A strong data strategy is crucial for FP&A teams to ensure accurate, timely and meaningful insights...
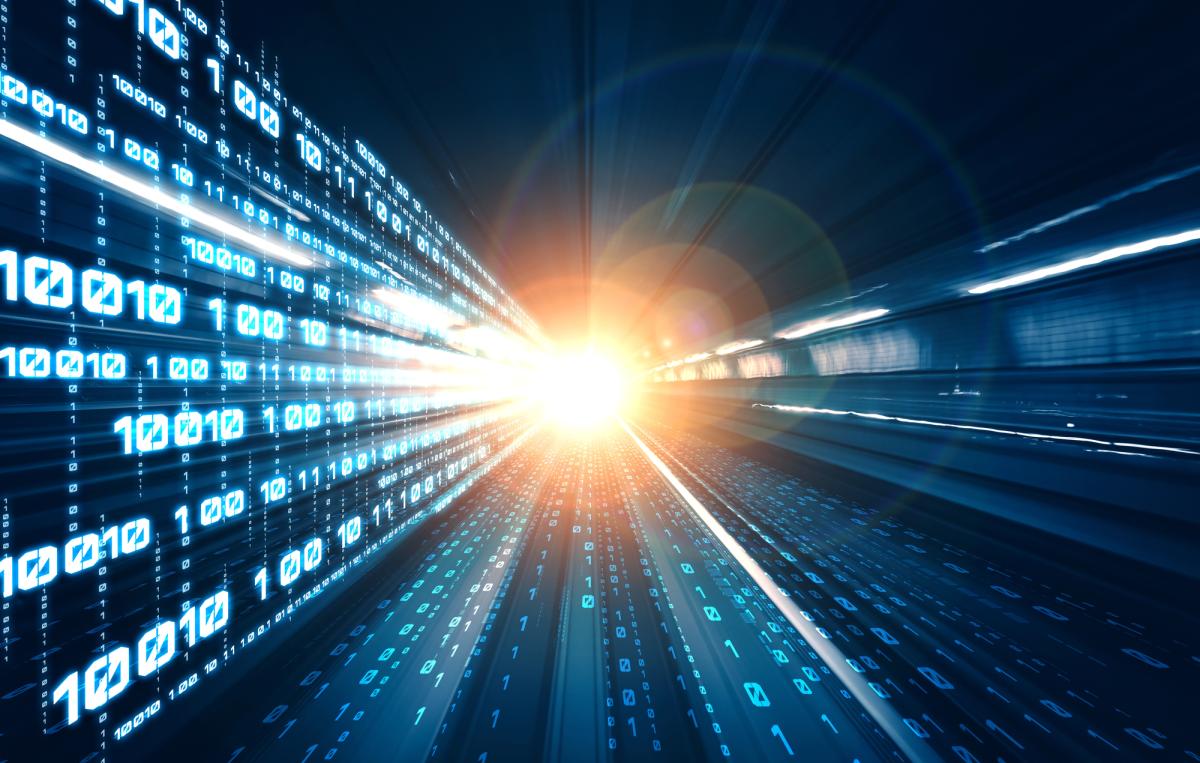
We’ve all been there: staring at financial statements, knowing the numbers tell part of the story, but feeling like a crucial chapter is missing. The income statement is in front of you, and the P&L is clear, but the meaning... the drivers... the future implications... remain elusive. In FP&A, we often feel like financial data alone is a lagging indicator, telling us what happened, but not always why, and certainly not what is likely to happen next.
And what is our typical reaction? We pick up the phone, launching into a ‘telephone game’ of calls and emails, chasing down the backstory behind the numbers. Even then, the narrative pieced together is often incomplete, anecdotal and, frankly, too late. This frantic, reactive approach highlights the fundamental problem: we are trying to understand a multidimensional business with a one-dimensional dataset — purely financial data.
However, the true power of FP&A is unleashed when we move beyond these lagging indicators and actively integrate the missing chapters — the non-financial data that truly drives business performance. Think customer-segment behaviour, market trends, operational efficiency, SKU-level performance, product engagement… By systematically merging these diverse datasets with our core financials, we don’t just get a ‘fuller picture’ — we gain a dynamic, multi-faceted narrative. We move from simply reporting what happened to understanding why it happened and, crucially, what is likely to happen next. It is not just about better, backwards-looking analysis; it is about dramatically enhancing our ability to plan the future strategically, with greater accuracy and confidence.
According to the latest FP&A Trends Survey, 64% of organisations report that the majority of their decisions are data-driven (a double-digit increase from the prior year’s survey), underscoring the growing importance of robust data integration.
So, how do we move from recognising this need to making it a reality in FP&A? The answer lies in embracing the right tools, statistical models, a data-driven philosophy and a practical approach.
In the following sections, we’ll unpack each of these critical elements, providing a roadmap for FP&A professionals to effectively merge financial and non-financial data and unlock a new era of insights.
Laying the Philosophical Foundation: The Data-Driven FP&A Mindset
If the above problem statement resonates, you are on the right track. Adopting a data-driven mindset in FP&A transcends simply using data; it is about embedding data into decision-making. It means cultivating a healthy curiosity, questioning assumptions and seeking empirical validation for strategic initiatives. Practitioners must become proficient in data storytelling, translating complex analyses into clear, concise narratives that resonate with diverse stakeholders. This involves developing a keen understanding of data quality and governance, ensuring the reliability and integrity of the information used. Moreover, a data-driven FP&A professional embraces experimentation, utilising A/B testing and scenario modelling to evaluate potential outcomes and refine strategies. They are comfortable with ambiguity, recognising that data often reveals trends and probabilities rather than absolute certainties (especially important when synthesising financial and non-financial data). This mindset fosters a culture of continuous improvement, where feedback loops are established to refine models and enhance predictive accuracy iteratively. Ultimately, a data-driven FP&A practitioner acts as a strategic advisor, leveraging data to inform and influence critical business decisions, driving sustainable growth and value creation.
How to Identify the Right Non-Financial Drivers
Building upon the data-driven mindset, we move from theory to action, starting with the fundamental principle of strategic data selection. There is a strong temptation, one I wrestle with, to simply ingest every available non-financial data point into our EPM platforms and see what “sticks”. However, this approach disregards basic data governance. As Tanbir Jusimuddin emphasised in a prior FP&A Trends Insights Paper, effective data management begins with data curation. Tanbir outlines a crucial sequence: an information strategy to pinpoint key business drivers, followed by a data strategy to capture, cleanse and organise relevant data, culminating in data governance to ensure ongoing data quality. While a deep dive into data/information strategy is beyond this article’s scope, it is vital to underscore the importance of a systematic approach to data selection and maintenance.
The challenge for FP&A is not just collecting non-financial data; it is selecting the correct data to answer specific business questions. What are the key drivers of customer churn? What operational metrics best predict changes in profitability? What market trends influence sales growth? By focusing on these core questions, we can avoid data overload and prioritise the non-financial variables that provide the most meaningful context to our financial analysis. Statistical models, even for those who are not statisticians, can be immensely valuable in guiding our decisions on which data to integrate into BI or EPM platforms and how to structure that data. For example, techniques like covariance and regression can help us objectively assess the strength and direction of relationships between financial and non-financial variables. And as AI capabilities expand, these analyses are becoming more accessible, allowing us to validate our assumptions and uncover previously hidden but critical correlations. Ultimately, the goal is to move beyond simply having more data to having more relevant data that tells a more complete and actionable story.
Tools: Start Small, Scale Smart
A common misconception is that effective data integration requires a complete overhaul of existing systems and a significant investment in cutting-edge technology. However, FP&A teams can begin their journey with the tools they already have. Spreadsheets, for instance, offer surprisingly robust capabilities for data connectivity and analysis through features like Power Query (Excel) and data connectors (Google Sheets). These can be used to experiment with combining financial and non-financial data, validating initial hypotheses and demonstrating the value of integrated insights. As FP&A teams become more comfortable and confident, they can strategically layer in more specialised tools, such as data visualisation platforms or cloud-based EPM solutions, to automate processes, enhance collaboration and handle larger datasets. The emphasis should be on starting small, iterating frequently and building a strong foundation on which it is possible to scale when ready.
Building on the previous point, there are some important capabilities to consider when it comes to levelling up your toolkit — functionalities that can enable even more robust insights as your integrated data strategy scales and matures. Tools that empower successful data integration in FP&A share several key characteristics. First, they provide seamless data connectivity, enabling FP&A to pull information from diverse sources, including CRM systems, marketing platforms, operational databases and market data feeds. According to the FP&A Trends Survey, 47% indicated they had multiple data sources that required time and effort to merge, reinforcing the importance of reliable data connectivity. Second, they offer data transformation capabilities, allowing for cleaning, standardising and preparing data for analysis. Third, robust data visualisation is essential for exploring relationships and communicating insights effectively. Finally, while not always necessary at the outset, tools that support statistical modelling and analysis become crucial for validating hypotheses and quantifying the impact of non-financial factors on financial outcomes. Whether leveraging a spreadsheet’s built-in analytical functions or employing a dedicated statistical software package, FP&A professionals need tools that facilitate a comprehensive and rigorous approach to integrated data analysis.
Getting Started: Practical First Steps Toward Data Integration
In my experience, it’s easy to get overwhelmed by the prospect of establishing an integrated data strategy, especially when there is a seemingly endless supply of data. But the good news is that it is a marathon, not a sprint and starting small is key. One way we do this is to leverage already-existing processes to down-select the most salient non-financial data. For example, we perform an annual business planning process (i.e. budget), in which our various business lines submit the underlying assumptions behind their budgets. A natural output of this exercise is a robust list of key, non-financial segmented metrics and stats (e.g. revenue drivers). For us, this represents a good starting point in our efforts to catalogue what the operation considers the most essential and financially impactful data points. Additionally, as this non-financial data directly informs the business’s financial projections, we can safely assume we will be able to develop a clear mapping between the two (e.g. North American sales <-> North American subscription volume).
If a naturally occurring process doesn’t afford this information transfer, the next step would be to discuss this topic directly with the folks outside of finance (something that should really happen regardless). Understanding what the front-line operations and sales leaders consider most germane in the context of non-financial data will then enable an efficient prioritisation and action plan for integrating that data with your financial data. And as a by-product, ensure alignment with non-financial verticals. Ultimately, the insights produced from executing on this can then be infused across the organisation, pointing decision-makers in the right direction and ensuring ‘external financialisation’, where everyone across the enterprise understands the financial implications of operational decisions. That should always be a principal aim of FP&A. To help bring this point to life, I’ll share an example. When working at a previous company in the healthcare space, we recognised that our methodology for prioritising different types of procedures was mostly arbitrary. We knew there was wide variability in the revenue of each type of procedure, but didn’t understand the true unit economics of one case vs another. By integrating operational metrics into our data set (procedure timing, materials used, wage rates, etc.), we were able to establish a unit economics profile for each type of procedure, updated in real-time, to prioritise then and operationalise the optimal mix of cases. Further, this capability was shared with the front-line decision makers (in this case, surgeons at a surgery centre), so that they had the agency to make financially optimal decisions for the company. It translated into improved earnings and better alignment between FP&A and the operation.
Conclusion
In conclusion, the journey towards integrating financial and non-financial data is transformative for FP&A. By embracing a data-driven mindset, strategically selecting relevant data and leveraging the right tools, practitioners can move beyond reactive reporting to proactive strategic advising. This evolution enables organisations to gain a deeper understanding of their business drivers, improve forecasting accuracy and, ultimately, drive sustainable growth. Integrating these data sets and the culture of data literacy ensures that every department understands the financial impact of its decisions and that the entire company operates with a single source of truth. As FP&A continues to evolve, this holistic approach to data will become an indispensable asset for navigating the complexities of modern business and unlocking unprecedented levels of value creation.