This article will explore the challenges faced by finance departments today regarding deviations and anomaly detection...
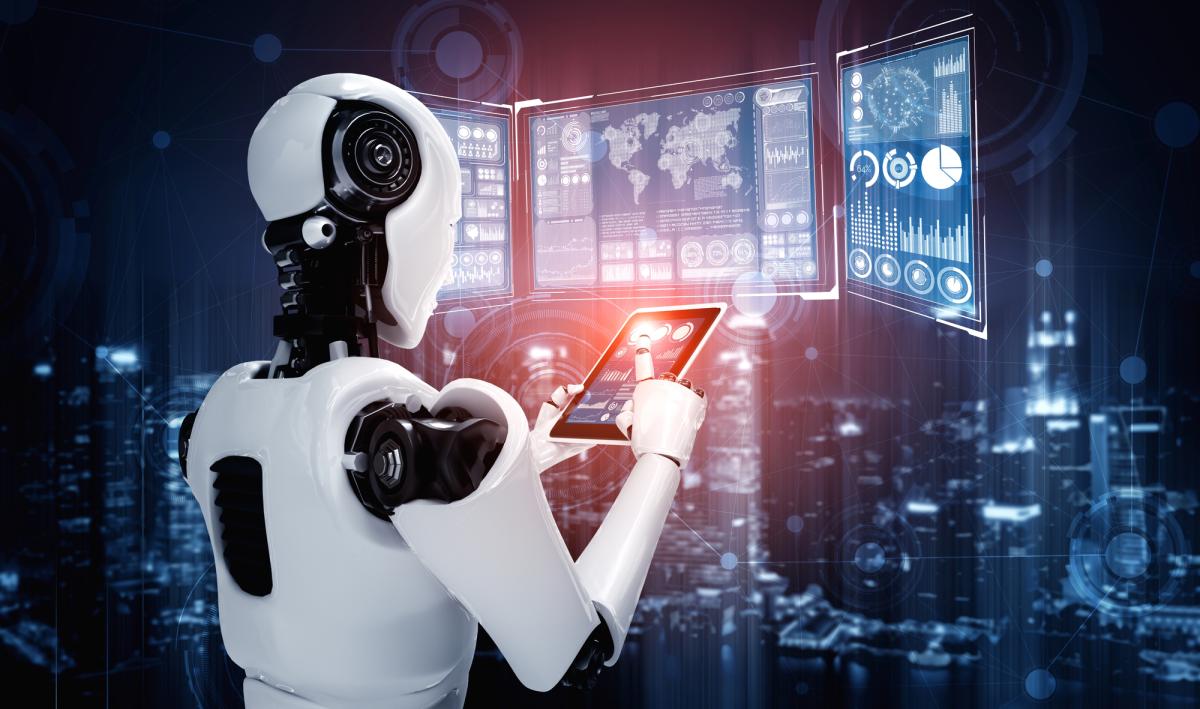
In Part 1, we examined how AI is reshaping financial close, and in Part 2, we tackled the biggest challenges accounting managers face. Now, in Part 3, we focus on practical steps to successfully implement AI-driven anomaly detection.
Challenges and Opportunities with Automated Anomaly Detection
During our in-depth interviews with five senior finance managers and CFOs, we identified challenges and opportunities related to how automation and Artificial Intelligence (AI) can enhance anomaly detection within financial data. The finance managers emphasised the need for a shift from the current manual processes to a more automated future, but they also highlighted specific technical and organisational barriers that need to be overcome.
To understand the role of AI in anomaly detection, it is important to first examine how finance teams currently manage this process and the challenges they face.
Current Practices and Challenges
Currently, manual reviews and processes dominate anomaly detection. The finance managers mentioned that their teams often spend much time manually reviewing transaction data to identify anomalies, which is time-consuming and inefficient. This approach leads to missed errors and delays critical decisions that could have been made earlier with better tools. Several interviewees pointed out that the company’s ability to detect anomalies often depends on individual employees’ experience rather than systematic processes, creating inconsistencies and risks.
Anomaly Detection: From Manual to Fully Automated Process
The finance managers acknowledge that we are in the early stages of transitioning from manual reviews to semi-automated anomaly detection with the potential of reaching fully automated solutions in the long-term. Many companies still rely on manual methods for identifying anomalies in financial data, often resulting in delays and mistakes. At the same time, they recognise that implementing AI-based systems that not only detect anomalies but also learn from historical data to predict future risks is a complex process.
Most interviewees underscored the need to invest in systems that can evolve over time and adapt to the company’s needs. Finance managers perceive AI and Machine Learning as key components for the future, as these tools can identify direct anomalies and create pattern recognition that provides deeper insights into potential risk areas before they escalate.
Strategic Advantages of AI-Based Anomaly Detection
In the long term, CFOs and finance managers see that automated anomaly detection can contribute to more proactive and informed business decisions. By automating large parts of the manual data review, teams can free up time to focus on more strategic analyses and decisions. It means that AI systems would not only help correct current errors but also provide predictions about future anomalies, enabling better risk management and financial stability.
Several interviewees stressed that AI’s ability to provide Predictive Analytics would be crucial for identifying early warning signs in financial data and preventing potential risks long before they escalate. This would allow a shift from a reactive to a proactive strategy for financial functions, significantly improving business planning and risk management.
Technical Barriers and Organisational Challenges
Despite the significant potential of AI and automation, the interviewees identified several technical and organisational barriers that may limit the implementation of these systems. A central challenge is that many of the existing business systems companies use today are incompatible with more advanced AI tools. This creates integration issues, where new systems often need to be developed or adapted to work in the existing infrastructure.
Other major challenges are data security and integrity. Several finance managers expressed concerns about how AI systems handle sensitive financial information, the need for robust security protocols and compliance with current regulations, such as GDPR. This means that any AI tool must be well-integrated and have access to secure and reliable data to deliver valuable insights.
At an organisational level, companies also need to invest in training and skill development to ensure employees have a sufficient understanding of AI and automation. Many finance managers emphasised that the shift to automated systems requires changes in culture and competencies, where teams must have the ability to understand and use the new tools to maximise their potential.
Despite these challenges, finance leaders agree that the key to success lies in scalable and adaptable AI solutions.
Scalability and Adaptability – Keys to Success
One of the most prominent insights from the interviews was that future AI-based anomaly detection tools must be scalable and adaptable. It highlights the importance of investing in systems that can be adjusted according to changing business models and specific industry requirements.
The New Finance Role – A Centaur in Reality
A common insight shared by all the interviewees was that the future finance role would undergo a significant transformation. Finance professionals will need to combine their traditional financial expertise with technical skills in AI and automation. This hybrid role, akin to a centaur representing finance and technology, will be central to driving progress forward.
Finance professionals of the future will be expected to not only manage financial analysis but also have a deep understanding of the technical systems used to automate processes. It requires a shift in how companies view training and skill development within the finance function, where technical expertise becomes as vital as traditional accounting knowledge.
The interviews with finance managers and CFOs highlighted a clear necessity to develop and implement AI-based systems for anomaly detection. The companies that successfully achieve this transition first will be able to manage their financial processes more efficiently and improve their ability to predict and handle future risks.
The new hybrid role that combines financial expertise and technical competence will be crucial in leading this change. Companies that invest in these systems and train their finance professionals to meet these new requirements will stand strong in an increasingly automated and digitalised future.
As the finance role evolves, organisations must rethink their approach to AI adoption and anomaly detection. The next step is understanding how to implement these solutions effectively.
The Road Ahead: Implementing AI for Smarter Anomaly Detection
Throughout this series of articles, we have explored how finance departments today struggle with managing anomalies in transactional data and financial reports, often through time-consuming manual processes. Automation and AI offer solutions that can streamline this process, but many companies have not fully embraced these technologies yet. Anomaly detection is one of the most critical aspects of modern financial management, and the successful implementation of AI-based tools can make a significant difference in ensuring data quality and financial stability.
Automation as a Solution for Anomaly Detection
Both surveys and interviews indicate that today’s finance departments still rely on manual processes to identify anomalies. This is inefficient, particularly as data volumes increase. Automation and AI can alleviate these processes by quickly and efficiently identifying anomalies based on historical data and real-time patterns.
Automated anomaly detection is not just a way to reduce the manual workload; it also provides the ability to analyse larger data sets and detect subtle anomalies that might otherwise go unnoticed. Systems can improve their ability to identify and predict anomalies via ML, enhancing financial reporting and ensuring accurate information for decision-making.
Flexible and Scalable Systems
Interviews with finance managers and CFOs have shown that future systems for anomaly detection must be flexible and adaptable. Each organisation has unique requirements depending on its business model, industry and size. The AI systems implemented must be scalable and modular to cater to different companies. It means that organisations can adjust their systems based on transaction volumes, data complexity and specific business needs.
Visual Data Analysis and Dashboards for More Efficient Monitoring
A recurring request from the interviewees was that AI tools for anomaly detection should have robust visualisation capabilities. By presenting anomalies in interactive dashboards, finance departments can quickly identify and act upon potential errors. Data visualisation allows finance professionals to quickly gain an overview of the company’s financial health and identify areas requiring further review.
Challenges and Technical Barriers
Despite the great benefits of automation, several technical obstacles were also identified. A common issue is that many companies use legacy systems that are incompatible with modern AI tools. It can create bottlenecks in data processing and complicate the implementation of automated systems. Additionally, finance managers pointed out that companies need sufficient technical maturity to fully benefit from these tools.
Overcoming these challenges requires a structured approach to AI adoption. Here’s how organisations can successfully implement AI-based anomaly detection.
Recommendations for Implementing AI-Based Anomaly Detection
1. Gradual Implementation of AI-Based Anomaly Detection
Companies should follow a structured approach to introduce AI solutions in a controlled and scalable manner, adapted to the company’s size and technical maturity:
- Step 1: Current State Analysis
Identify and map current processes to pinpoint areas where manual tasks are most time-consuming, error-prone or inefficient. This analysis forms the basis for prioritising the right areas for automation and ensures that the company starts with processes that provide the greatest business value. - Step 2: Choose an AI Solution Tailored to the Company’s Needs
The choice of AI solution should consider the company’s size, complexity and technical capacity. Small and medium-sized enterprises can start with simpler, semi-automated tools that help detect anomalies without requiring extensive system changes. Larger companies with more complex business models and larger data volumes can consider more advanced systems offering full automation and integrated analysis. - Step 3: Conduct a Pilot Project and Scale Up
To minimise risks and ensure smooth implementation, companies should start with a limited pilot project within a department or a specific cost centre. This allows testing the AI solution, evaluating results and adjusting the system before a broader rollout. Once the pilot project is validated, the solution can be scaled up to
2. Integration with Existing Systems
For the AI solution to function effectively, seamless integration with existing ERP, accounting, and business systems is required. Companies should prioritise AI tools that can integrate through modern interfaces (APIs) to avoid costly and extensive system replacements. Companies can gradually implement AI without disrupting existing systems by investing in compatible solutions, enabling a smoother transition to automation.
3. Training and Change Management for Long-Term Success
It is important to invest in training and skill development to ensure the AI solution is used effectively. Controllers, finance managers, and other finance personnel need relevant training to understand how the AI system works and how it can detect and address anomalies. Additionally, Change Management is crucial for integrating AI solutions into daily operations and ensuring that staff adapts to new workflows.
4. User-Friendly and Visualisation-Focused Systems
User-friendliness is a critical factor for maximising the benefits of AI solutions. AI tools should offer clear visualisation features, such as interactive dashboards, to make it easy for finance personnel to quickly identify and understand anomalies in real-time. This aids in improving decision-making and faster responses to potential issues.
Following this structured approach, companies can gradually adapt to new systems and processes without disrupting existing workflows. Smooth integration, clear training efforts, and Change Management are key to successful AI implementation. At the same time, companies must ensure that the systems are user-friendly and easy to use to create a sustainable and scalable solution for future growth.
Conclusions on Business Value with AI-Based Anomaly Detection
1. Efficient Management of Large Data Volumes and Ensuring Accurate Financial Reporting
AI-based solutions will play a central role in future finance departments by automating the identification of anomalies in real time. By using AI to manage large data volumes, companies can ensure that their financial reporting is accurate and reliable. It will enhance operational efficiency and reduce the manual workload.
2. Predictive Analysis for Future Risks and Strategic Decision-Making
AI offers real-time detection and Predictive Analysis, enabling companies to anticipate future risks and opportunities based on historical data. This capability strengthens strategic decision-making and allows them to act proactively to reduce future financial risks.
3. Investment in the Right Technology and Training for Sustainability and Competitive Advantage
To maximise the benefits of AI-based anomaly detection, companies must invest in the right technology and training while ensuring their systems are compatible with AI solutions. Companies that invest early in automated anomaly detection will reduce errors and create a stronger and more sustainable financial structure for the future.
4. The Future Hybrid Role for Finance Professionals and the Impact of Automation
The role of finance professionals will evolve into a hybrid one where financial expertise is combined with technical skills in AI, Machine Learning, and programming, such as Python. Semi-automated solutions will become standard in most companies, while fully automated processes will dominate businesses with high digital maturity. This “centaur role” enables finance professionals to not only make their work more efficient but also drive the development of systems for automated anomaly analysis, making them key players in future finance departments.