Mastering AI in FP&A isn’t just about technology — it’s about overcoming human resistance to change...
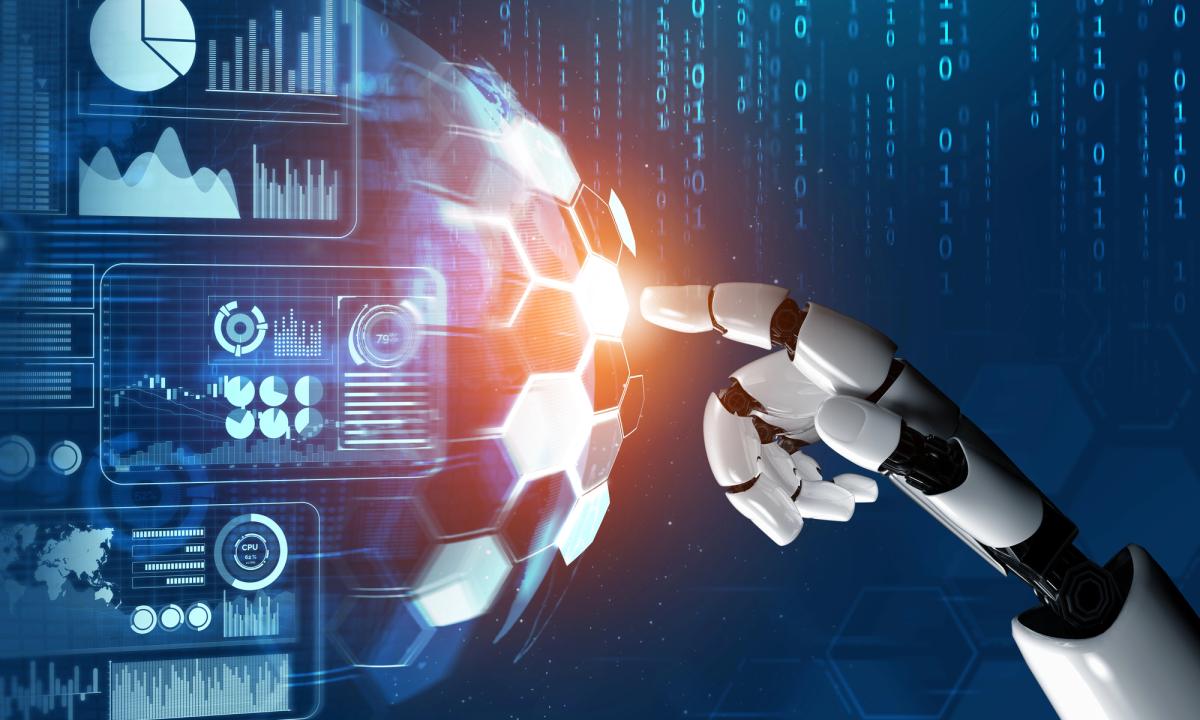
Artificial Intelligence (AI) and Machine Learning (ML) are not new technologies. However, what is new is FP&A’s adoption of AI and ML in supporting management decision-making.
In the latest FP&A Trends Survey, although only 6% of FP&A departments claim to currently be using AI/ML technology, a large proportion (59%) said they would be exploring its use in the near future. This surge in interest can be attributed to the rising need for improved forecast speed and accuracy in the existing unpredictable business environment. The survey shows that, on average, 42% of organisations rate their forecasts as great or good, but this statistic increases to 65% for those organisations that are using AI/ML.
The improvement in forecast accuracy is derived through AI or ML’s ability to uncover the underlying performance drivers, from large internal and external data sets, and then predict their future values. The results are then used to forecast outcomes within driver-based models. Higher forecast accuracy is, therefore, now something made easier through the availability of data and AI/ML technology solutions aimed at the finance community.
How AI/ML Is Used in FP&A
Machine Learning (ML) is a branch of Artificial Intelligence (AI) that utilises algorithms — sets of instructions for data analysis. These instruction sets, or algorithms, are self-adjusting based on the results of previous analyses, therefore creating a recursive process which inspires the name 'machine learning.' The processes of data analysis, drivers prediction and forecast generation can be automated, thereby eliminating a lot of manual effort and time.
One company we interviewed mentioned that through AI/ML they had cut down their annual forecast process by approximately 30,000 full-time equivalent (FTE) hours. They can now identify in real-time the factors that shape their business in specific markets, a task that previously took two months.
Another company we spoke to found that AI/ML predictions are better than manual forecasts by 1.6%. Yet, the real benefit lies in the time and effort saved. Today, this company can run a revised forecast off of a new set of sales figures and generate 80% of the full P&L automatically in around 2-3 hours. Previously, this would have taken the team several days and involved around 200 people.
Consequently, both companies found that due to their use of AI/ML, the FP&A teams now spend more time on business partnering and driving actions. As one executive told us, “What we are now seeing is FP&A skills transformation.”
Avoiding the Problems Associated with AI/ML
As with any new technology, there are challenges to overcome. The best approach involves running a pilot study to experiment and learn the intricacies of the technology as well as prove the worth of AI/ML to the broader organisation. Our research indicates the following issues and solutions:
1. Culture
Adopting AI/ML means a change in the way things are done and a required focus on the real performance drivers. All of the companies we interviewed told us that C-level support was essential, and that management needed to move towards fact-based decision-making for the adoption of AI/ML to be successful.
2. Trust
AI/ML is often seen as a black box that few people understand and can also be seen as a threat to management independence. In reality, AI/ML is a powerful management tool that can greatly help management make better decisions. A successful pilot project that verifies the technology will go a long way to diminish fears.
3. Data Quality
Repeatedly, we find data quality to be a major issue. Our recent survey found that only 22% of organisations have one single source of data they can rely upon. This was also considered to be the biggest issue that FP&A departments face when planning. Data issues must be addressed, even if only to cover the pilot project.
4. Technology Systems
To analyse the large and diverse quantities of data, a modern technology platform is required. The spreadsheets that still dominate FP&A planning, are simply not good enough. This becomes obvious when we look at an organisation’s ability to run scenarios which are effectively the bedrock of planning in any uncertain environment. The latest FP&A Trends survey found that only 22% of organisations can run scenarios in real-time or less than one day, while 21% are unable to run scenarios at all.
Adopting AI/ML means implementing a solution on a recognised planning platform that has embedded AI/ML capabilities, and that allows for the development of driver-based models. Fortunately, there are many low-cost cloud-based systems available that are quick and easy to use.
5. Expertise
Using AI/ML needs expertise in algorithm selection and application, as well as result interpretation. This is where the role of an FP&A Data Scientist comes in — someone who acts as the bridge between the finance and data science functions. When approaching the implementation of AI/ML, for the first time, interviewees recommended starting out with a small team of experts. Typical teams are cross-functional and include, the already mentioned, role of the FP&A Data Scientist, one business manager from the selected pilot area, one IT person to manage the data, and one Data Scientist to choose and code the algorithms.
What AI/ML Means for FP&A
AI/ML algorithms are a game changer in that they allow FP&A to be more proactive within the business. In essence, AI/ML elevates FP&A from the role of analyst to that of an effective business partner.
AI/ML is here to stay and will only become more critical as data and uncertainty grows. The question for FP&A is do they want to be part of the revolution or will they fade into irrelevance.
This article was first published on the SAP Blog.