This article aims to share some insights on why digital transformation in finance has failed in...
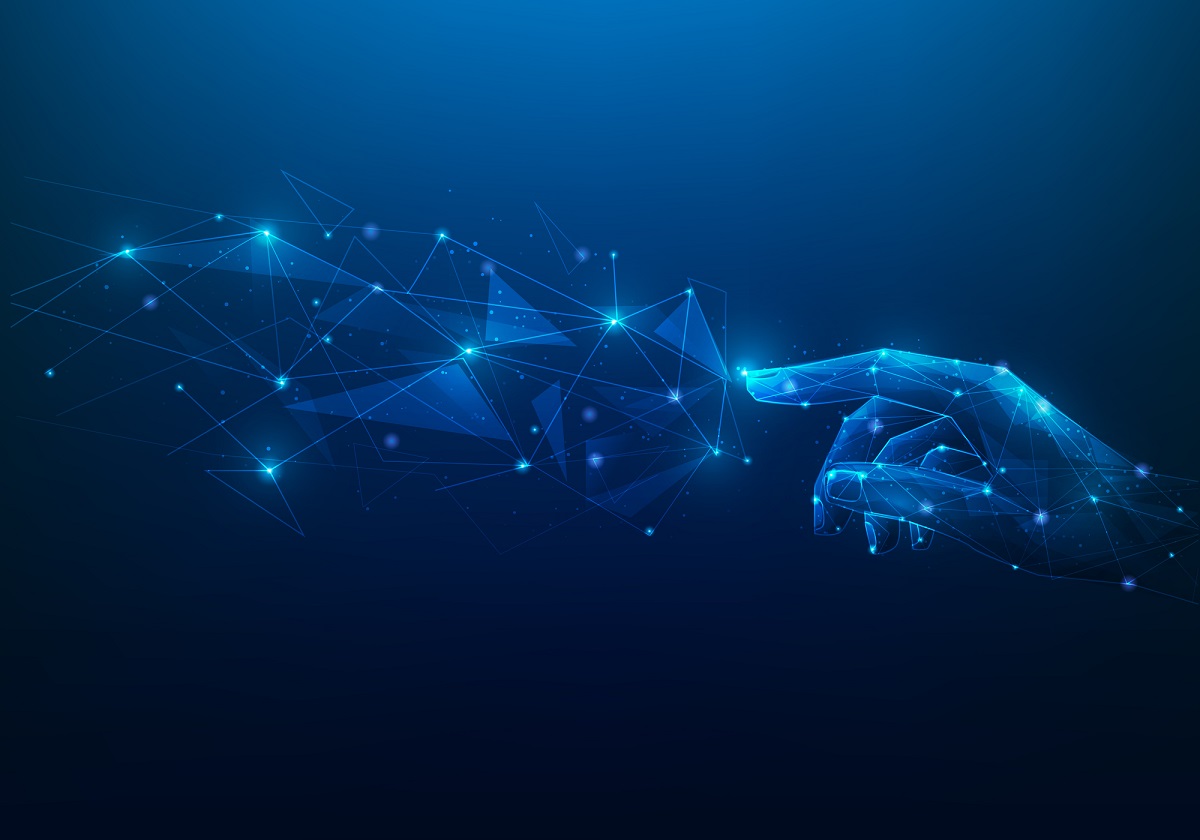
While digital transformation roundtables and events have somehow slowed down, the trend has moved towards the role of Artificial Intelligence (AI) in the future of the finance profession. Unfortunately, this has been driven by the limitless potential of technology and a few standout digital transformation projects in major companies, leaving many others behind. In fact, our profession needs to undergo a change in culture, which is the toughest in any Change Management process.
Post-Pandemic Challenges
The world has been moving too fast after the pandemic towards more IT-based solutions to deal with the vast datasets available within different systems in a corporation. The pressure to use data more efficiently and link it with financial Key Performance Indicators (KPIs) continues to grow, and this aligns with Integrated Reporting and the Global Reporting Initiative (GRI). Amid global economic challenges, shifting social expectations like work-life balance and Gen Z preferences, and the demand for cost savings due to inflation, there is increasing internal pressure to work efficiently by adopting technology and creating real-time scenarios with fewer resources. Our profession is stuck between all of those pressures, but we still have the capacity to learn, trust, and produce accurate reports using unproven technology and techniques.
Our culture as finance professionals is based on standardisation and well-established processes aimed at generating the most critical annual document for a commercial entity regardless of its legal form. The inherent control background in the process, coupled with the finance professionals’ backdrop, adds more difficulty and complexity to the process of change. Resisting change is in human nature, especially when you are learning something not at a young age and a senior level in professional life. These factors often limit creativity and create a less positive environment in a typical finance function.
Given the need for better analysis and Business Partnering, which wasn't provided at the required level, implementing data science was the closest solution. However, it made everything more complicated. The tension caused by finance teams refusing to use Key Performance Indicators (KPIs) from non-finance systems or only accepting them after strict vetting has hurt relationships between departments. In turn, it led to the budgeting process being focused on board approvals and seen as irrelevant to performance management.
The current situation does not differ much from the introduction of PCs, ERPs or spreadsheets and their impact on the finance profession. Still, I don't believe we are dealing with it in the best possible manner. We need to accept that the requirements are not the same as before and that FP&A professionals should change their skill sets. Finance functions need to find a common language with the IT community to be able to utilise the available tools. We need to be equipped with the required knowledge to make the choice, implement and operate such tools to tailor to organisational needs. It seems to be limited now to sales seminars and cases that might not be related to other organisations.
The Roadmap to Digital Transformation in Finance
I do believe that a “Real Option” approach to such decisions allows FP&A leaders to sample, pilot and make a strategic long-term decision on the way forward. I see that free Microsoft tools offer finance leaders full visibility into micro-level operational inefficiencies and redundancies, providing a flexible environment to document inputs, processes, and outputs while working on possible solutions. The latest part related to the documentation should provide the audit trail to vet inaccuracies, the required transparency for automating audited accounting data models, and, finally, serve as the business analysis material if an organisation decides to go for a developed paid tool.
Digital transformation is an enabler and not a goal. It enables us to eliminate redundancies, repetitions and long working hours. It gives a finance function the capacity to answer questions and ad-hoc requests on the same day or even during the same meeting. The road map to that starts with the mindset change. We must accept that the finance skill set of now and the future requires an understanding of database structures, the basics of data science and relevant programming languages such as DAX and Python. Understanding the limitations of other systems and how to bridge and document different variances between other systems and General Ledger is crucial for achieving the “Holy Grail" - “One Source of Truth”.
I have gone through very successful use cases in two companies, and I can outline a roadmap to guide finance leaders in their digital transformation initiatives:
1. Formulate a vision about the part of the business to be transformed.
2. Identify which elements need to be sourced internally and others to be outsourced. This will be based on the elements requiring governance and secure systems, such as Accounts Payable, Accounts Receivable and others, that can be outsourced compared to the elements requiring flexibility and experience to mature, which should be sourced internally.
3. Define the available talent pool in your team. Approach the tech-savvy team members and onboard them to your vision.
4. Invest, motivate, and incentivise tech skill acquisition and improvement.
5. Start identifying the required operational databases and the relative financial sub-ledger or trial balance lines to be linked to.
6. Discuss with the operations team the specifics of the database and identify:
- Identifier columns: Key data that could be used in reconciliation or linking to other databases.
- Closing calendar-driven variances.
- Accurate value columns to allow close to accurate analytics and estimates. The examples of that can be as follows:
6.3.1. Stored supplier price list vs. actual purchase order (PO) value
6.3.2. Database currencies
6.3.3. Inventory value vs. company valuation policy
6.3.4. Estimated receipt date vs. PO actual lead time
7. List down the values and analytics to be captured from the new database, including mixed operational and financial ones.
8. Decide on the technology approach: DAX, Power Query, Power Pivot, Power BI, Tableau, Python, etc.
9. Break down your calculations into steps to allow extracting the required data points by finance and operations. This step should allow scenario simulation based on the factors impacting business operations.
10. Decide on the technology to be used and the medium to keep the data model on, such as a shared drive, local server, cloud solution, and so on.
11. Test on capable devices and share results with the operations team.
12. Build reports and dashboards that would serve financial and operational purposes.
13. Decide the best approach for update: report extracts vs. database connection.
14. Involve IT to help with pre-soft launch requirements: server/database connections, computing capacity requirements, software licenses, etc.
15. Train users on using the newly available dataset:
- Finance team to build reports and possible ad-hoc requests.
- Operations team to build reports and update dashboards.
- Discuss and prove with data counterintuitive findings resulting from connecting the two datasets and quickly drill down into a longer-time period results.
16. Agree on new KPIs and new performance management processes.
The above journey should provide involved functions with enough insights about specifics and challenges the other functions face and equip finance Business Partners with a wealth of operational knowledge, which, in turn, should make their job in challenging forecasts more successful. The acquired knowledge should equip finance Business Partners with an understanding of drivers impacting the forecast accuracy and business scenarios. The above process will be documented in the data model and should serve the required business analysis if a more secure vertical is required after the process has matured.
Conclusions
Finally, the transformation of the skills required from finance professionals is the answer to all the challenges we are facing in post-pandemic corporate life. It will modernise the FP&A practice, enable data visualisation, automate and centralise critical processes, and will be the vehicle for moving to AI-assisted finance operations.