In this article, the author explains three key strategies for fostering a data-driven culture in your...
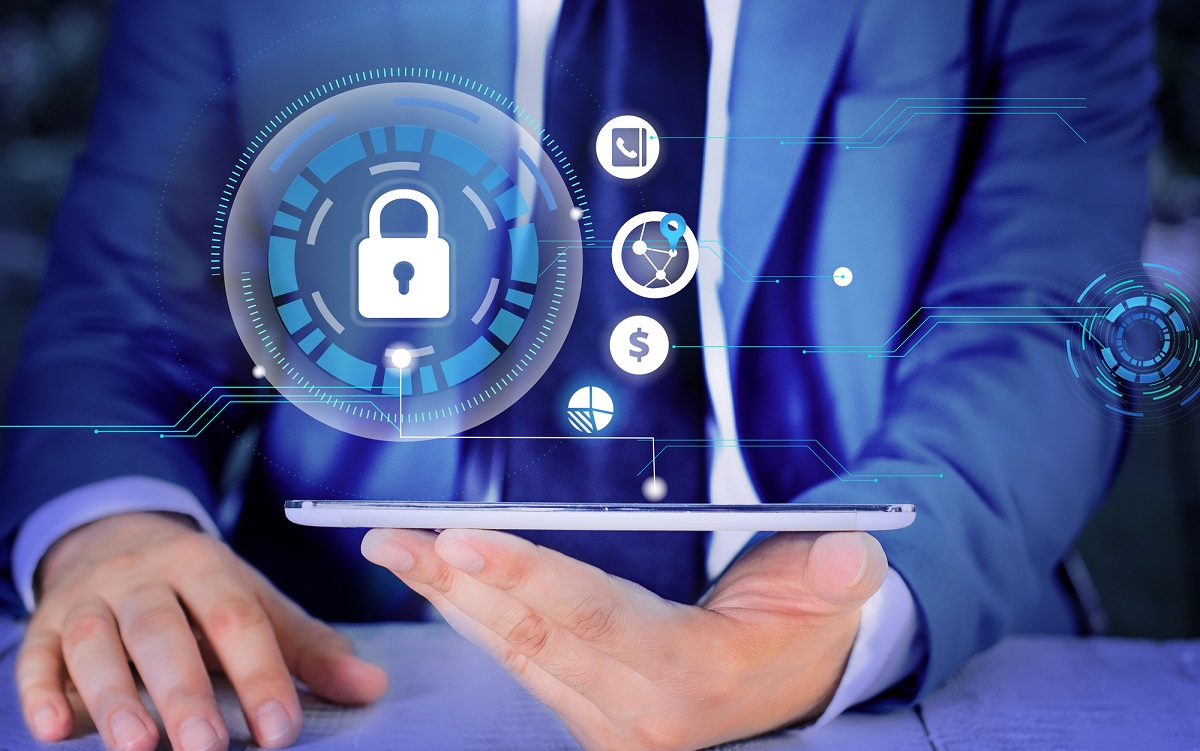
In today's fast-paced business environment, accurate forecasting is essential for companies aiming to maintain a competitive edge and drive strategic decision-making. The transformation journey to enhance forecasting capabilities hinges on the effective use of internal and external data.
This article delves into the importance of data in forecasting, managing data accuracy and completeness, common mistakes companies make, transformative opportunities presented by next-generation Artificial Intelligence (AI), and the role of the CFO in this process.
Why Do We Need Data?
Data serves as the foundation for precise forecasting, enabling companies to predict future trends and make informed decisions. Internal and external data are two critical sources that provide comprehensive insights.
Internal data includes historical sales data, inventory levels, and customer behaviour. Such data is invaluable for understanding past performance and current operations and offers a detailed view of the company's internal environment.
External data encompasses market trends, economic indicators, and competitor analysis. This data type is crucial for understanding broader market dynamics and anticipating future trends. By integrating external data, companies can gain a more holistic view of the factors influencing their business environment.
Accurate and complete data is essential for reliable forecasting. Data management and transformation processes, including data cleansing, integration, and validation, ensure high-quality data. Accurate data allows companies to make precise predictions, while complete data provides a comprehensive view of the factors influencing these predictions.
Despite the importance of data, many companies make critical mistakes that undermine their forecasting efforts:
- Poor data quality: Inaccurate, incomplete, or outdated data leads to flawed forecasts, impacting decision-making and Strategic Planning.
- Lack of integration: Failing to integrate internal and external data effectively results in a fragmented view, limiting forecasting accuracy.
- Inadequate data management practices: Insufficient data governance and lack of standardised processes hinder the ability to maintain high-quality data.
- Overreliance on historical data: Ignoring external factors and emerging trends can result in forecasts that do not account for future changes.
Next-generation AI offers transformative opportunities to enhance forecasting accuracy. AI and Machine Learning technologies can analyse vast amounts of data and identify patterns and trends humans might overlook. Predictive Analytics, anomaly detection, and trend analysis are just a few of the capabilities AI brings to the table. Additionally, AI can automate and optimise data management processes, ensuring data accuracy and completeness while reducing manual effort.
Adopting a data-centric approach yields significant long-term benefits:
- Enhanced decision-making: High-quality data and advanced forecasting models lead to better strategic decisions, improving business outcomes.
- Increased agility: Companies can quickly adapt to market changes and emerging trends, maintaining a competitive edge.
- Cost efficiency: Accurate forecasts help optimise resource allocation, reducing waste and improving profitability.
- Customer satisfaction: A better understanding of customer needs and behaviours leads to improved products and services, enhancing customer loyalty.
Conversely, companies that overlook the importance of data face several adversities:
- Inefficient operations: Poor forecasting leads to inefficient operations, such as overstocking or stockouts, negatively impacting profitability.
- Missed opportunities: Lack of insights into market trends and customer preferences results in missed business opportunities.
- Competitive disadvantage: Companies that fail to leverage data effectively fall behind competitors who use data to drive their strategies.
- Reputational damage: Frequent errors and misjudgments due to poor forecasting can harm a company's reputation, affecting customer trust and loyalty.
Championing the Journey to Data-Driven Insights
The CFO plays a pivotal role in the transformation journey. As a strategic leader, the CFO ensures that data-driven forecasting aligns with the company’s financial goals and strategies. Their responsibilities include:
- Championing data-driven culture: Promoting the importance of data across the organisation and ensuring investment in necessary tools and technologies.
- Data governance: Establishing robust data governance frameworks to maintain data quality and integrity.
- Cross-functional collaboration: Facilitating collaboration between IT, finance, and other departments to integrate data effectively.
- Strategic oversight: Using data insights to guide financial planning, budgeting, and risk management.
Practical steps for a successful transformation should include but not be limited to:
- Assess current capabilities: Evaluate the existing data infrastructure and identify gaps.
- Develop a data strategy: Create a comprehensive data strategy aligning with business objectives.
- Invest in technology: Implement AI and Machine Learning tools to enhance data analysis and forecasting.
- Foster a data-driven culture: Encourage data literacy and promote a culture of data-driven decision-making across the organisation.
- Engage stakeholders: Involve IT, data analysts, and business leaders in the transformation process to ensure alignment and get buy-in.
A Practical Use Case in an FTSE 50 Company
A FTSE 50 company, GlobalTech, struggled with fluctuating profitability due to inaccurate demand forecasting. This was caused by fragmented data systems and an overreliance on historical internal data, leading to misaligned production schedules and inefficient resource allocation.
The challenges faced were as follows:
- Fragmented data: Sales, production, and market data were not fully integrated, resulting in incomplete forecasting models.
- Outdated forecasting methods: The company relied on traditional forecasting models that did not account for real-time external factors like market shifts and competitor actions.
- Operational inefficiencies: Inaccurate forecasts led to overproduction in some regions and underproduction in others, affecting profitability.
Their CFO led a strategic transformation to enhance forecasting accuracy and drive profitability.
- Data integration: GlobalTech implemented an advanced data integration platform that unified internal data (sales, production, financials) with external data (market trends and economic indicators).
- AI-driven forecasting: The company adopted AI and ML tools to analyse the integrated data, enabling more accurate and dynamic forecasting models.
- Cross-functional collaboration: The CFO promoted collaboration between finance, operations, and IT to ensure the forecasting models were aligned with corporate strategy and market realities.
Outcomes
- Increased forecast accuracy: Forecasting accuracy improved by 40%, leading to better alignment of production and demand.
- Enhanced profitability: Optimised resource allocation and reduced waste resulted in a 15% increase in profitability within the first year.
- Strategic agility: The company became more agile, quickly adjusting production and supply chain strategies in response to market changes.
Conclusion
By integrating internal and external data, leveraging AI, and fostering cross-functional collaboration, GlobalTech significantly enhanced its forecasting accuracy and profitability. The CFO’s role was pivotal in aligning these efforts with the company’s broader financial and strategic goals, demonstrating the critical impact of data-driven decision-making in a large, complex organisation.
Both internal and external data play a crucial role in improving forecasting capabilities. Managing data accuracy and completeness is paramount for reliable predictions. By addressing common data-related mistakes and leveraging next-gen AI, companies can significantly enhance their forecasting accuracy and make more informed decisions.
In addition, embracing a data-centric approach offers long-term benefits, including enhanced decision-making, increased agility, cost efficiency and improved customer satisfaction.
Conversely, overlooking the importance of data can lead to inefficiencies, missed opportunities, competitive disadvantages, and reputational damage. With the CFO's leadership in championing data initiatives, companies can navigate their transformation journey effectively and achieve sustainable success.