In this article, the author shares a few practical strategies for implementing AI and data science...
FP&A Trends Group recently hosted a webinar on the deployment of Artificial Intelligence (AI) and Machine Learning (ML) in financial planning, budgeting, and forecasting. This insightful session brought together over 920 registrants from 65 countries and featured presentations by experts from various industries. They discussed the transformative role of AI/ML in enhancing the accuracy and efficiency of FP&A processes.
Here, we recollect the key takeaways from this webinar.
Agenda of the Webinar
The FP&A Trends Webinar covered key topics to guide FP&A professionals through the process of AI/ML adoption. The agenda included:
- Key Concepts and Definitions: the panel clarified what AI/ML entail and how these technologies can be applied in FP&A.
- Machine Learning for Forecasting and Budgeting: speakers shared practical examples of using AI/ML and showcased how ML can optimise forecasting processes.
- The Importance of Good Data for AI Deployment: one of the panellists highlighted the importance of understanding how data quality impacts AI effectiveness.
- Leveraging Technology through Good EPM with AI/ML Capabilities: we’ve explored how technology platforms like Enterprise Performance Management (EPM) can support AI/ML integration.
- Conclusions and Recommendations: actionable insights for organisations looking to implement AI/ML.
1. Key Stats and Challenges
The webinar facilitator, Hans Gobin, started the session by highlighting some stats from the 2022 FP&A Trends Survey. Among the most notable points were:
- 45% of the FP&A team’s time is spent on low-value activities, such as manual data entry and non-strategic tasks like data validation.
- Only 33% of the time is spent on high-value activities, like Strategic Planning, insight generation and decision-making, which are crucial for business success.
- The desired time distribution should be 55% on high-value tasks and 45% on low-value tasks.
- 42% of respondents can produce a forecast in less than a week, but only 21% can do so in less than two days.
- Only 39% of respondents are satisfied with their forecasts, citing issues with accuracy and timeliness.
However, the overall picture changes when AI/ML are introduced:
Time spent on high-value activities increases by 16% to 49%. On sharing these stats, Hans Gobin gave the floor to webinar panellists.
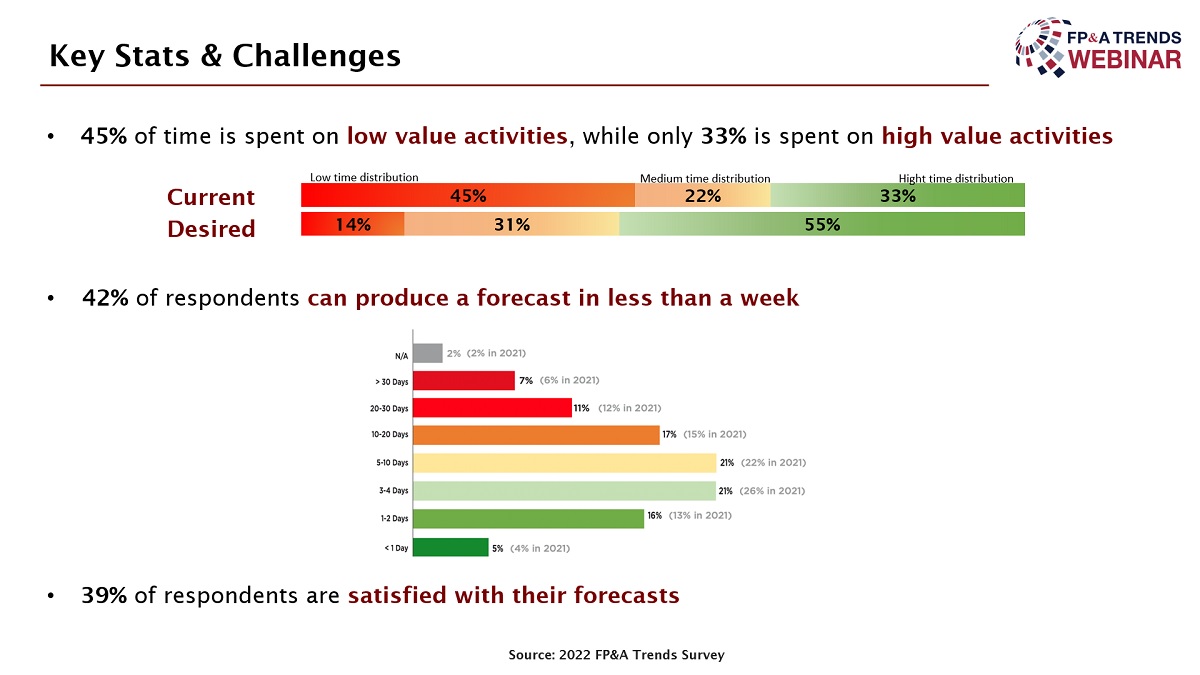
Figure 1
But what if we compare this information with the insights from the 2024 FP&A Trends Survey? Are there any significant changes? Unfortunately, the time distribution on non-value-adding activities has remained unchanged as per the most recent survey. However, we can point out that the use of AI/ML increases time distribution on value-adding activities by 5%, up to 39%.
Machine Learning for Forecasting and Budgeting
During the session, Hashim Ahmed, former CFO at Jaguar Mining, delved into Continuous Planning enhanced with ML. He emphasised how traditional forecasting methods, prone to human bias and errors, can be replaced or augmented by AI to make the process more agile and data-driven. To achieve this, you need to make sure the organisation has a robust cloud-based computing platform and runs financial statement flows at least monthly. In his insightful presentation, he shared the following examples of AI-powered Continuous Planning:
1. Pricing:
- Digitised Input: New purchase orders or contracts signed.
- Plan Impact: Automatic update of price assumptions in the plan.
- Automated Action: The price for the term of the contract is changed without human intervention.
2. Labour:
- Digitised Input: Vacation schedules and planned leaves, along with resignation and rehiring windows.
- Plan Impact: Updates headcount and labour availability projections automatically.
- Automated Action: Gaps in labour availability are directly fed into the plan.
3. Maintenance:
- Digitised Input: Sensors and telematics monitoring tyre wear and utilisation on vehicles.
- Plan Impact: Updates assumptions related to maintenance needs, such as tyre consumption.
- Automated Action: Generates a flag if the two-month moving average consumption exceeds 5% of current assumptions, prompting review.
4. Capex (Capital Expenditure):
- Digitised Input: Delays in capital expenditure due to ordering or logistics issues.
- Plan Impact: Updates capital allocation and timing of spending based on real-time data.
- Automated Action: Generates a flag for review if delays are detected, ensuring plans are adjusted accordingly.
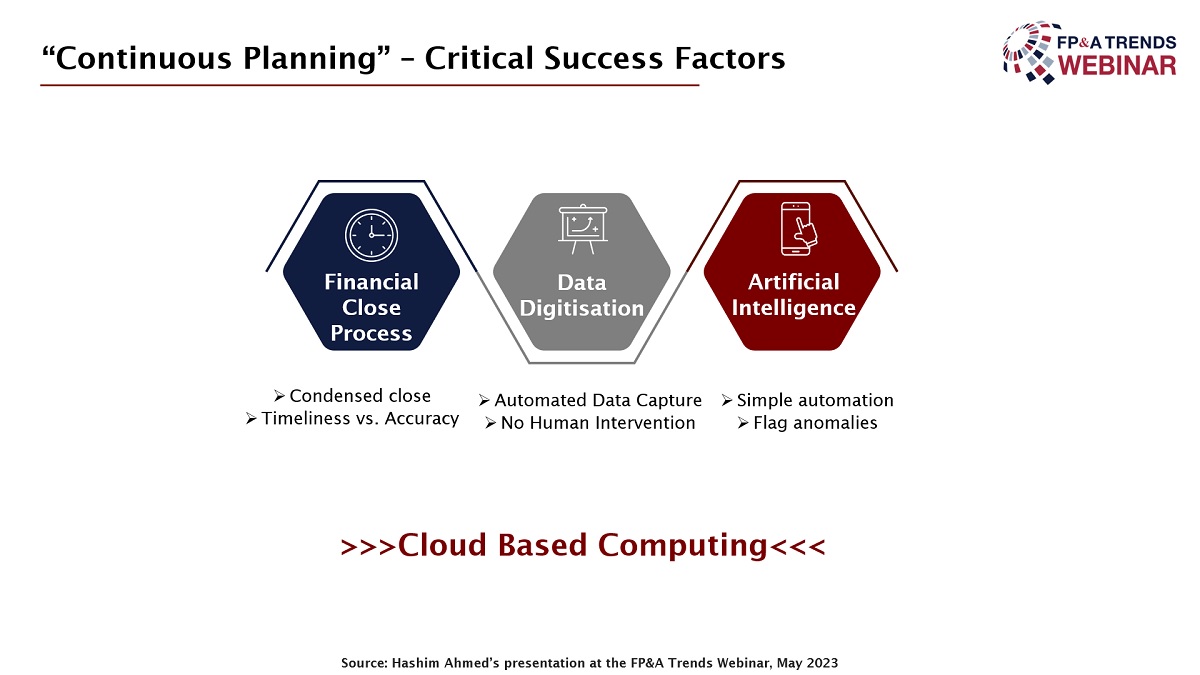
Figure 2
Where Are We? Do Businesses Leverage AI/ML in FP&A?
Right after Hashim’s presentation, the webinar audience was asked the first polling question. Surprisingly, only 1% of the attendees use AI/ML in finance and FP&A, whereas 52% report they do not use AI/ML. 33% plan to do so, and the rest, 13%, state they leverage only some elements of the enormous AI/ML potential. Good news: according to the 2024 FP&A Trends Survey, the percentage of those who actively leverage AI/ML in FP&A rose to 6%.
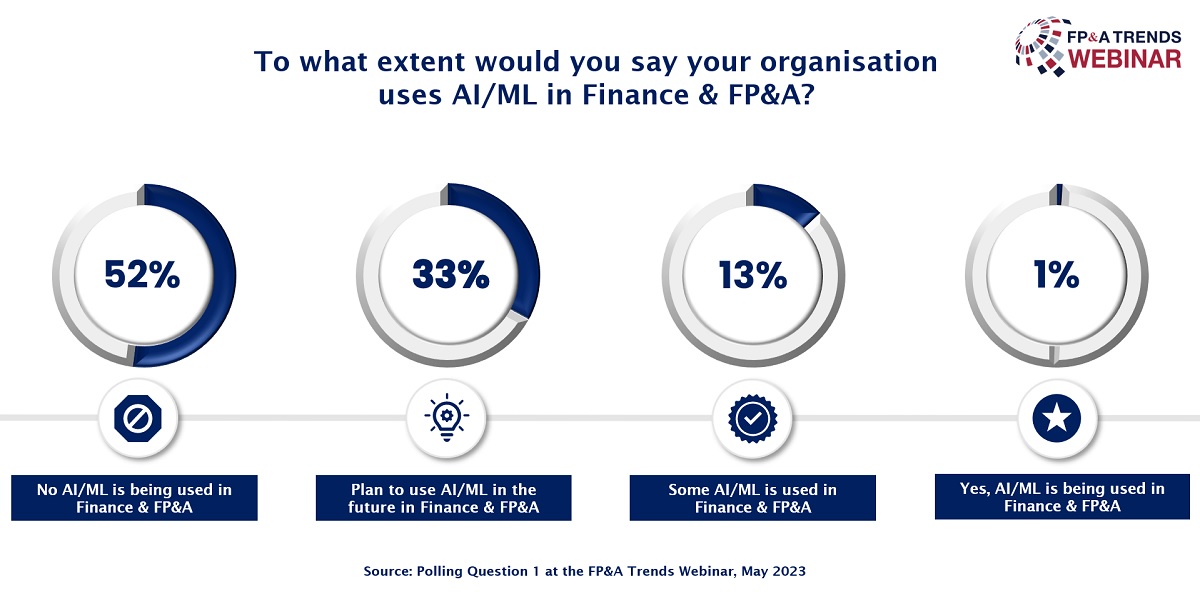
Figure 3
The Importance of Good Data for AI Deployment
Tanja Schlesinger, VP OneSource at Deutsche Bahn Regio AG, underscored the critical role of data quality in AI/ML deployment. According to Schlesinger, the accuracy and completeness of data are paramount for success. In her organisation, AI models are used to reduce energy consumption, optimise fuel consumption by analysing driving behaviours, and improve train operations. They also deploy AI models to achieve better punctuality, which leads to higher customer satisfaction through timely data.
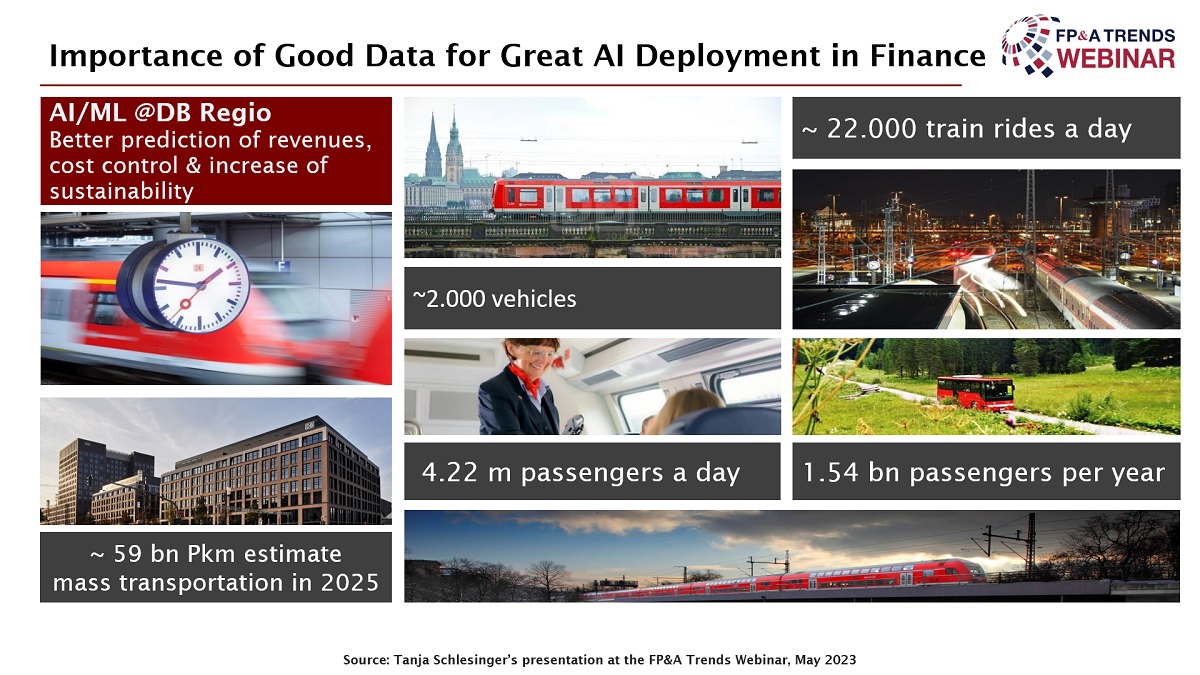
Figure 4
She also stressed that data digitisation, supported by consistent quality checks and ownership, forms the foundation for successful AI implementation. Without these checks in place, even the most advanced AI solutions will struggle to deliver reliable results.
What Efforts Is Data Validation Worth?
Hans Gobin launched the second polling question after Tanja’s presentation. We explored what efforts organisations make to validate their data. Most (64%) claim they implemented manual or semi-automated Excel checks, while 12% say they do not put effort into this process. Introducing KPIs to monitor the data input interface is the right way for 17% of the respondents, and 8% conduct continuous quality assurance throughout the business.
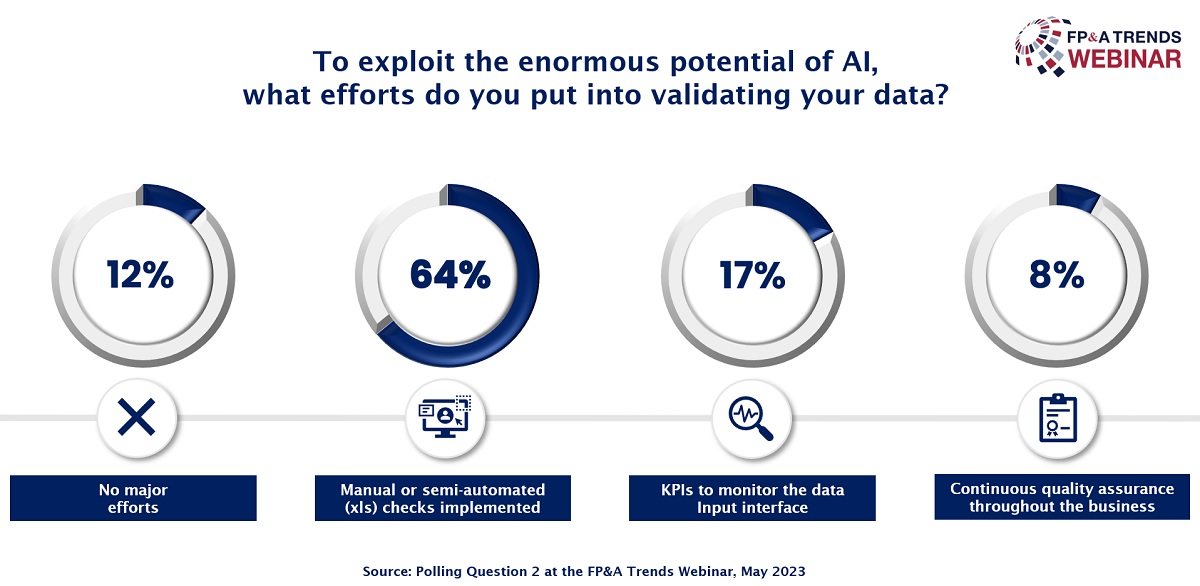
Figure 5
Leveraging Technology through Good EPM with AI/ML
Cody Johnson, Manager, Product Management at Workday, discussed how technology platforms like Enterprise Performance Management (EPM) can be the cornerstone of integrating AI/ML into FP&A practices. By embedding Predictive Analytics, organisations can use anomaly detection, outlier reporting, and Machine Learning-driven forecasting to enhance their planning processes.
Cody demonstrated that leveraging AI can help businesses move from time-consuming manual processes to automated, more intelligent systems. In turn, they will drive better accuracy and allow finance teams to focus on strategic tasks rather than data entry and error correction.
Conclusions and Recommendations
The key takeaway from the webinar was that AI/ML can significantly enhance the accuracy and efficiency of FP&A functions. By reducing the time spent on manual, low-value tasks, finance teams can focus more on strategic analysis and influencing decision-making. The experts recommended starting small with AI implementation, focusing on automating specific processes. Ensuring data quality remains a top priority.
This webinar was sponsored by Workday.