This article summarises the presentations and discussions during the recent Digital FP&A Circle on how Artificial...
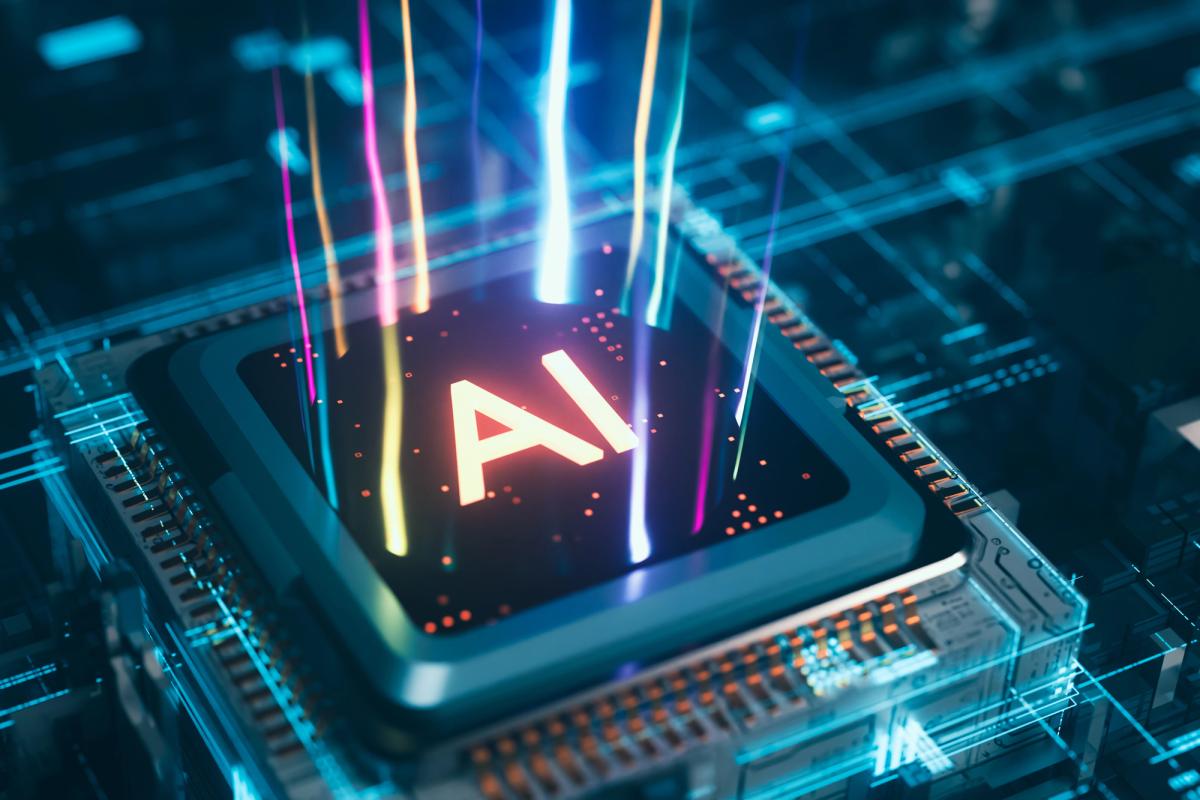
Introduction
Generative Artificial Intelligence (GenAI) is transforming how organisations work by providing easy access to information, improving process efficiency, and enhancing effectiveness across functions like Finance, Operations, Sales, and Marketing. While there was initial hype about GenAI’s feasibility and projects, it has become a reality with organisations adopting it to boost productivity. There is a misconception that GenAI will eliminate jobs, but the future favours those skilled in using GenAI effectively.
This article focuses on GenAI in Finance, detailing various Enterprise GenAI Solutions, use cases within the Finance domain, and an implementation approach through a practical case study of Juniper Networks. It highlights steps taken and lessons learned during their GenAI project journey.
About Juniper
Juniper Networks (JNPR) is a networking industry company with $5.07 billion in annual revenue (FY’24) spanning enterprise, service provider, and cloud segments. Juniper Global Business Service (Juniper GBS) was established in 2005 as a Shared Service Centre. Over 20 years, it has been transformed into a Centre of Excellence across multiple areas. At the time of writing, Juniper GBS is operating predominantly from locations in India and the USA, providing services in accounting and reporting, Financial Planning and Analysis (FP&A), operational analytics, Quote to Cash (Q2C), order management, master data management, service delivery, Accounts Payable (AP) and so on. Hackett Group has rated Juniper GBS as a Digital World Class Organisation for six consecutive years.
GenAI Foundation
A GenAI application relies on a Large Language Model (LLM) that must be regularly trained. This model understands user queries, makes inferences, generates relevant content, and offers recommendations.
Enterprise-Level GenAI Applications & Use Cases
There are three types of enterprise-level GenAI applications:
Embedded GenAI Applications: Include third-party tools where GenAI functionality is integrated within the application. They require minimal customisation and are plug-and-play solutions.
Domain Specialised: These solutions require domain expertise in the insurance, banking, and finance sectors. Examples include internal audit and compliance activities.
Native GenAI: These are enterprise versions of foundational models that function as digital or virtual assistants, aimed at increasing productivity, effectiveness, and decision-making capabilities. Native GenAI Solutions require extensive customisation, with the best approach being the development of a private LLM with appropriate security and governance measures.
GenAI Solution Adoption
GenAI Solutions across Finance and Operations involve three broad areas of work optimisation.
Structured: This type of work follows clearly defined business rules, applied to structured inputs with slight variation, to deliver predictable and repeatable outcomes.
Unstructured: Work involving application or acquisition of knowledge, including cognition, decision-making, creation, contextualising, conceptualisation, and risk assessment.
Interactive: Work involving interpersonal communication within business functions, function staff, internal customers, external customers, external service providers, and regulators.
Let us delve into the Juniper Networks Finance case study, where the Juniper Finance team implemented enterprise-wide GenAI projects.
Case study 1: Virtual Assistant
Background:
The Juniper Global Sales Compensation (GSC) team, part of the Juniper Finance and Operations team, handles queries from Sales team members (20% in volume) related to non-sensitive, policy-related topics such as system access, leave of absence policies, and special incentive programs. Previously, Sales team members sent their queries via email to the GSC team, and responses were provided manually based on time zone and perceived priority. There was no mechanism to track priority and respond accordingly during periods of high volume.
Project Details:
The project involved leveraging GenAI to develop a Sales Compensation Virtual Assistant (Digital Assistant) that would respond to non-sensitive, policy-related queries (workflow-driven, rule-based engine). In cases where the response was unsatisfactory, a virtual agent would automatically create a ticket on behalf of the Sales team member, assigning it a priority level to ensure timely follow-up by the GSC team.
Solution Design:
The digital assistant was developed on an enterprise knowledge base platform. It allows users to ask questions and type in a prompt. The assistant returns a policy-aligned answer in seconds.
In the background, all relevant policy content, covering topics like system access, leave of absence, incentive programs, and termination, had to be carefully uploaded and refined to fit the platform’s predefined Knowledge Base (KB) format. It was done iteratively, improving the assistant’s responses with each pass.
Virtual Assistant responses were evaluated on Completeness, Accuracy, and Reliability.
A repository of historical queries was created with correct output provided by the GSC team, and it was compared with the assistant-generated virtual production. In case of discrepancies, the model (KB article) was trained to generate accurate responses by assigning probability and refining it further.
Workflows were created for hot topics (top 4 most searched items related to system access, leave of absence, incentive mechanism, and others) to augment the quality of virtual assistant responses. (Retrieval Augmentation Generation)
Database: All the queries with data logs, responses, and prompts, workflow were stored within a repository (MySQL database)
Benefits:
Drives Standardisation via automated response that adheres to policy.
Enables Self-Service (rather than depending upon the GSC team), including automation of help desk creation, leading to faster response times. Leveraging the virtual assistant has led to a 20% reduction in GSC team efforts (processing time).
Improves Customer Experience by leveraging the latest GenAI features and driving operational efficiency.
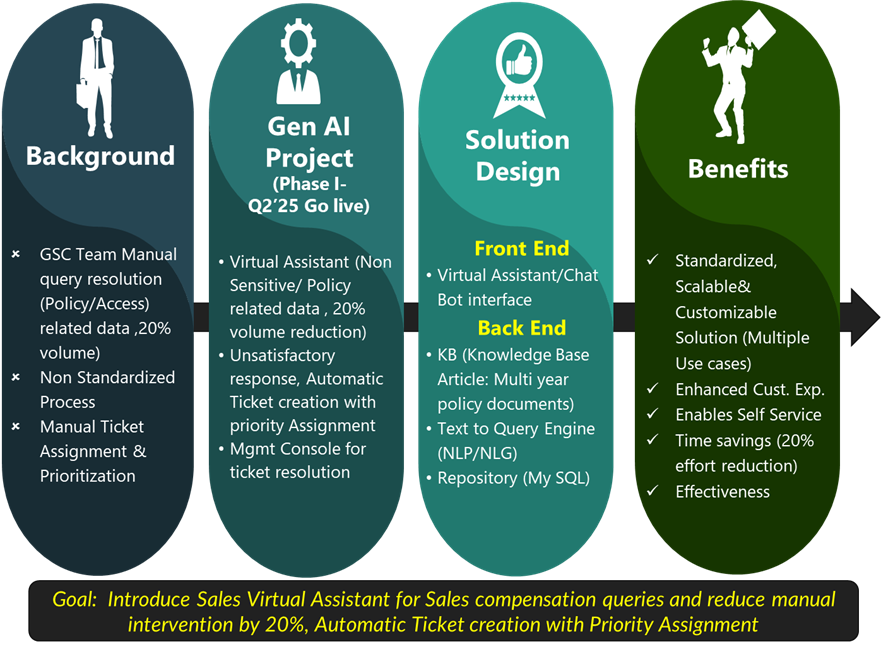
Figure 1: Juniper Sales Comp Virtual Assistant: Native GenAI Application
Case Study 2: An AI-Powered Expense Validation Solution
Background:
Lack of a mechanism to detect risk and potential Policy violations in Expense Reimbursement. Whenever users submitted expense receipts, they were reviewed manually for compliance, an inconsistent, labour-intensive process that wasted time and resources.
Project Details:
Leverage AI to detect non-compliance, fraud, and manual errors by employees. The solution also identifies external risks such as Politically Exposed Persons and Merchant Category mismatches. Enable auto-processing of expense reports for valid claims.
Solution Design:
An AI-powered rules engine was integrated into the company’s expense processing workflow to validate reports in accordance with internal Travel and Expense (T&E) policies. The system identifies potential policy exceptions and automatically approves the expense report based on predefined thresholds for valid claims.
Benefits:
Policy Adherence: Helps to comply with Government regulations and Juniper Policy.
Actionable Insights: Utilise AI insights that are beyond human review insights.
Drive Policy Changes: Any policy change can easily transition into a new policy without any hassles.
Reduction of two FTEs through productivity gains.
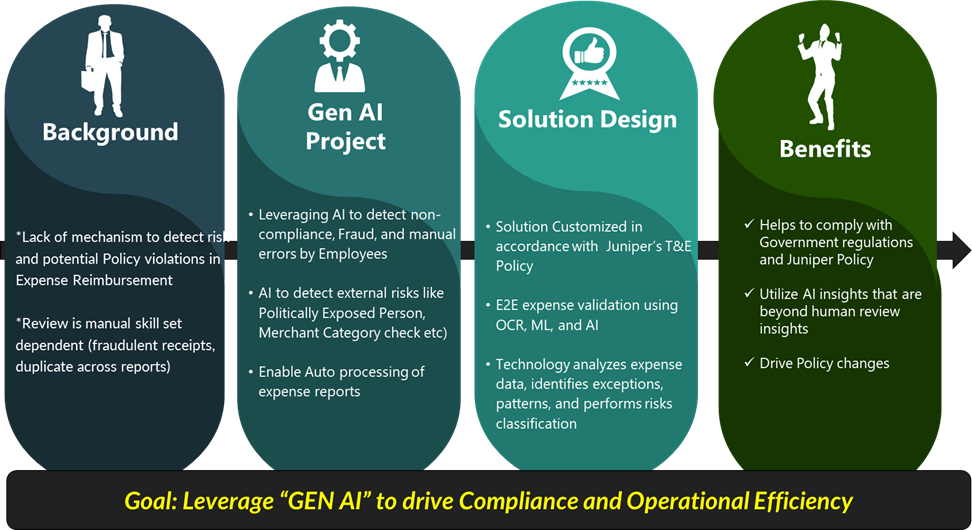
Figure 2: Juniper T&E GenAI: an AI-Powered Expense Validation Solution
Juniper Finance GenAI Projects: Key Learnings
Not ‘Plug n Play’ Solution: Generative AI Solutions require a structured approach like traditional enterprise projects, involving multiple milestones and a significant learning curve that typically spans 4-5 quarters before achieving reliable outcomes.
Probabilistic vs. Deterministic: GenAI Solutions are inherently probabilistic, with low initial accuracy. For example, Juniper’s initial response accuracy for specific virtual agents was around 40%, which improved to approximately 90% after refining models and collaborating with vendors.
Preparation and Background Work: A thorough preparation phase is essential, during which teams must build a repository of accurate outputs to compare against GenAI-generated results. It enables the assignment of probabilities and guides further model improvement.
Juniper Finance GenAI Projects: Critical Success Factors
Prompt Engineering: The initial queries (prompts) provided to GenAI Solutions should be assessed via three key parameters: the Completeness, Accuracy, and Reliability of their responses. It is an iterative process in which probabilities are assigned to validated outputs, helping to identify prompts that succeed on the first attempt and those that require refinement or additional training of the GenAI model.
It is essential to comprehensively test all prompts, utilising fuzzy logic, near word search, and exact search methods.
Cross-Functional Team: To ensure project success, the GenAI Project team should consist of a balanced representation of domain expertise, IT, and project management.
Change Management: GenAI projects entail significant change management, including process refinement and adoption of modern technologies. A robust management strategy, communication plan (including periodic roadshows, training sessions, and detailed user guides), and risk assessment are crucial to project success.
Executive Support: GenAI projects involve substantial costs and resource investments, making top management support for funding, timelines, and alignment critical for success.
Governance: A governance framework for data reliability and accuracy is essential to address the risk of AI hallucinations. At Juniper, the Governance Council and committee validate use cases and provide approval at the POC and Go-Live stages.
Next Steps for Practitioners Trying to Implement GenAI Projects in Finance
Assessment: Identify the nature of work that needs to be automated via GenAI, whether it involves Structured, Unstructured, or Interactive work. Depending upon the use case, one can explore various enterprise-wide GenAI Solutions viz. 3rd Party inbuilt applications, domain-based, or Native GenAI Solutions.
Structured Approach: GenAI Solutions need a structured approach with multiple milestones and a 4-5 quarter learning curve to achieve reliable outcomes.
Probabilistic Nature: Initial GenAI accuracy is low but can improve significantly with model refinement and vendor collaboration.
Preparation Phase: Build an accurate output repository to compare against GenAI results, guiding model improvement.
Prompt Engineering: Assess initial queries by Completeness, Accuracy, and Reliability. Iteratively assign probabilities to validated outputs, refining prompts and training the model as needed.
Comprehensive Testing: Test all prompts using fuzzy logic, near word search, and exact search methods.
Cross-Functional Team: Involve domain experts, IT, and project managers for balanced project execution.
Change Management: Implement robust change management, including process refinement, modern technology adoption, and comprehensive communication plans.
Executive Support: Due to substantial costs and resource investments, ensure top management support for funding, timelines, and alignment.
Governance Framework: Establish a governance framework to ensure data reliability and address the risk of AI hallucinations.
Subscribe to
FP&A Trends Digest
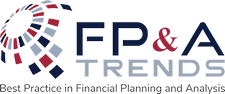
We will regularly update you on the latest trends and developments in FP&A. Take the opportunity to have articles written by finance thought leaders delivered directly to your inbox; watch compelling webinars; connect with like-minded professionals; and become a part of our global community.