In this video, Sebastian Poduch, Head of FP&A at Royal Schiphol Group, shares his experiences utilising...
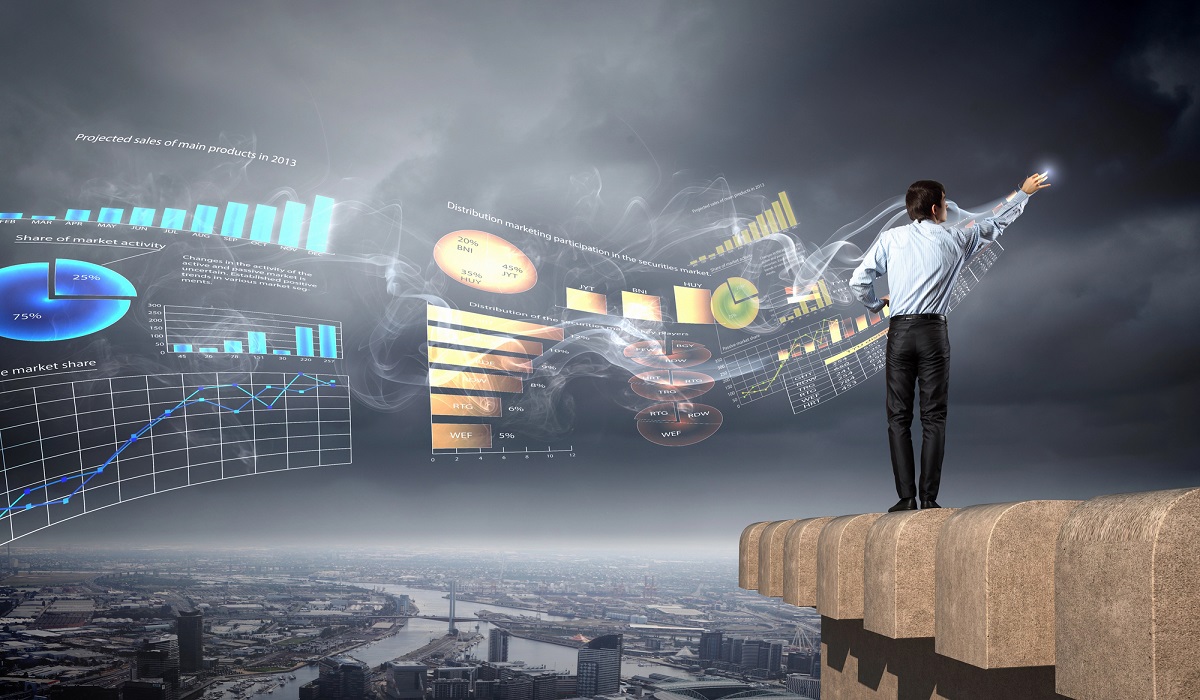
A Danish aphorism, “It’s difficult to make predictions, especially about the future”, will likely resonate with every FP&A professional. Even though we now have sophisticated tools, data, and technology at our disposal, the future remains inherently uncertain in a business landscape characterised by volatility, uncertainty, complexity, and ambiguity (“VUCA”). Accurate predictions still remain a significant challenge, yet businesses count on their FP&A teams to cut through the uncertainty and prepare robust plans and budgets aligned with the company’s strategic objectives. How can modern FP&A professionals overcome this problem?
Crystal Ball 2024: Predictive Planning and Forecasting (PPF)
Frustratingly for people working in FP&A, certainty about the future will forever be out of reach. However, we can anticipate future trends, demands and outcomes and help our organisations get ready for them. FP&A professionals can make better predictions about the future and prepare plans that optimise resource allocation factoring in macroeconomic landscapes by using a combination of historical data, statistical analysis and Predictive Analytics. Transitioning from traditional planning to Predictive Planning and Forecasting (PPF) is a critical but challenging step that FP&A teams should take to continue to add value to their organisations.
The potential for businesses to derive value from PPF spans across sectors. For example, retailers and fast-moving consumer goods companies can use predictive models to analyse historical sales data, seasonality, promotions and external factors such as holidays and weather forecasts to enhance their predictions about future demand for specific products or categories. Improvements in demand forecasting will allow them to reduce stockouts and optimise their inventory management across supply chains.
Alternatively, in manufacturing or utilities, using sensors to collect data from machinery or infrastructure to provide real-time inputs to predictive models can help predict when components may fail. It can be used to reduce downtime or failures by ensuring timely maintenance and to provide cost savings through more efficient maintenance resource allocation.
These are just two examples of the benefits that can be unlocked with Predictive Planning and Forecasting, which has numerous potential use cases across other businesses and sectors. However, all organisations seeking to implement PPF face a common challenge: data quality.
Game of Data: A Song of Accuracy and Predictions
One of the most significant challenges in developing Predictive Planning and Forecasting is ensuring that an organisation has a data governance framework that can support the data quality requirements for this approach. Since the early days of modern computer science, “garbage in, garbage out” and equivalent phrases have succinctly described that poor input can only lead to similarly poor output. The same remains true today, especially when we rely on Artificial Intelligence (AI)/Machine Learning (ML) techniques as the key components of predictive capabilities. Multiple elements are required to achieve and maintain proper data quality and enable PPF, including already familiar and new challenges:
- Accuracy is, as it always has been, pivotal to reasonable predictions and decision-making;
- Completeness is another familiar challenge which can lead to gaps in analysis and ineffective predictive models unless addressed;
- Consistency challenges are heightened as data from disparate sources is required to enhance the accuracy of predictive models;
- Timeliness is increasingly important as data can change rapidly, and outdated data can significantly impair forecasting accuracy; and
- Relevance is a newer consideration that requires organisations to identify appropriate data to be incorporated into their predictive models in line with their goals to avoid skewing predictions with irrelevant data and wasting computational resources.
Further, there are associated technological and methodological considerations regarding the selection of appropriate ML algorithms for data analysis, large dataset management, and the integration of multiple data sources.
Overcoming the Challenges
The good news is that the solutions required for managing data integrity effectively are well established, widely known and increasingly manageable for businesses to deploy well with better technological enablement. FP&A can already support data quality management by performing data audits. For example, at a major retailer, the FP&A team regularly audits inventory data, which helps strengthen data quality practices. By doing so, they also perform their core role better by improving forecast accuracy, driven by enhanced data accuracy and enabling decision makers to optimise inventory levels through savvier inventory management decisions.
The less positive news is that implementing strong data management remains a challenge due to a variety of factors, such as outlined below:
- the extremely large and growing data volumes to be managed,
- legacy systems that don’t support modern data quality practices or, worse, reliance on spreadsheets and
- persistent data silos when different departments store data independently or in different systems.
Putting the right data foundation in place is a critical prerequisite for enabling Predictive Planning and Forecasting and other leading FP&A practices. Developing a robust data governance framework to support advanced analytical capabilities requires a particular focus on the technology and data/enterprise architecture components of the framework to deliver the following:
- Data validation techniques such as type, format, length and presence checks to ensure data consistency, adherence to expected formats and have been entered into key database fields;
- Error detection to identify data impairments that arose during data transmission through, for example, checksums and two-dimensional parity checks;
- Data imputation methods, which may include missing value prediction, average interpolation or moving average techniques to address and compensate for any gaps in the data;
- Data harmonisation to create a composite dataset with common data fields, formats and dimensions from disparate data sources;
- Creating a modern data-streaming architecture that enables the ingestion of live streaming data, provides for its cleansing, transformation and analysis and can be combined with structured data from data warehouses; and
- Data selection by domain experts to ensure that only the most relevant to predictive model objectives data points and Key Performance Indicators (KPIs) are provided as model inputs.
Although these capabilities directly remedy the data quality issues outlined previously, they will not adequately safeguard data quality in the long term if the rest of the data governance framework is underdeveloped. Therefore, ensuring that adequate roles and responsibilities relating to data governance are identified and constituted in the organisation remains just as important as ever. We should also establish governance bodies like a data governance committee to oversee policies and decisions and check whether sufficient directives and policies are developed and data quality metrics are defined and monitored regularly.
Getting Ready for Enhanced Insight
For FP&A, it can be tempting to see data governance as an upstream process performed elsewhere by other people in the business that provides input for our analytical work. However, it’s a critical prerequisite for unlocking the value of Predictive Planning and Forecasting. We can’t afford to sit on the sidelines. So, here are five ways FP&A professionals can support data quality improvement in their organisation:
- Articulate clear data requirements so that the data required for PPF and other FP&A activities (e.g. fields, type, format and frequency) can be delivered;
- Collaborate cross-functionally, particularly with IT and data management teams, to support the required delivery and manage changes to requirements;
- Define suitable data ownership and data management roles for FP&A focused on data analysis;
- Work closely with other data owners to ensure that their data adequately supports FP&A analytical processes; and
- Champion data management initiatives to embed leading practices in the organisation so FP&A has a solid foundation for developing advanced capabilities.
Effective data governance will enable us to make better predictions and plans for our organisations. FP&A teams should seize this opportunity to support robust practices and enhance the value we deliver to the business.