In this article, the author exemplifies why Artificial Intelligence is insufficient for analysing the data and...
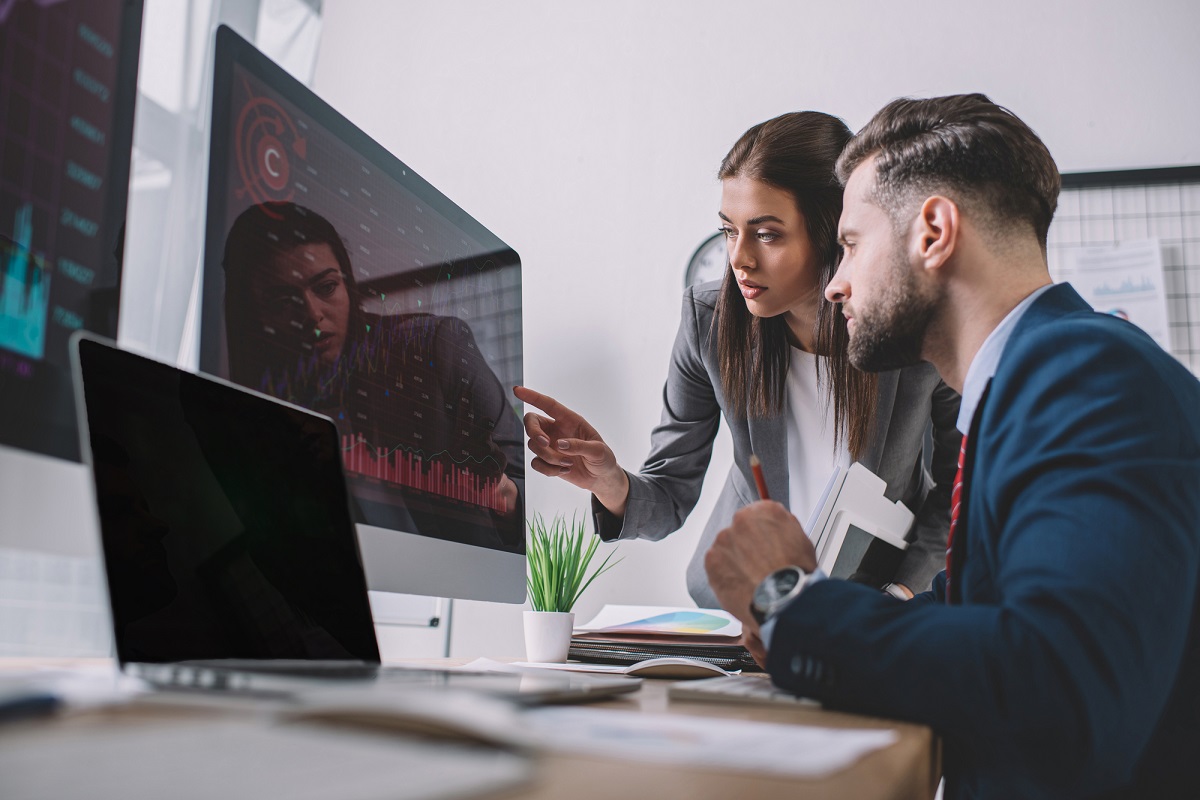
Organisations striving for automation and streamlining human resources rely on systems and data from different sources. The trend in implementing robotics and automation is modern and progressive. At the same time, the reliability of taxonomy and consistency of the data is a prerequisite for applying automation.
Maintaining data consistency is crucial for the forecasting process to ensure the accuracy, reliability, and credibility of financial information.
FP&A teams encounter significant challenges in ensuring the accuracy of data that feeds forecasting models. The evolution towards Extended Planning and Analysis (xP&A) exacerbates these challenges, particularly due to the incorporation of non-financial parameters into analytical systems. How can we, as FP&A professionals, mitigate the issues related to data consistency?
Data Consistency Challenges
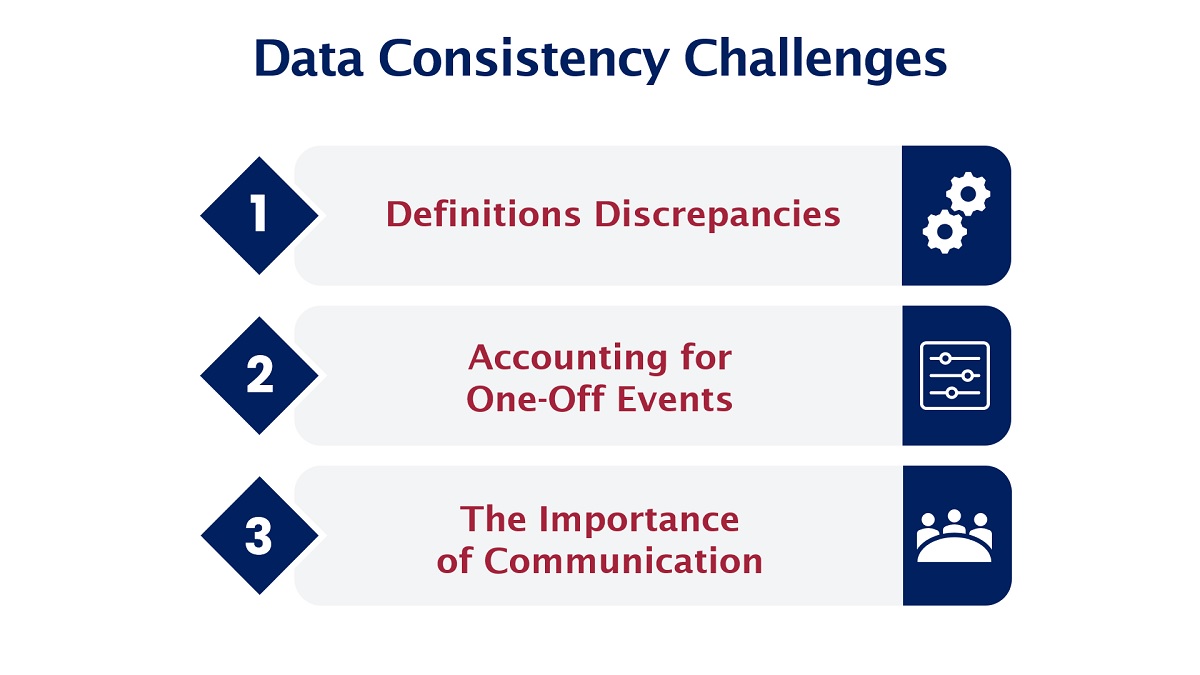
Figure 1
Key challenges related to data consistency include the following:
Definitions Discrepancies
Variations in the timing of transaction recording across sources pose a significant hurdle.
For instance, discrepancies in revenue recognition parameters can represent:
- status of an order to be recorded as revenue volume (at which time the sale is recognised)
- discount types classification (to be included or excluded from revenue volume)
- sales channel analytics classification
These discrepancies can lead to inconsistencies in reports containing sales revenue lines and other non-financial data, such as number of orders, sales per order, etc.
Solution
Define clear and consistent definitions for budget/forecast items, metrics, and assumptions across departments and stakeholders. It can help avoid misunderstandings and discrepancies in data interpretation. Document all data sources, calculations, assumptions, and methodologies used in the reporting and budgeting/forecasting process. Documentation helps us understand the rationale behind budget/forecast figures and ensures consistency.
Accounting for One-off Events
Forecasting based solely on historical actuals may lead to erroneous conclusions, especially when one-off events significantly impact data. The algorithms relying on actuals may project the recurrence of such events inaccurately. Manual and time-consuming efforts are often required to identify and address these impacts.
Illustrative Case
An office rent discount provided during the COVID-19 pandemic reflected as a current operational item within standard Profit and Loss (P&L) lines for accounting and financial reporting may mislead future forecasts. The algorithms used for Rolling Forecasts, set to compute the average of the previous twelve months, erroneously predict the repetition of positive impacts that are not anticipated.
Solution
The introduction of a separate line for one-off events within the forecasting model and the split of actuals to accurately reflect baseline and one-off impact ensures accuracy. The definition of one-off items is vital for implementation. The FP&A team’s responsibility is to define and communicate solutions to stakeholders.
1. We have to implement procedures to validate and clean data before incorporating it into the forecasting process. This includes identifying and correcting errors, inconsistencies, and duplicates in the data. Let us take a one-off event case, for example. Discount recorded as a P&L cost decrease. The forecasting algorithm: prior 12 months with inflation adjustment.
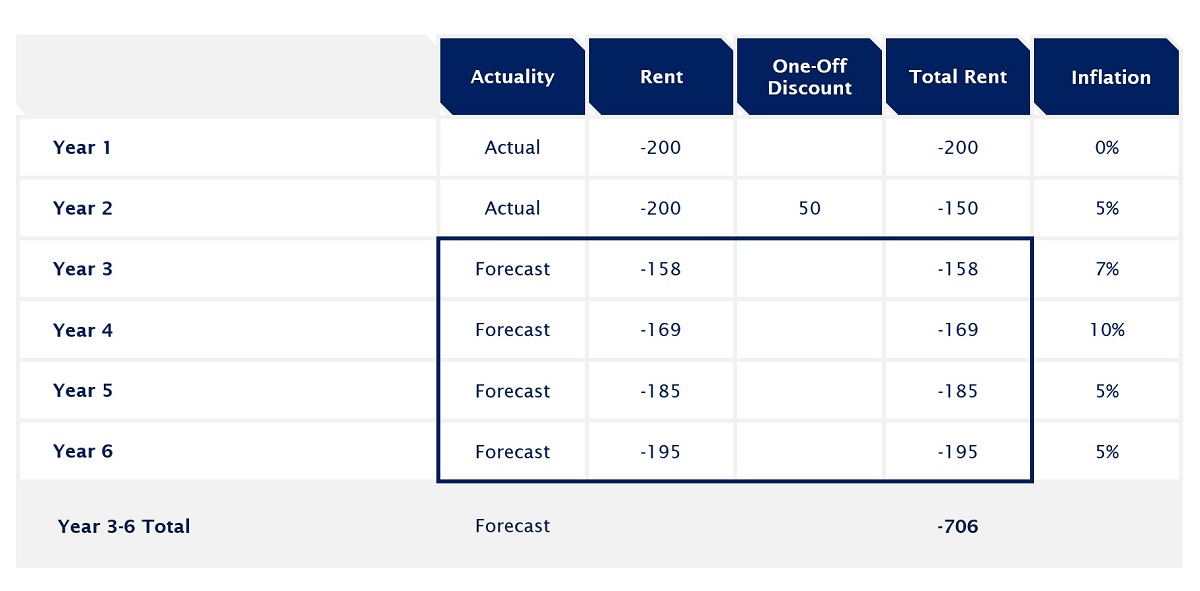
Figure 2: An Illustrative Case on Rent Discount as One-off Event
2. Separate line for one-off events introduced. Discount is not included in an actual base for forecasting. The forecasting algorithm: prior 12 months with inflation adjustment.
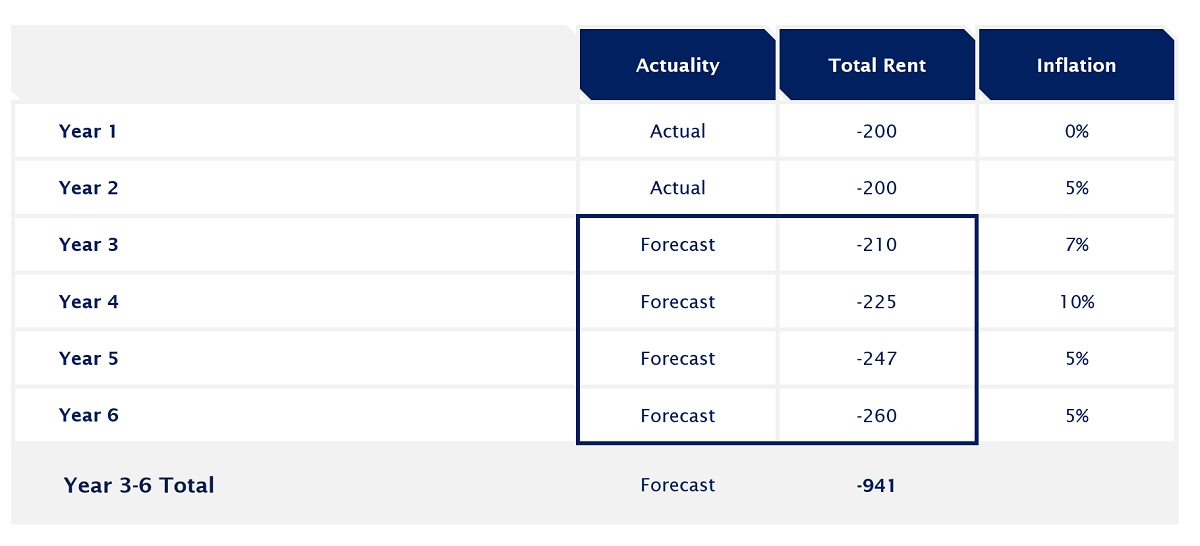
Figure 3: An Illustrative Case on Rent Discount as One-off Event
The forecast includes full cost without repetition of one-off discount.
Cost underestimation in Case 1 is 33% as the model’s algorithm predicts the possible repetition of discount. Depending on the algorithm, damage can be more significant. If a one-off event happens in a certain quarter and the forecasting model predicts repetition of it in the next quarter, it can be multiplied.
FP&A teams continue to encounter challenges and lack the tools to correct the baseline in the automatically estimated forecasts. The results are often downloaded into Excel and adjusted manually, consuming valuable time. The solution of adjusting the base via source data management ensures effective usage of the automated model, saving the time and effort of the FP&A team.
Importance of communication
In the dynamic environment of changes and a variety of systems and reports, it becomes vital to properly communicate any adjustments of the definitions to relevant stakeholders. Potential causes can include:
- Change of accounting treatment for existing process
- Introduction of new processes (driving the necessity for revised definitions)
- Organisational restructuring impacting cost centre structures
- Redesign of reports
Solution
Establish governance process for systems and reports and communicate its principles clearly to relevant stakeholders. Maintain a comprehensive knowledge base covering definitions and available reports, ensuring it reflects all changes and adjustments introduced. Providing timely information about the changes is crucial to ensure alignment among stakeholders.
Conclusions
In the environment of automation, visibility of algorithms and transparency of data definitions disappear, leading stakeholders to prioritise trustworthiness in systems. Consequently, the significance of financial information credibility increases.
By prioritising these aspects, organisations can establish a framework for maintaining data consistency in the budgeting and forecasting process, sourcing automated forecasting models with reliable data, which, in turn, can lead to more accurate financial forecasts and informed decision-making.