To bring some perspective to this position of AI within the context of FP&A activities, the...
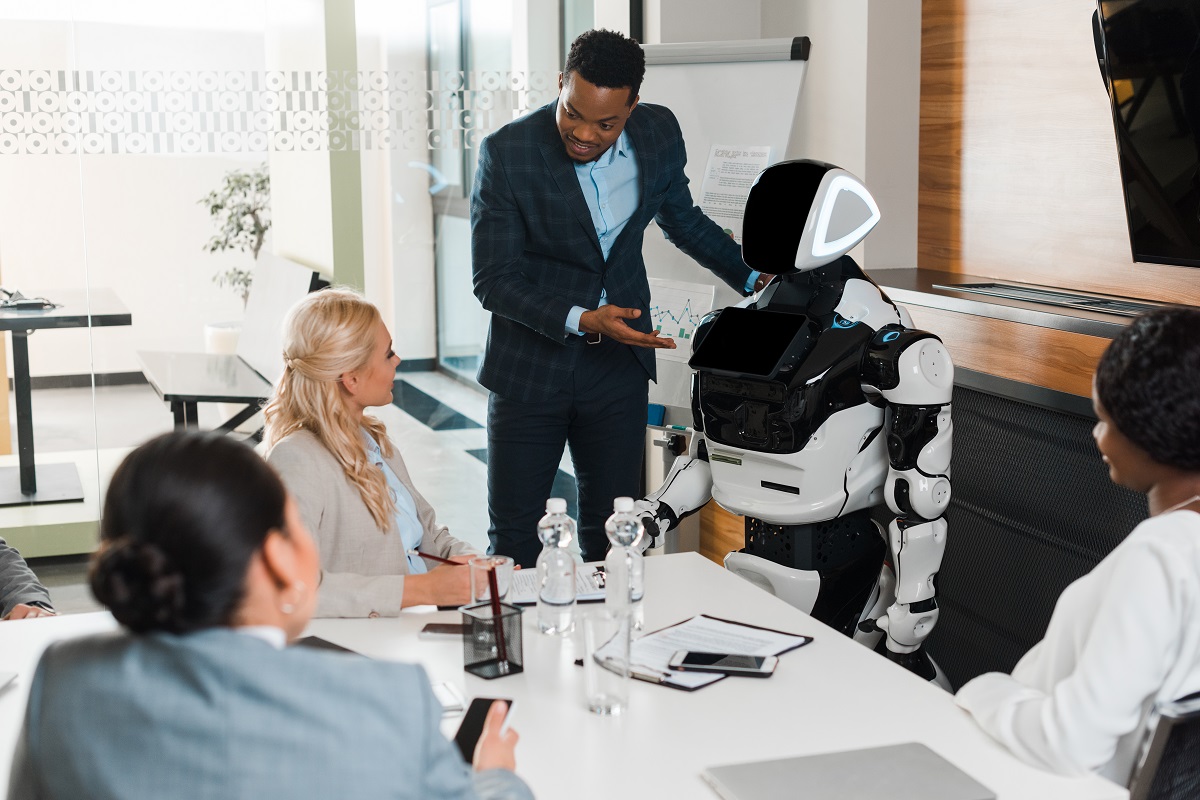
2023 has emerged as a landmark year with the advent of transformative generative Artificial Intelligence (AI) technology. Currently, generative AI applications are predominantly centred around content creation. Looking ahead, the next phase of AI development will venture into more complex domains, including data synthesis, reasoning, and decision-making.
The domain of finance and accounting is not exempt from this transformation. Financial Planning and Analysis (FP&A) teams, renowned for their collaborative and communicative work ethos, are now at the forefront of embracing this technological shift.
The Conventional Landscape of Financial Planning & Analysis
Traditionally, FP&A teams function as an organisation’s nexus of financial forecasting and Strategic Planning. Their methodology starts with gathering data, insights, and forecasts from various business units and then synthesising these into a comprehensive, company-wide perspective. This process is inherently collaborative, involving extensive communication with Business Partners. The resulting analysis forms the bedrock of financial guidance for internal leadership and external stakeholders.
AI Transformation in FP&A
With the advent of AI, traditional processes are poised to undergo significant changes and will bring remarkable efficiencies. Here are the key shifts that we may see in FP&A soon:
- AI automates data collection and integration, enhancing data accuracy and reducing errors, leading to more reliable financial forecasts.
- Advanced algorithms in AI facilitate more accurate financial forecasting.
- Generative AI enables robust Scenario Analysis and realistic financial simulations.
- Real-time reporting and analysis capabilities of AI foster dynamic decision-making.
- AI in routine tasks frees up resources for strategic and analytical activities.
The Evolution of Collaborative Dynamics in FP&A
As we delve deeper into this transformation, we should consider the varying degrees of AI integration and the resultant impact on FP&A operations. These range from AI enhancing human-to-human interactions to AI autonomously interacting with other AI systems. Each scenario presents unique opportunities and challenges. They could occur sequentially or concurrently as we move forward with the AI adoption.
AI as a Facilitator in Human-to-Human Interaction
In this scenario, AI serves as an advanced analytical tool for FP&A professionals, enhancing their ability to provide insights to human Business Partners. AI can process vast amounts of data more efficiently than humans, identifying trends and generating predictions. This will allow FP&A teams to focus more on strategic analysis and less on data processing, improving the quality of the insights provided to Business Partners.
Direct AI-to-Human Interaction
FP&A teams may directly interface with AI systems representing their Business Partners. In this model, AI systems could communicate findings and recommendations, potentially streamlining the decision-making process. However, this raises questions about the nuances of business negotiations and decisions, areas where human judgment and experience are currently invaluable.
AI-to-AI Communication
The most futuristic scenario involves AI systems of corporate entities interacting directly with each other, with minimal human intervention. In such a setup, FP&A teams may evolve into roles focused primarily on setting parameters for AI interactions and interpreting the insights generated. This could lead to highly efficient, data-driven decision-making processes but may also require a reevaluation of the role of human judgment and oversight in financial planning.
Anticipating and Addressing Emerging Challenges in FP&A
Anticipating and addressing the challenges of integrating AI in FP&A is crucial for harnessing its full potential while mitigating risks. These challenges span various dimensions, ranging from technical integration to ethical considerations.
Loss of Nuance
One of the primary concerns is AI's potential inability to fully replicate the nuanced understanding that seasoned FP&A professionals bring. In the realm of finance, human judgment is crucially dependent on the interpretation of subtle cues, an understanding of cultural and organisational contexts, and the ability to make informed decisions even when faced with incomplete information that is not formally documented. In contrast, AI is predominantly reliant on its training data. This data often lacks the nuanced, practical knowledge that primarily resides within the experiential understanding of its users. This distinction highlights a significant gap between human cognitive abilities and the current capabilities of AI in financial decision-making processes. To address this lack of context, AI systems need to be complemented with human oversight. This involves ensuring that FP&A professionals are not just data interpreters but also serve as contextual translators for AI-generated insights.
Integration and Compatibility
Effective communication and integration of AI systems across different departments and functions present a significant technical challenge. Financial data is often interconnected with various business functions like operations, sales, and marketing. For AI to be truly effective in FP&A, it must be seamlessly integrated and communicated with diverse systems, each possibly with its own data formats and protocols. Overcoming these integration challenges requires a robust IT infrastructure, standardised data formats, and interoperable AI algorithms. Establishing cross-functional teams that work collaboratively to ensure seamless integration of systems and data can be a strategic approach to address these challenges.
Ethical and Governance Issues
The shift towards AI-driven FP&A raises critical questions regarding data governance, privacy, and ethical considerations. There's a risk of AI algorithms inadvertently introducing biases or making decisions that lack transparency. To mitigate these risks, it's essential to establish clear governance frameworks that define the scope, limitations, and accountability mechanisms for AI systems in FP&A. Regular audits and reviews of AI decisions, coupled with transparent reporting mechanisms, can help maintain ethical standards and compliance.
Conclusion
The ideal scenario in the foreseeable future is a hybrid model where AI systems handle the heavy lifting of data processing and analysis while humans interpret, guide, and make final strategic decisions based on AI-provided insights. This blend leverages the strengths of both AI and human intelligence, ensuring that FP&A remains dynamic, insightful, and strategically sound in the era of digital transformation.