The 35th in-person London FP&A Board convened on 23 January 2025, bringing together 37 senior finance leaders from organisations such as Vodafone Group, GE Healthcare, Cognizant Technology Solutions, State Street, Financial Times, Bank of England, JLL, IQVIA, Amnesty International and Cleveland Clinic. Held at The Clubhouse, St James the session explored how FP&A is using Artificial Intelligence (AI).
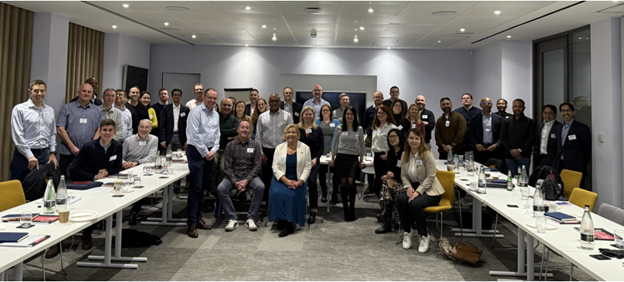
Figure 1. The 35th in-person London FP&A Board, January 2025
Larysa Melnychuk, CEO of FP&A Trends Group and Founder of the International FP&A Board, chaired this meeting sponsored by Workday Adaptive Planning in partnership with Robert Walters and International Workplace Group.
The agenda covered the two branches of AI that are used for decision-making, the vision for AI in FP&A and the human role in this new environment, supported by active discussions about challenges and opportunities experienced by the participants in their organisations.
As an ice-breaker, participants were asked to describe, in one word, how they feel about AI’s impact on FP&A. Most responses reflected optimism: ‘exciting’, ‘efficiency’ and ‘opportunity’ were the most popular answers. Other sentiments included ‘potential’, ‘insightful’, ‘important’, ‘transformation’, ‘intrigue’, ‘open-minded’, and ‘fabulous’. However, some also expressed ‘uncertainty’ and ‘challenge’, highlighting a cautious approach to AI adoption.
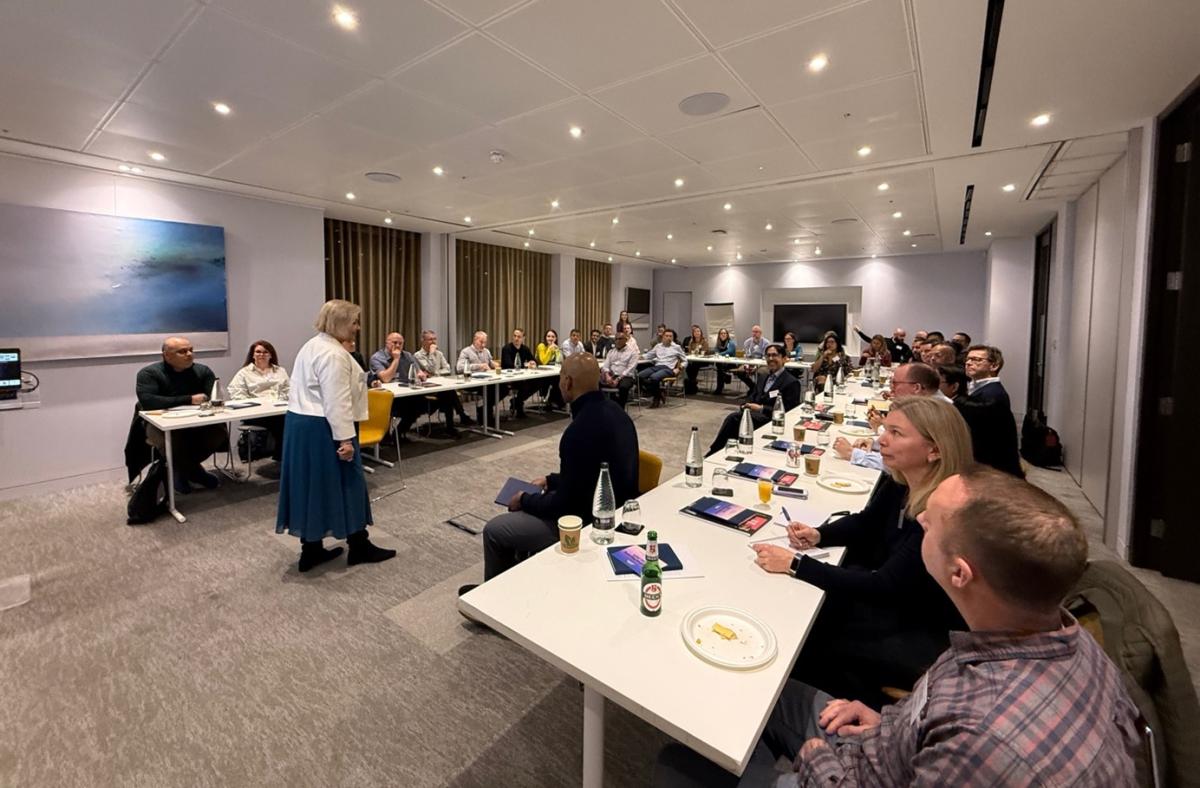
Figure 2. The 35th in-person London FP&A Board, January 2025
Two Branches of AI for FP&A: ML and GenAI
The two main branches of AI are:
- Machine learning (ML) — Analyses numerical data, identifies patterns and makes predictions based on historical trends.
- Generative AI (GenAI) — Processes text, enhances ML with natural language interfaces and generates new content beyond predictive analysis.
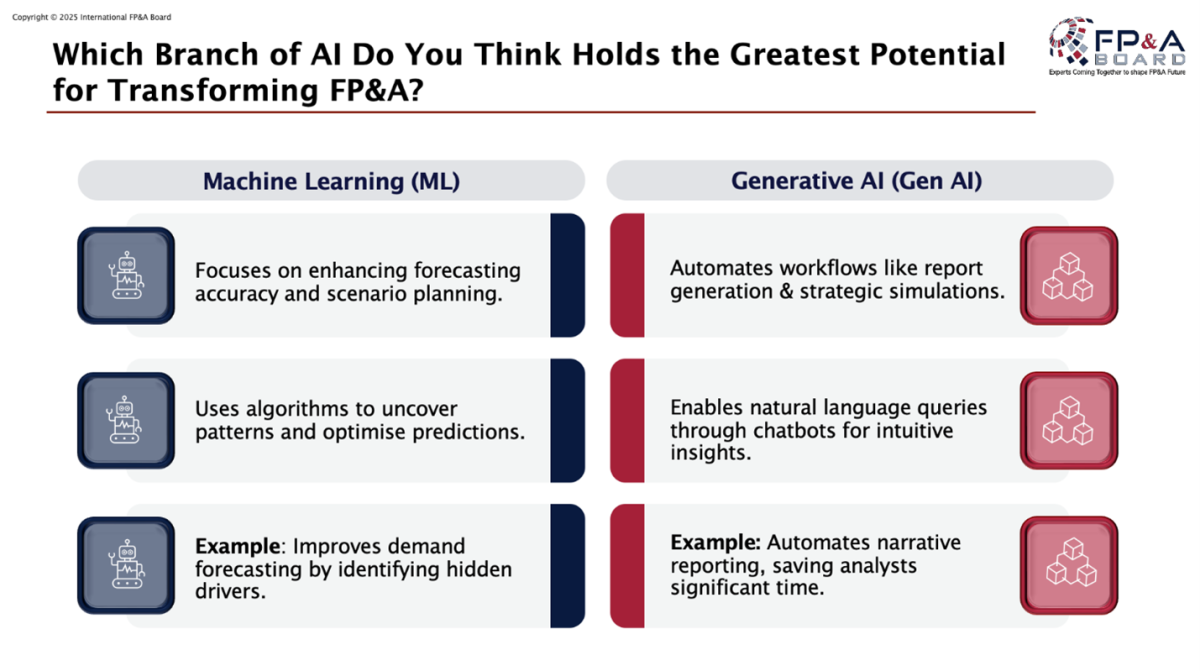
Figure 3
Challenges & Opportunities
Participants shared their AI adoption status:
- 15% use AI for FP&A report summaries and insights
- 12% leverage AI for data pattern analysis and process automation
- 54% are not yet using AI in FP&A
This echoes the 2024 FP&A Trends Survey, where only 6% of organisations reported using Machine Learning, a drop from 11% in 2021, with 35% of organisations still having no plans to adopt AI.
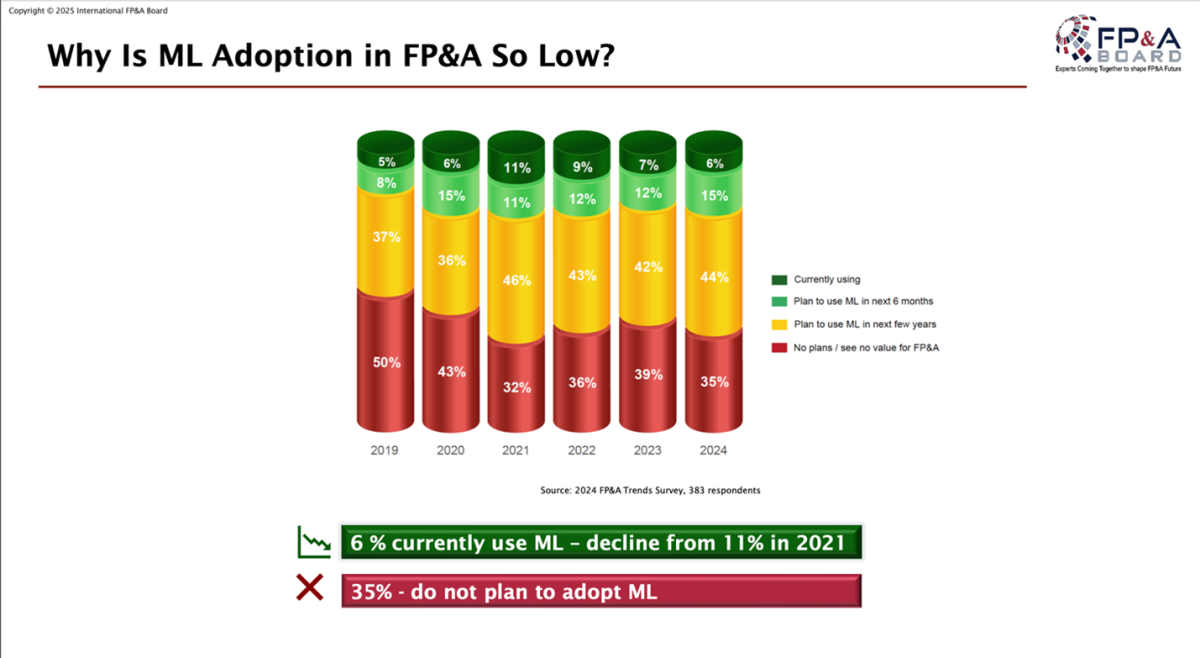
Figure 4
The sentiment in the room was that many organisations lack quality data (33%). Ideally, ML requires 10 years of historical data. Another challenge is the "Black Box" effect, making AI-generated forecasts difficult to interpret.
- Do we understand our business enough to explain the underlying drivers of ML-generated forecasts?
- Do we recognise the data challenges to avoid making incorrect conclusions?
Supporting the AI implementation and getting value out of AI-generated forecasts also requires a cultural change.
One of the new finance roles to address these challenges is FP&A Data Scientist, who is a bridge between IT and finance. While many employers try to upskill their existing teams, many still lack the expertise to work with ML models (38%). There is also a growing need for sufficient knowledge of AI to initiate the transformation, with an understanding of what should be changed in systems, processes and data.
The GenAI is a game-changer as it doesn’t require the knowledge of a programming language, which is likely to lead to a lot more people trying it.
The AI transformation journey typically spans several years and starts with building a high-quality data warehouse. The foundation for AI success in FP&A is data readiness — a good history of reliable data. The next step is the implementation of integrated tools — FP&A software with AI capabilities.
The first use case for AI capabilities needs to focus on an important business problem everybody cares about. Be prepared to take some risks. Empower a team who are passionate about getting the outcome and resilient to challenges, support them and follow through with change management.
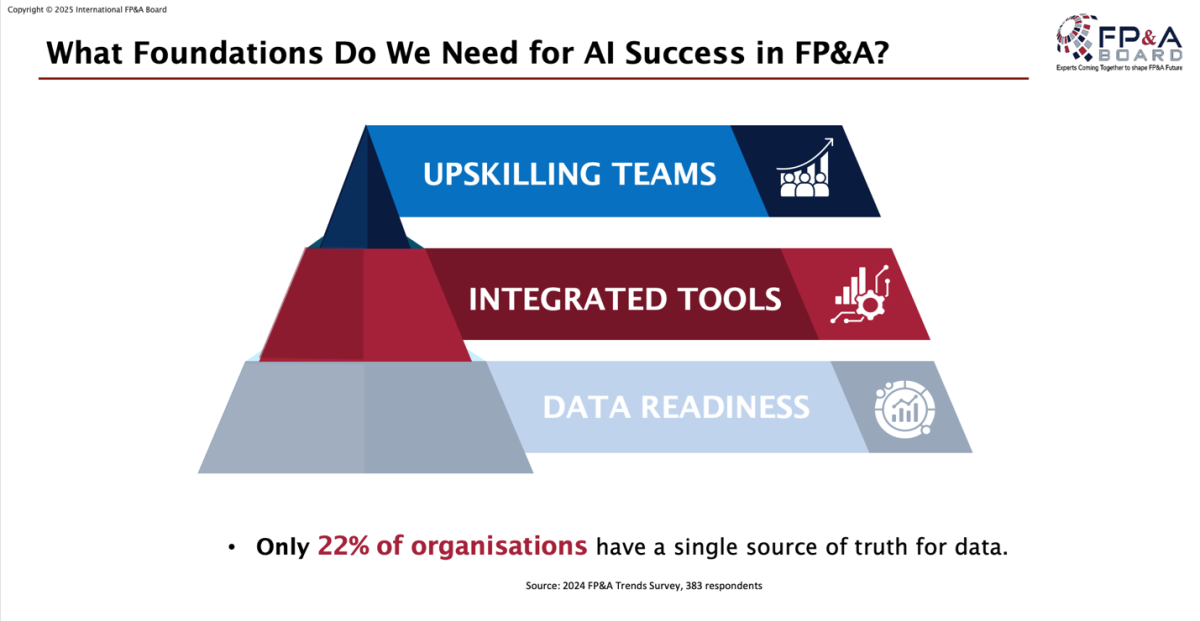
Figure 5
How Does AI Work Together with Human Intelligence?
AI does not replace Human Intelligence — it enhances it. While AI can process vast amounts of data, it lacks business acumen and intuition.
In FP&A, storytelling, strategic thinking, ethical judgment and creativity remain human-led activities. When using GenAI for reporting and presentations, finance teams must critically assess outputs, verify accuracy and apply governance frameworks to ensure responsible AI usage.
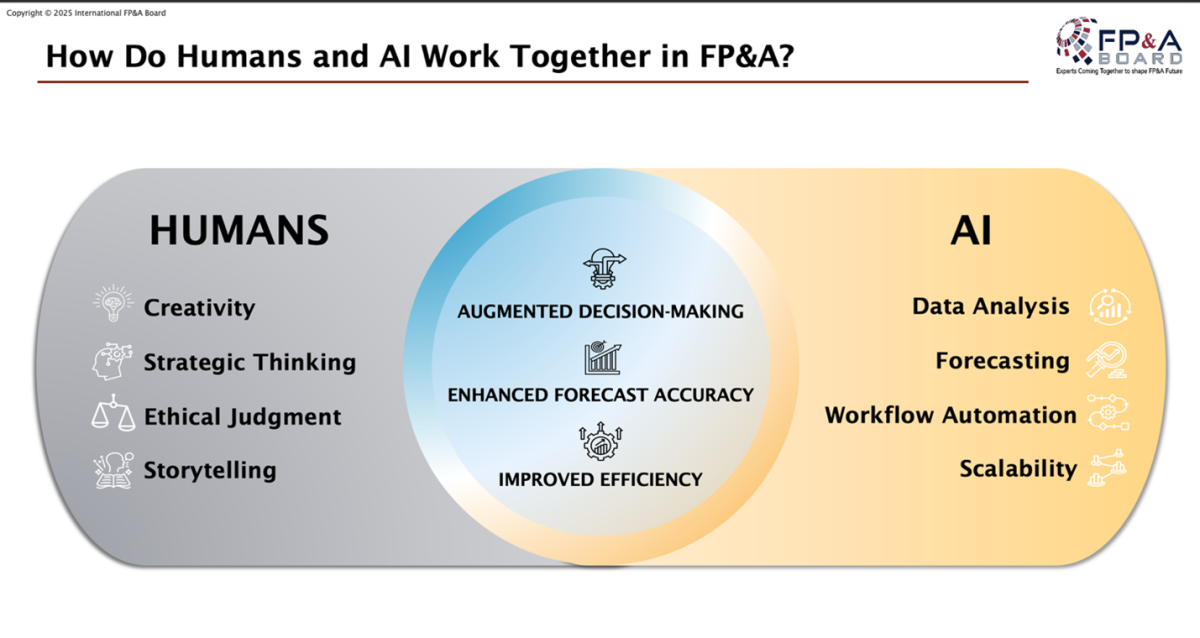
Figure 6
Practical Strategies for Integrating AI in FP&A
Tanbir Jasimuddin, Interim FP&A Transformation Director and FP&A Trends’ author, shared some practical strategies for integrating AI in FP&A.
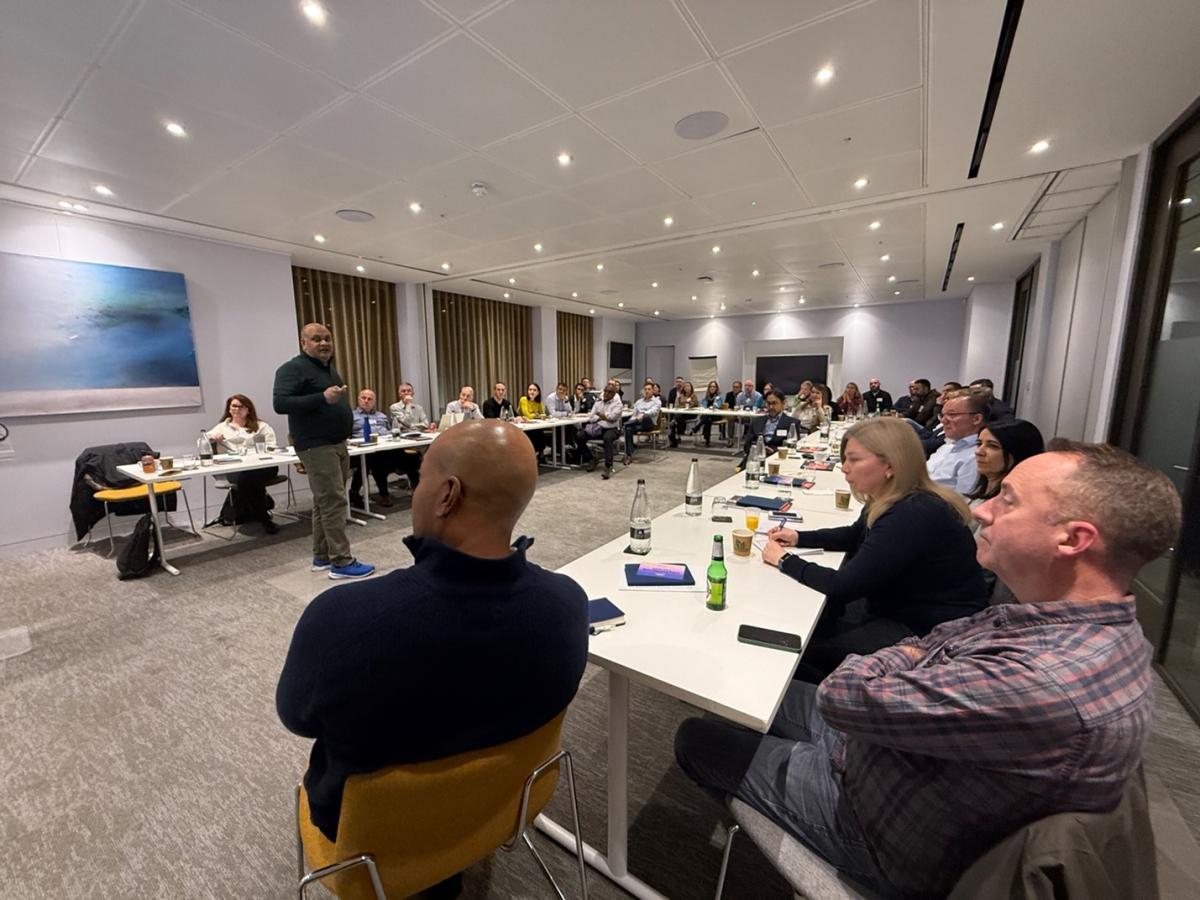
Figure 7. The 35th in-person London FP&A Board, January 23rd, 2025
Technology is not the barrier that it once was. Using new technology became significantly easier since the knowledge of a programming language is no longer a prerequisite.
What Can FP&A Teams Do to Accelerate AI Adoption?
1. Replace spreadsheets with a planning system
The 2024 FP&A Trends survey has found that most teams still use spreadsheets for planning, almost half spend time collecting and structuring data, while only 9% use fully driver-based models.
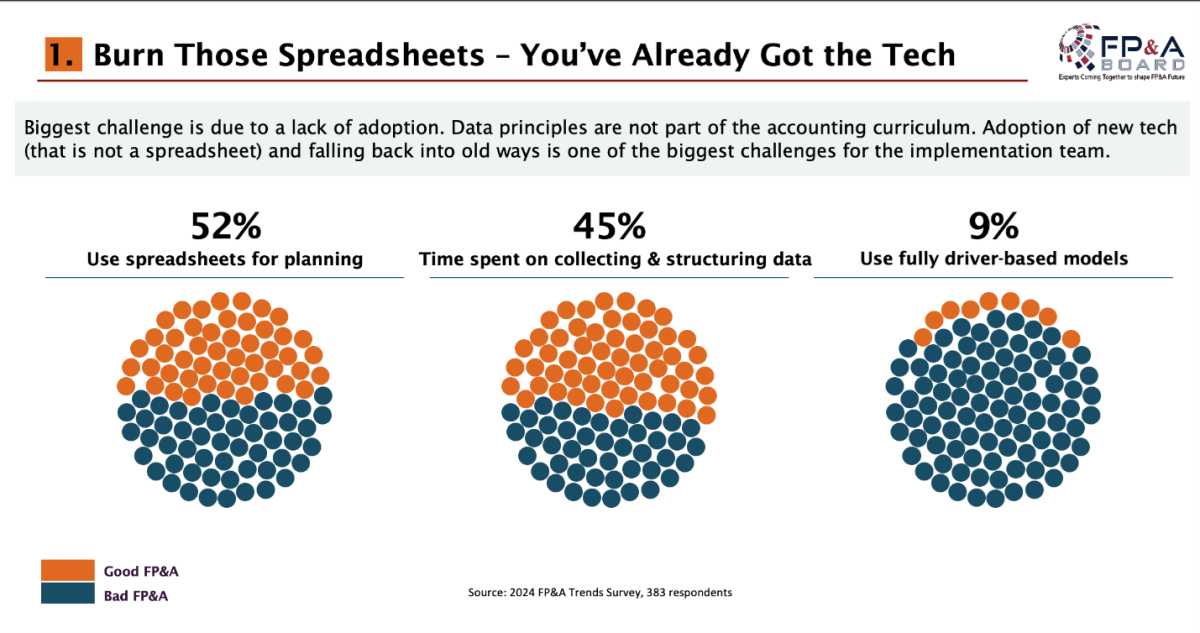
Figure 8
2. Create a common data platform
Governed, cleansed and curated data will form the foundation of AI adoption. Organisations with good data governance are 3-4 times more likely to deliver accurate forecasts.
3. Advance data quality
AI is only as good as the data that you provide. In the 2024 FP&A Trends Insights Paper on Mastering Data in FP&A, Tanbir Jasimuddin describes 3 steps that help achieve good quality data:
- Assign data accountability to the right teams;
- Establish one source of truth (‘The Golden Source’);
- Follow the data quality review process.
4. Invest in your team
The people aspect is the most important building block for AI adoption. Establish a cross-functional team, invest in your team and give your people time to learn and space to fail. New skills may include:
- Prompt engineering — how do you ask AI the right questions?
- Data governance — how do you deal with data ethically and responsibly?
5. Deploy technology in sync with data culture
AI implementation should evolve in parallel with organisational data literacy and data culture. If technology is deployed too fast, and people aren’t ready, they don’t use it. The opposite extreme is where teams become data literate much faster than the organisation’s systems can keep up with.
6. Get started
Encourage your team to start using GenAI products to understand their strengths and weaknesses, to be more productive, and to think bigger as to what is possible.
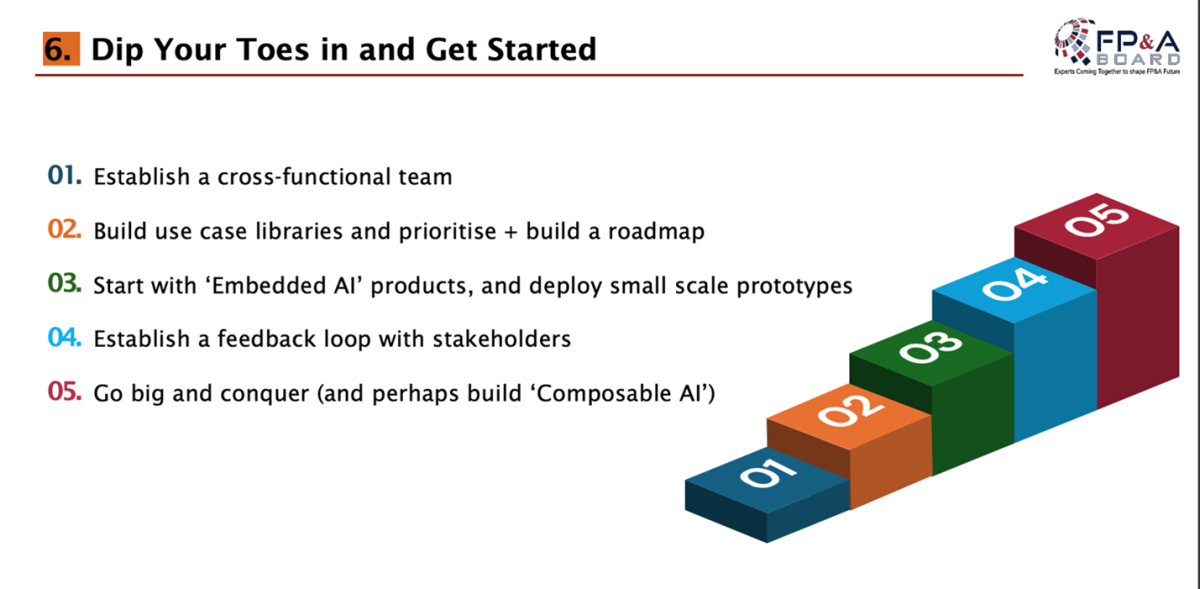
Figure 9
7. Continuous improvement mindset
To stay ahead, FP&A teams must continuously refine their processes, embrace innovation and foster a culture that adapts to ongoing technological change.
Modern FP&A Technologies with Embedded AI Capabilities
Michael Stanuszek, Account Executive at Workday, presented 3 examples of how a restaurant chain uses AI capabilities.
- Predictive Forecasting: AI-assisted forecasts help managers refine projections based on data-driven insights.
- AI Assistant: AI-powered chat interfaces enable quick financial analysis and decision-making.
- Variance Analysis: AI identifies data anomalies, allowing teams to proactively address potential issues.
Practical Implementation Steps
Participants split into three groups to discuss AI implementation strategies.
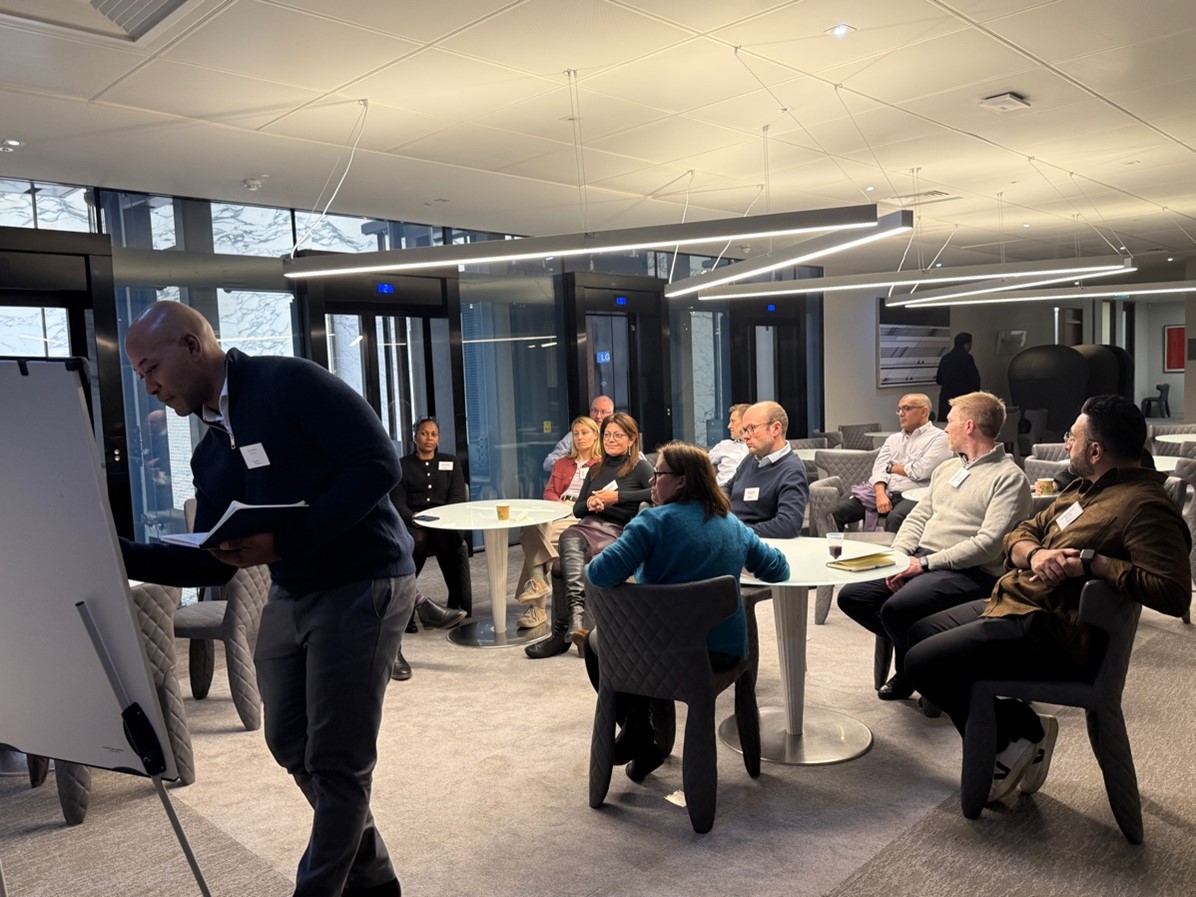
Figure 10
GROUP 1 — Generative AI Adoption
A maturity assessment within the group has revealed that 40% use AI.
Use cases include answers to common questions, variance analysis, recommendations on actions and strategic focus, decision-making support suggesting options and analysing pros and cons, productivity improvements, and producing first drafts of reports with commentaries which can then be fine-tuned.
The enablers include:
- Skills evolution
- Data (governance, access, quality)
- Securing trust in AI output
- Risk management (e.g. mitigating sensitive data leaks)
- A solid business case demonstrating the value and scalability of the AI solution, and return on investment
The success of AI adoption can be demonstrated by
- Accurate reporting and more reliable forecasts
- Time saved on producing actionable insights
- Efficiency gains and new capabilities
GROUP 2 — Machine Learning in FP&A
- Consider a wider vision for ML than just the Finance function. ML adoption will influence decision-making and impact people’s careers. A lagging behind technology-wise organisation will struggle to attract the right talent.
- Have a plan of what you are trying to do and why, which becomes the basis for the business case that can demonstrate the value.
- Engage stakeholders to identify additional use cases, and add additional investment requirements and respective value to the business case.
- Consider data structure and teams’ structure to support the journey.
- To implement machine learning, one needs at least 10 years of historical data. The quality of the data determines the quality of the output.
- Assess the skillset available to deploy the technology. Build a relationship with a third-party consultancy with a history of successful implementations to bring in the methodology for deployment.
- Perform a risk assessment and address fear, uncertainty and doubt with stakeholders to ensure the team is confident in achieving desired results.
- Ensure the diversity of talent within the team, maintaining the right mix of experience and new graduates passionate about technology.
GROUP 3 — Human + AI Collaboration
- Understand your data and align teams over definitions of each metric.
- Build internal capabilities to manage disparate data sources and governance to build trust in the AI output.
- Encourage business and IT to interact effectively. Avoid having each business unit or function purchase its own AI tools. A consistent approach across the company will add more value.
- Educate the business to build adoption, and inspire end users to learn more and understand the output.
- Where previously company culture may have involved everyone making corrections in their spreadsheet or slide, every user should provide feedback directly to AI so that technology offers a better output the next time.
- AI tools should enhance, not replace, human judgment and expertise.