This article explores the roles of Human Intelligence and AI in FP&A, supported by findings from...
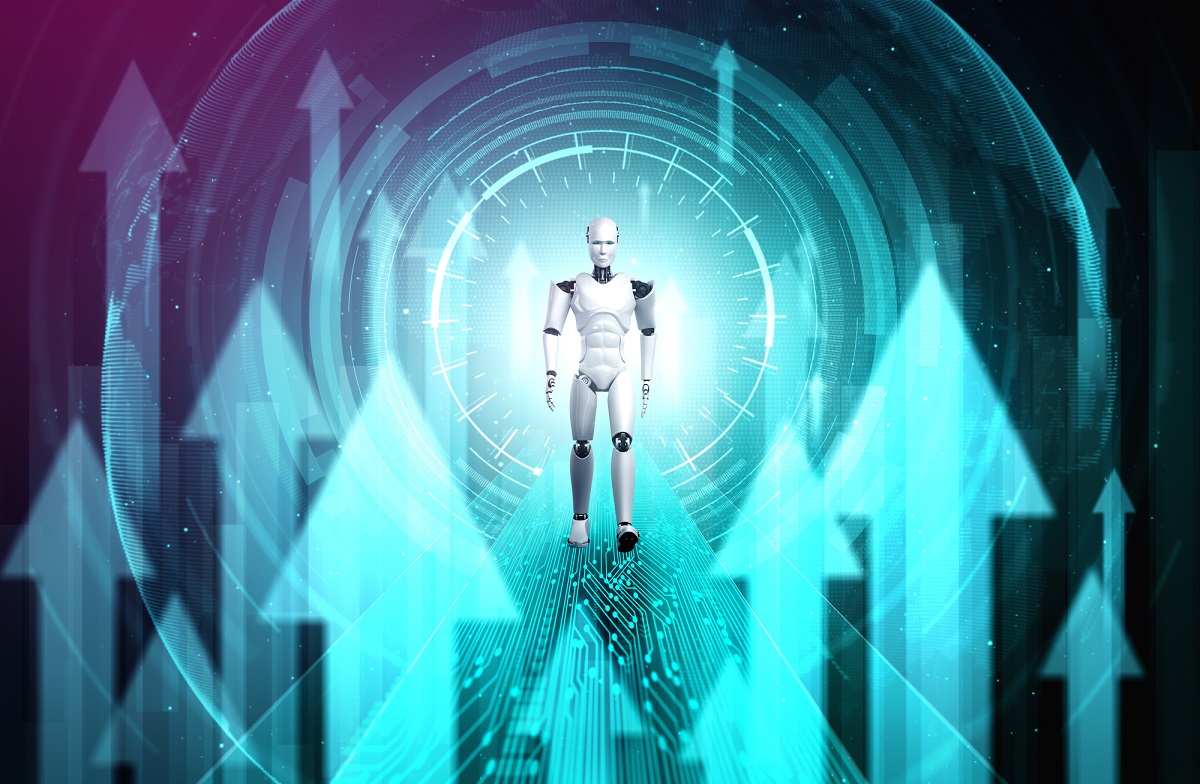
Introduction
Artificial Intelligence (AI) and Machine Learning (ML) integration is nothing but a revolution aimed at redefining financial forecasting, risk management, and, ultimately, how we work with this comprehensive tool for the decision-making process. While the use of AI-enabled techniques promises unparalleled precision and efficiency, there are some associated challenges and potential risks.
Achieving Better Accuracy and Speed with AI/ML
Historically, financial forecasting has always been a complex and uncertain subject. It heavily relied on past data and humanistic perception, which was often erroneous due to bias, leaving alone a limited capability of analysing Big Data. Artificial Intelligence has overcome these limitations, and now, a large amount of data can be evaluated with incredible speed and accuracy. Moreover, Machine Learning predictions have become more accurate, and they improve each time we use them due to the self-learning mechanism implemented in the system.
I can think of an example from my experience when AI greatly enhanced our ability to predict the future. We used an AI-powered model to forecast sales for a wide range of products on a quarterly basis. Due to the sheer volume of data and the unpredictable nature of the market, our previous approaches frequently failed to deliver the desired results. In contrast, the AI-powered model examined past sales figures, current market tendencies, and the tone of social media to produce predictions that were 30% more precise than our earlier projections. We were able to reduce costs and increase customer satisfaction by adjusting our inventory levels accordingly.
However, the potential use cases of AI and ML go far beyond just forecasting. Let us consider risk management, where AI and ML models can provide in-depth know-how for assessing credit risks, detecting fraud and ensuring compliance with policy and regulations. For example, banks are widely using AI language-based models in Know Your Customer (KYC) to evaluate their creditworthiness. These models not only consider the traditional credit scoring factors but also have built-in functions to evaluate each person individually, some of which can be overlooked in a generic approach.
Significant losses that arose in the 2008 financial model due to miscalculation of credit risks might have been avoided if modern AI systems had been in place in past. Modern tools evaluate not only financial data but also non-conventional payment records for utilities rents and so on, allowing for a more comprehensive risk assessment. According to McKinsey & Company, banks employing AI-driven risk management systems saw a 25% reduction in credit losses1.
Regulatory Challenges and Transparency Issues
Despite the growing popularity of AI and ML in the financial sector, we still need to consider some elements. One of the main factors is the absence of detailed regulation and legislation for AI and ML models, reducing the transparency of its operations to its users. This can be problematic in a highly regulated financial environment where data preservation is crucial.
Moreover, while AI systems are highly adaptive for processing past data, they struggle with one-time events. For instance, the COVID-19 pandemic may cause data disruptions or provide data unsuitable for evaluation. It is an inherent limitation of sole reliance on AI and ML for decision-making. In such scenarios or unexpected situations, human intervention is highly significant.
Data derived from AI and ML may be biased if the model has been trained on historical data and assumptions, which can result in unfair outcomes. For example, an AI system used for defining creditworthiness may favour some customers, provided they have been somehow prioritised by conventional models in the past. Moreover, we cannot overlook the potential losses associated with AI-driven decision-making.
Another consideration and one of the main issues of AI deployment is the inherent complexity of these models, which can complicate consumer understanding of how specific forecasting outcomes are produced. This difficulty may cause mistrust among interested parties, which could prevent some firms from integrating AI-powered technologies. In particular, finance professionals need a clear grasp of AI-driven decision-making to properly explain and defend these conclusions to clients and authorities.
Moreover, the implementation of AI systems comes with significant financial investments. Developing, testing, and applying automated financial forecasting models requires a considerable investment in cutting-edge technology and highly skilled personnel. These huge requirements can cause a significant problem for small and medium-sized (SMEs) businesses, making it challenging to delegate the necessary resources. Furthermore, the ongoing requirement to upgrade and maintain these systems to incorporate new data and changing market conditions only increases the operational strain.
While a complete adaptation of AI and ML models may have some inherent risks, a perfect mix of AI and human expertise can bring us far better results. Trained ML models can do Big Data analysis, and humans can judge those findings for decision-making. Let’s say a financial brokerage firm is using an AI-based model to analyse market data to highlight promising stock investing opportunities. At the same time, a financial analyst can use this analysis along with current market sentiments in terms of geopolitical events and recent emerging news to find a winning, profitable opportunity.
Conclusions
In summary, it is irrefutable that AI and ML are transforming financial forecasting, risk management, and decision-making. Although they have substantial risks and challenges, they provide incomparable efficiency and accuracy. A well-adjusted approach that integrates the strengths of AI with the judgment and oversight of human experts is the key to unlocking their maximum potential. It is essential to maintain a vigilant stance regarding the regulatory, ethical, and real-world implications as we continue to navigate this technological frontier, ensuring that the revolution in finance benefits all stakeholders. The future of finance is not solely about the development of more sophisticated algorithms. It is also about the integration of technology and human insight in a more intelligent manner, thereby establishing a more equitable and resilient financial ecosystem.
References:
1. The Future of Risk Management in the Digital Era (Report). December 15, 2017. McKinsey & Company. https://www.mckinsey.com/capabilities/risk-and-resilience/our-insights/the-future-of-risk-management-in-the-digital-era#/
Accessed July 24, 2024.