In this article, the author explains how Artificial Intelligence (AI) can help FP&A professionals become invaluable...
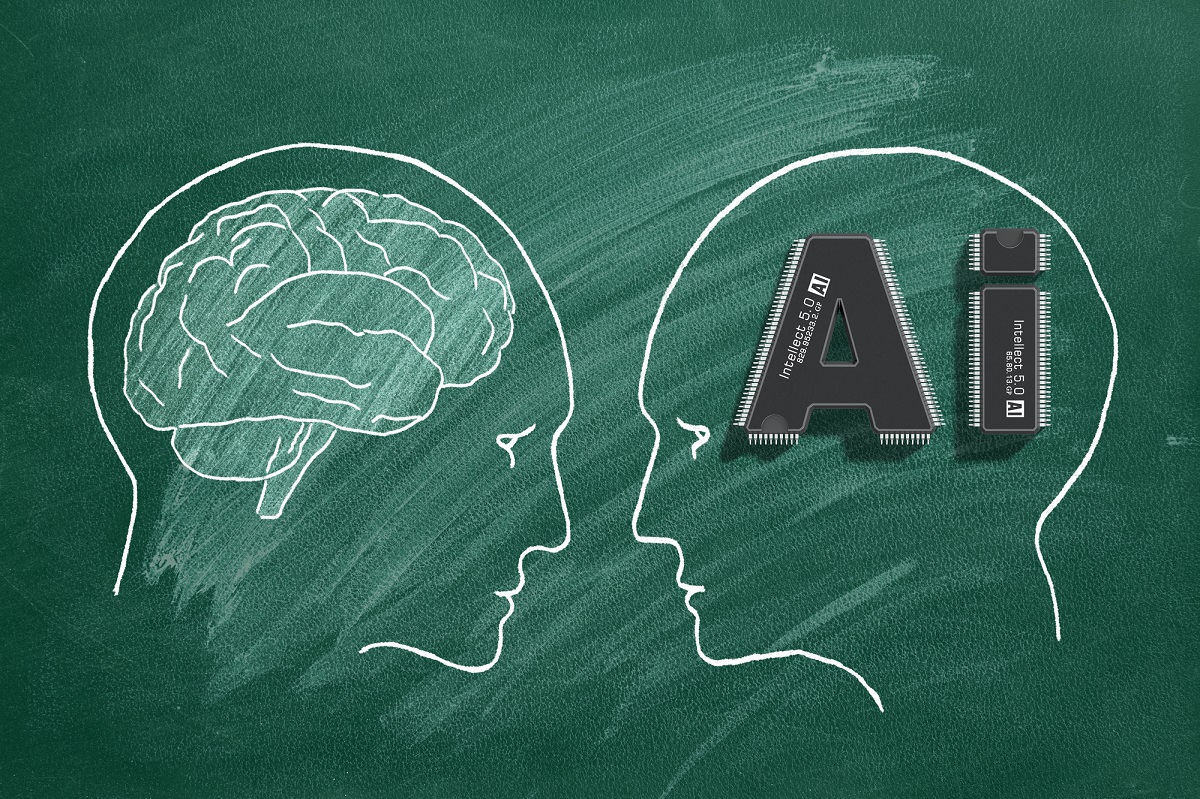
Advocates of Artificial Intelligence (AI) promise that it will bring impressive breakthroughs across industries, societies, and standards of living. We have already seen evidence of its impact on the Financial Planning & Analysis (FP&A) domain through improved analyses and evolved practices over the last two decades.
In this article, we will explore the possibilities of human and AI interaction, describe some practical examples, review considerations about responsible use of and value generation with AI, and end with practical references about upskilling and adoption.
First, Let’s Clarify a Few Ideas
AI is a term encompassing methods and techniques to handle data and provide solutions that seem to be human. Data Science, Machine Learning (ML), and Deep Learning are all embedded in AI. Overall, these methods and techniques fall into major categories:
- supervised learning, where the relationships between the input and output are known and, thus, the system learns how to respond to queries;
- unsupervised learning, where there is no prior knowledge of the data, so the system detects patterns in them;
- and “other learning”, where the system combines the above and learns without explicit programming instructions.
The latter is the domain of the so-called “Deep Learning,” which is built with neural networks that can handle all sorts of data types, such as text, video, voice, etc., out of foundation models1 (Figure 1).
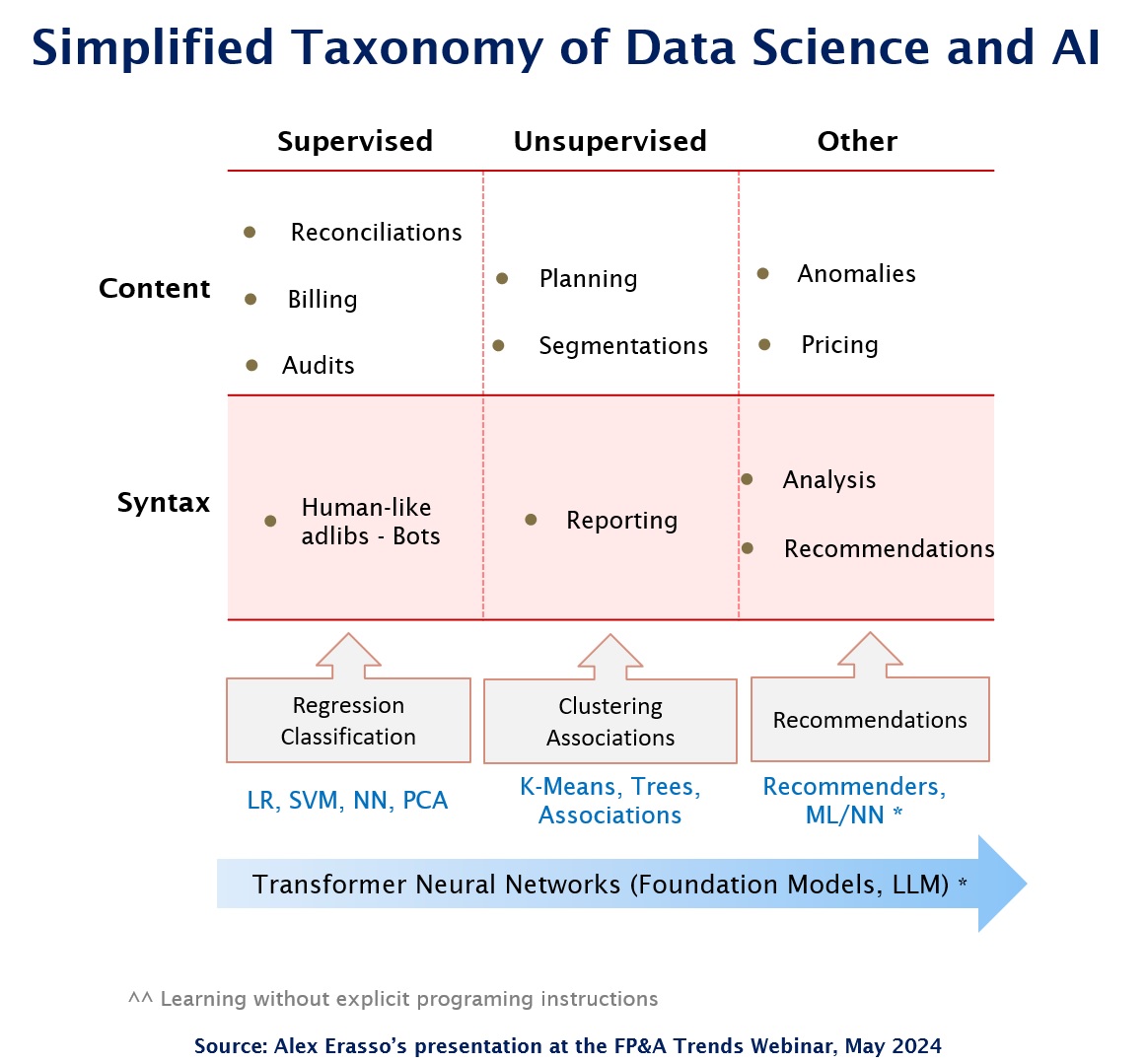
Figure 1
Earlier phases of AI development focused on raw content, enabling insights using Data Science and Machine Learning. The advent of Deep Learning allowed systems to address the syntax of the information, thereby producing “human-like language responses,” among other results2. However, we should remember that these systems do not have human-like thinking, and often, their inner workings are a “black box.”3
When we work in FP&A, we report results, plan operations, build analyses, provide observations and recommendations to Business Partners, and make decisions. AI is already helping us with data collection, data cleaning, analysis and content generation through Robotic Process Automation (RPA) (Figure 2).
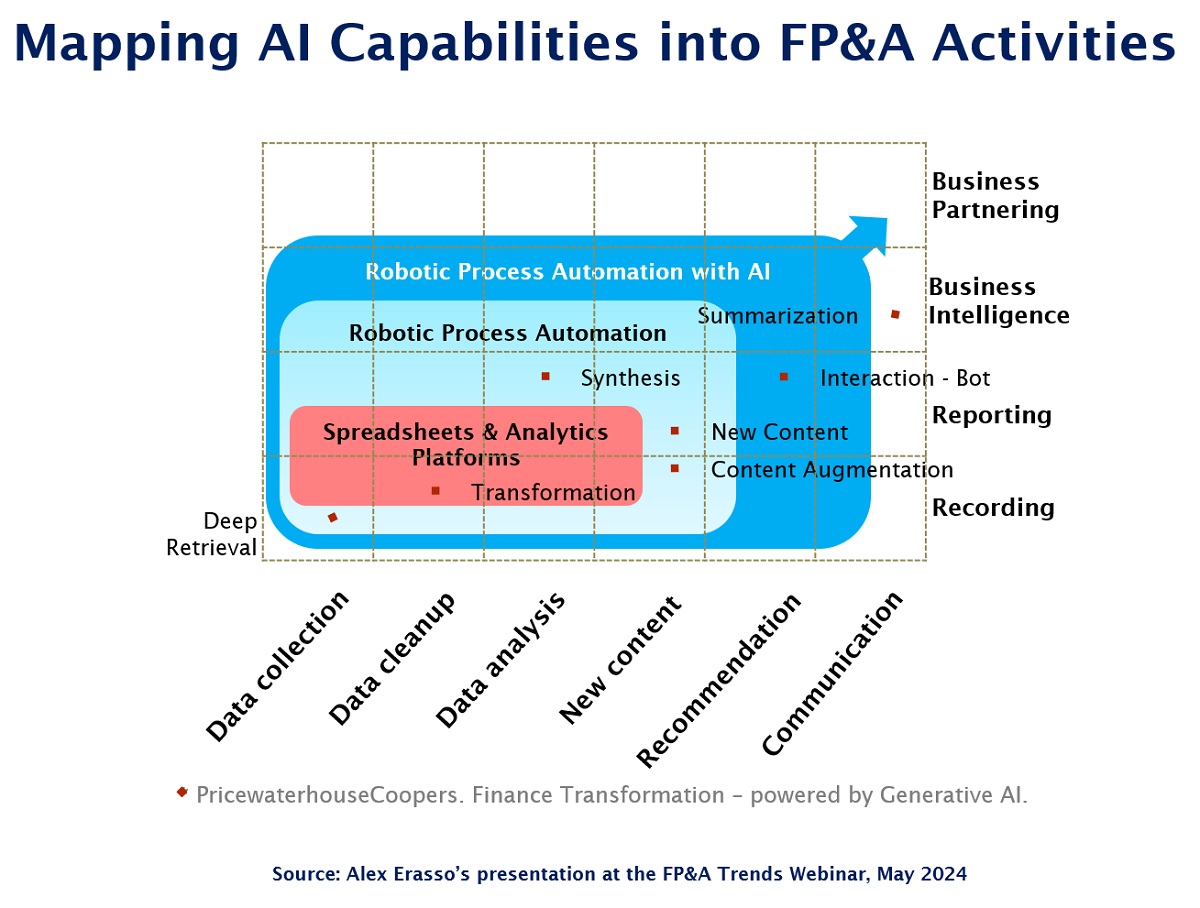
Figure 2
Some successful use cases of AI usage in FP&A by leading firms in the technology industry include (but are not limited to):
- In planning, the simulation (generation) of scenarios for operating plans.
- In analysis, market basket analysis of simultaneous or sequential purchases, segmentation of customers by buying behaviour and channel partners by profitability.
- In reporting, automated forecasting of expenses and booking levels and summarising the results of simple operations for presentations.
- In auditing, anomaly detection in quoting and purchasing that allows us to spot suspicious transactions.
- In functional activities supervised by FP&A: recommending optimal pricing for sales and product management; increasing productivity in manufacturing, customer service and sales; optimising shipping costs and reducing delivery times for logistics.
The initial impact of AI on FP&A practice is expected to be noticed in the product, go-to-market, and operational areas of the business4. For instance, quantifying production patterns or customers’ behaviour goes beyond streamlining processes. AI methods provide intelligence for smarter operations and competitive advantage for the financial benefit of companies. FP&A workflows are the means to understand those operations, their benefits, and the challenges that enable them.
Examples of AI in Practice Today
Now, let’s discuss practical examples of customer value and profitability at a global software firm with over $13B in annual sales (Figure 3).
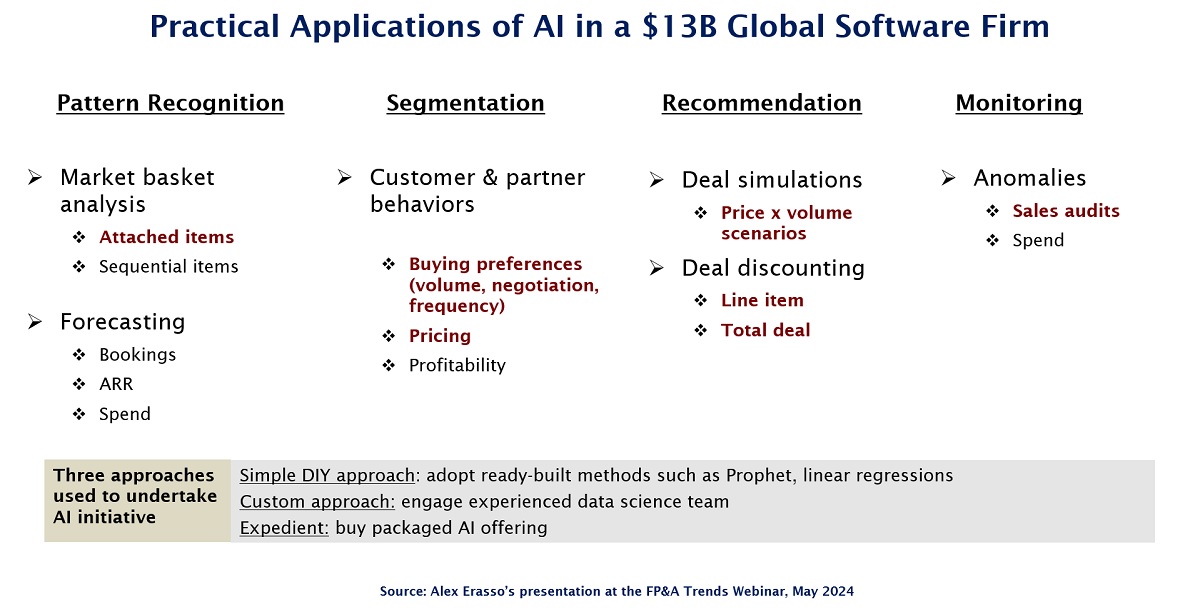
Figure 3
Traditional analysis of sales transactions looking for attached rates of products sold together (clustering and associations) was expanded to understand the buying preferences of customers and channel partners. This additional effort brought insights into their typical preferences, including purchase volumes, pricing sensitivity, and negotiation practices (segmentations and classification models). Armed with these insights, finance proposed a set of guardrails to recommend negotiated discounting of large transactions in alignment with standard pricing used in small and highly frequent transactions. Such guardrails were tested with massive simulations, which provided insights into the potential impact on sales velocity, profitability, and repeatable purchases (recommender models). Once implemented, the model was monitored for efficiency, accelerating turnaround time of transactions and effectiveness, improving overall sales, win rate, and profitability. It was also applied to detect anomalies in requested pricing, terms, and conditions to support internal audit efforts.
Our lesson for implementation was to deploy AI systems gradually, based on achieving the measured goals, and once the humans responsible and accountable for the results understood and trusted them.
Responsible Use of AI in FP&A
One may wonder how organisations can ensure the responsible and transparent use of AI in FP&A processes. Tactically, they should focus on the data privacy of all stakeholders, protect trade secrets and intellectual property, find cost-effective options to adopt AI and strive to clean up their data.
However, everything depends on the firm’s culture at the macro level. AI tools extract data from sources directed by humans such as vendors, consultants or employees. They are also configured and controlled by humans, even when augmenting data sets. For instance, see RAG networks5, which add recent news or relevant details. Top management executives can lead by example and show responsibility and transparency when using AI in FP&A or anything else. These habits should eventually become part of the governance, such as policies and procedures and also a part of the social fabric of the organisation.
Upskilling the FP&A Professionals and Capturing Value
FP&A professionals must work on two fronts:
1. understanding the scope of application along with the potential and limitations of AI techniques;
2. leveraging their current skills and experience to determine where and when these new tools will work well.
The first front requires getting out of the comfort zone and learning the practical essence of the techniques and software applications available to them. They should look for Data Science courses for non-technical professionals or read literature for beginners. To be clear, they do not have to become Data Scientists and learn mathematics or coding, but they do have to sharpen their quantitative intuition to detect whether information, processes or outcomes make sense. In short, they should remain FP&A professionals and expand their expertise to be data literate, jargon-knowledgeable, and immune to IT fads.
The second front is where their humanity comes into play by matching the new AI capabilities with their experience and domain expertise. Let’s remember that AI does not think; it may look like an expert, but it is not. FP&A professionals must always test the assumptions used by any software, challenge the rules, check the data, and verify results. Only after long practice can they build confidence that the AI results can be trusted.
Finance organisations act as stewards of the capital involved in operations and investments. Since Data Science and AI are becoming some of the most powerful tools in CFOs’ toolkits, CFOs are turning into the new economic architects of the firm and the most strategic players in the C-Suite. All this empowers finance professionals to focus on strategic analysis and value-added activities.
“Humans Are Underrated”6
It is evident that AI is still an immature technology in search of practical applications. It will force the creation of new business processes and impose changes to existing ones. Its emerging needs for hardware, software and data are still being sorted out. Overall, it is suffering from extreme hype, mostly driven by its power to “connect the dots” and provide packaged content in a human-like format.
AI has also started to increase existing risks and create new ones: on data privacy, on quality of output (bias and “hallucinations”7), on reputation and compliance, and so on.
AI needs lots of standardisation to be practical, including regulations and controls and validated practices and protocols to source, use, and protect data.
Tesla's Autopilot is a useful reference for the general future of AI. While expectations and hype have been ahead of reality, the change will be gradual even though the technology is improving so quickly, and yet AI is coming.
To watch the webinar where Alex presented his insights, follow this link.
Acronyms:
LR = Linear regressions
SVM = Support Vector Machines
NN = Neural Networks
PCA = Principal Component Analysis
ML = Machine Learning
LLM = Large Language Models
References:
- Friedland, A. (2024, March 11). What are generative AI, large language models, and foundation models? Center for Security and Emerging Technology. https://cset.georgetown.edu/article/what-are-generative-ai-large-language-models-and-foundation-models/
- Wolfram, S. (2023, February 14). What is ChatGPT doing . . . and why does it work? Stephen Wolfram Writings. https://writings.stephenwolfram.com/2023/02/what-is-chatgpt-doing-and-why-does-it-work/
- Anthropic. (n.d.). Mapping the mind of a large language model. https://www.anthropic.com/news/mapping-mind-language-model
- PricewaterhouseCoopers. (n.d.). Finance Transformation – powered by Generative AI. PwC. https://www.pwc.com/us/en/library/webcasts/replay/finance-transformation-and-gen-ai.html
- Amazon Web Services, Inc. (n.d.). What is RAG? - Retrieval-Augmented Generation Explained. https://aws.amazon.com/what-is/retrieval-augmented-generation/
- Colvin, G. (2015). Humans are underrated: What high achievers know that brilliant machines never will. https://www.amazon.com/Humans-Are-Underrated-Achievers-Brilliant-ebook/dp/B00OZ0TLBK
- MIT Sloan Teaching & Learning Technologies. (2024, May 7). When AI Gets It Wrong: Addressing AI Hallucinations and Bias - MIT Sloan Teaching & Learning Technologies. https://mitsloanedtech.mit.edu/ai/basics/addressing-ai-hallucinations-and-bias/