine months to complete. By leveraging innovative AI/ML solutions, the organisation was able to move to a much leaner and more effective process, which now takes only six weeks.
As for implementation, their strategy zeroed in on three key pillars:
- Targeted scope: Revenue and priority marketing expenses.
- AI Tools: Automated sales forecasting and marketing budgets.
- Data Infrastructure: A dedicated team in Barcelona for data and AI.
a. Data collection and preparation began 18 months prior to going live.
b. During this period, the AI model was refined through repetitive iterations.
c. Data cleansing was another critical step.
Christine Fromont emphasised that the successful go-live of the project and the subsequent adoption of the model were possible due to three key factors, as illustrated in Figure 10 below.
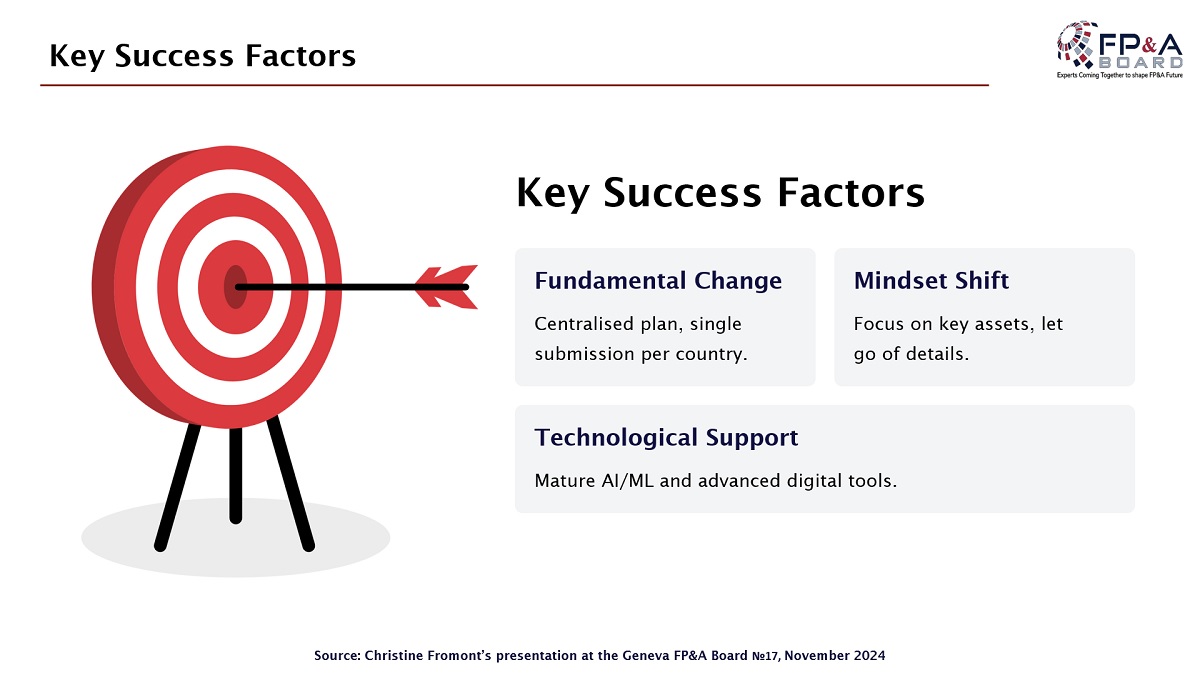
Figure 10
Finally, she concluded her presentation by sharing three key lessons for effective Change Management, which was one of the three pillars of this project (Figure 11):
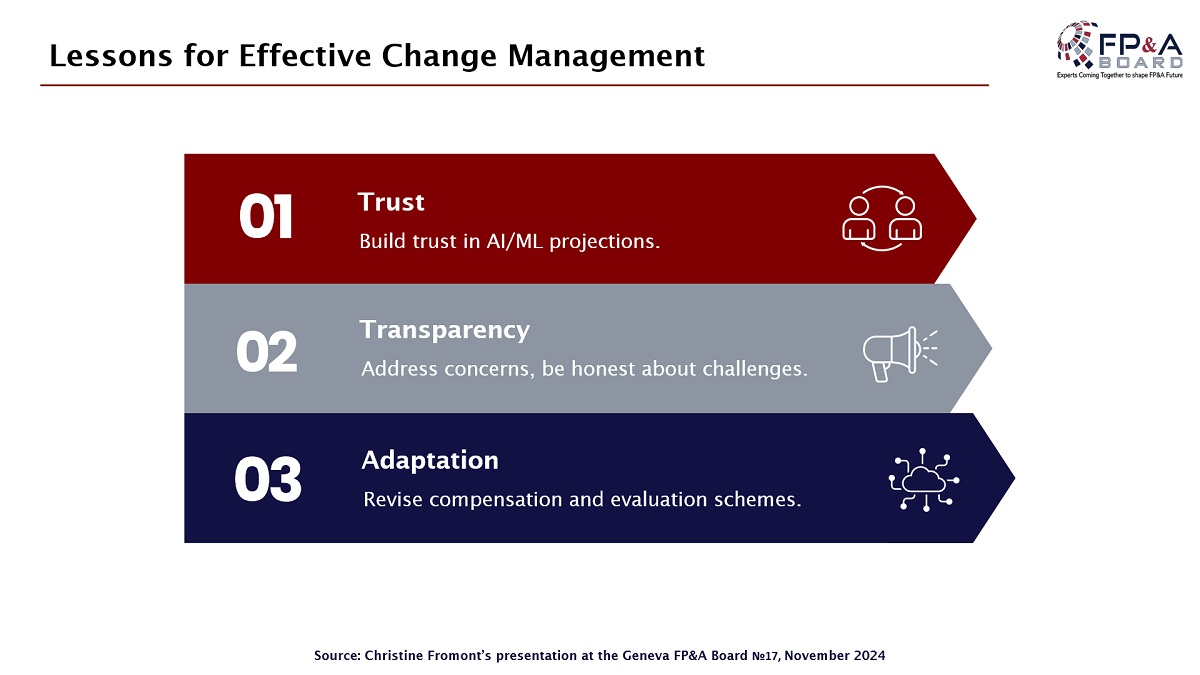
Figure 11
6. Group work, Conclusions and Recommendations
Lastly, the attendees were divided into three workgroups. Each group was tasked with contributing to a common objective: identifying the essential steps an organisation may take to reach the leading stage of Predictive Planning and Forecasting.
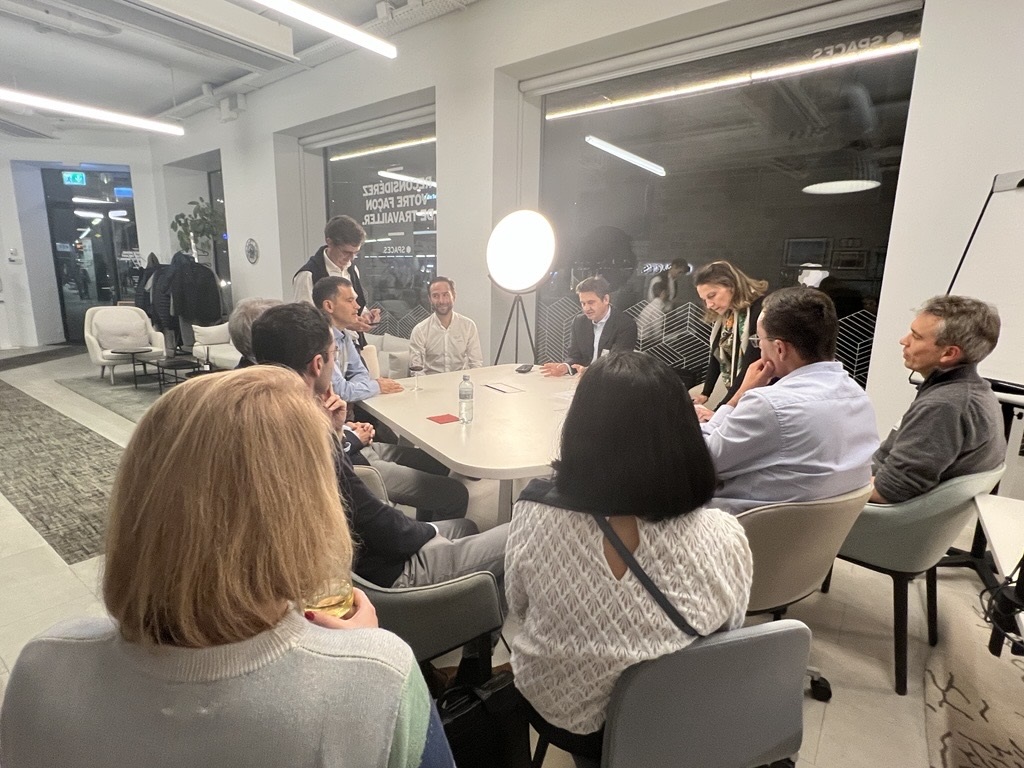
Figure 12: Group Work, Geneva FP&A Board, November 2024
To achieve this shared goal, each team was assigned a specific task and was then asked to present their conclusions and insights to the rest of the workshop.
Group 1: Dynamic driver-based model development
Task: Define the steps to create a dynamic driver-based model that enhances forecasting accuracy and adaptability.
Recommendations:
- Identify, test, communicate and explain drivers.
- Remove biases.
- Allow enough time to build data sources.
- Run the iterative process.
- Balance complexity and materiality (80/20 rule).
Group 2: Process Improvements
Task: Identify the process improvements required to make forecasting more agile and responsive.
Recommendations:
- Map out weaknesses of the as-is state.
- Strive for automatisation and standardisation.
- Buy-in within the organisation, as communication and collaboration are critical.
Group 3: Technology and Analytics
Task: Outline the technology and analytics capabilities necessary to support advanced Predictive Planning.
- Data input quality is as critical as output quality.
- Data connectivity.
- Data visualisation.
- Training and competencies development of the FP&A teams.
The meeting ended with a lively networking session.