Artificial Intelligence (AI) is one of those terms surrounded in mystery and which promises a lot...
Without a doubt, Artificial Intelligence can improve the speed and quality of our work. To what extent is it valid for Financial Planning, Budgeting and Forecasting? Can we completely delegate those jobs to modern technology, or is the human being still an irreplaceable part of the equation?
The Digital Middle Eastern FP&A Circle has attempted to answer key questions related to deploying AI/ML in FP&A: does it improve accuracy? What is inside the black box? How does it affect FP&A team size and skillset?
This article provides an overview of the topics and cases presented and discussed by the expert panellists in the "Deploying AI/ML for Planning, Budgeting & Forecasting" webinar, as well as the results of our polling questions.
Machine Learning for Forecasting and Budgeting
Wibe Spekking, CFO at Microsoft Netherlands, has shared a Microsoft case study and has demonstrated how the product revenue forecasting process has changed after Machine Learning Technology implementation.
Wibe has started by outlining the main challenges before ML and the opportunities new technology brings:
- ML allows us to overcome conscious and unconscious bias using a highly accurate statistical model.
- ML allows us to improve forecasting speed dramatically (from 3 weeks to 30 minutes in this case study).
- ML allows us to increase finance teams' efficiency allowing hundreds of analysts to focus on higher ROI projects rather than spending hours preparing forecasts.
Building an ML-based forecast takes much time, but the results are certainly worth it:
Figure 1: Using Machine Learning for Product Revenue Forecasting in Microsoft
There are several steps Microsoft has learned on the way, and they are certainly with to consider when you start implementing ML in your organisation:
- Have conviction on ROI and other benefits.
- Stakeholder buy-in is essential – the tone from the top and the leadership vision are crucial.
- Think about where centralisation makes sense.
- Try, fail, learn, improve – it takes time before ML-based forecasts become more accurate than FP&A team forecasts, and it is worth running both processes in parallel to convince the stakeholders.
- Understand the Machine Learning "black box".
- Machine Learning needs to be incorporated into finance processes.
- Close partnerships with finance + data scientists are needed – human interaction is still required to provide inputs and interpret results.
How Does ML Deployment Affect FP&A Team Size?
During the webinar, attendees from the FP&A industry participated in the survey and stated how the size of their FP&A team had changed after the introduction of AI/ML. 48% of respondents said team size had stayed the same, while 34% had decreased.
Figure 2: Changes in FP&A Teams after Implementing Machine Learning
Next Generation Forecasting
Gizelda Ekonomi, Financial Planning Transformation Senior Manager at Vodafone, shared a practical case study from Vodafone on a Hybrid Product Management Approach to Planning.
Gizelda has shared with us common challenges in Financial Planning:
- Low level of financial modelling automation in Financial Forecasting, mainly dominated by Excel.
- Limited use of Predictive Analytics & Machine Learning.
- Commercial planning is not always fully integrated with Financial Planning.
AI can aggregate and process data with far greater speed, accuracy, and scalability than humanly possible. However, it is important to understand the "What", the "How", and the "Why" of AI-based forecasts.
The Product development approach helped the Vodafone team identify the Top 5 feedback themes of the FP&A community on the implementation of ML in FP&A:
- Explainable AI – understanding ML and how it affects the financial drivers.
- Accuracy of ML forecasting vs humans is key to gaining trust and understanding value.
- In a world of uncertainty, FP&A needs to look beyond the past – scenario-based planning.
- Create actionable insight through data modelling and visualisation.
- More independence for the FP&A teams to train and run ML models.
Collecting that information helped the team to create a Hybrid Product Management Approach To Planning which combines automation and human input:
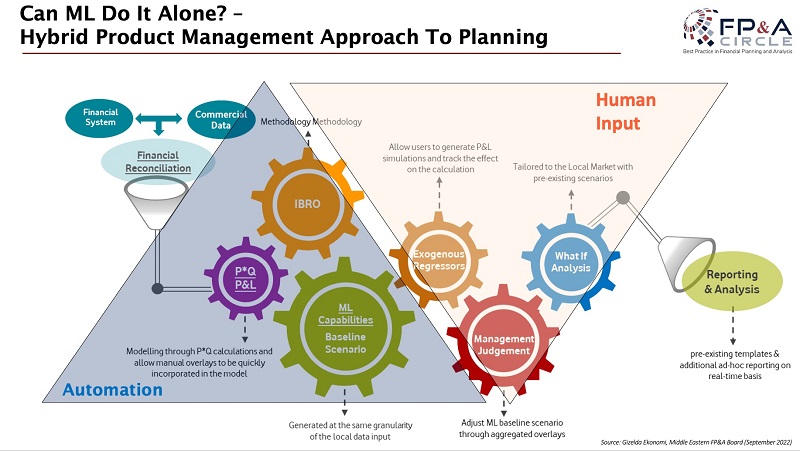
Figure 3: Hybrid Product Management Approach to Planning
Leveraging AI-based Analytics for Agile Planning in FP&A
Muhammad Zeeshan Taqi, Managing Partner, FP&A at Hub of Finance Transformation, presented a series of case studies where planning accuracy was tremendously improved thanks to AI-based analytics.
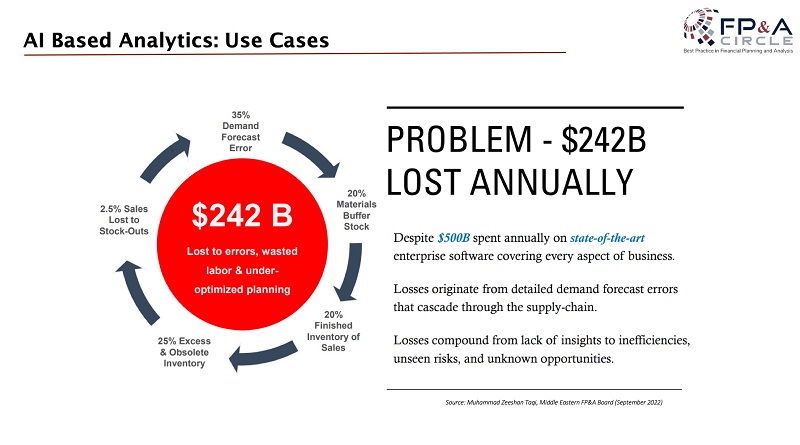
Figure 4: The Use Cases of AI-Based Analytics
- In the 1st case, demand forecasting accuracy was improved from 65% to 95% thanks to AI-based technology.
- In the 2nd case, the sales propensity score was introduced to focus on the "right" deals and deliver higher-quality revenue.
- In the 3rd case, AI helped to generate four times4x more billing with only 30% more products.
- The 4th case allowed the identification of up to 8Mm USD of previously unseen demand opportunity.
- In the final 5th case, multiple optimisation effects were achieved, including a 50% reduction in chargebacks and a 5% sales uplift.
Muhammad has summarised his learnings from his vast experience with AI-based forecasting tools in the following five points:
- Analytics mitigates human bias to yield insights for better decisions that improve revenue and cost management.
- Analytics can mitigate the $242B lost to under-optimised planning.
- However, analytics can be a "heavy lift" because skilled people are needed to connect tool features with loaded data.
- AI-Based Analytics automatically connects its capabilities with the data to deliver fast, valuable insights for better decision-making and planning.
- AI-Based Analytics can be applied across demand forecasting, inventory, supply chain, operations, finance and sales.
In which FP&A Areas Do We Use AI/ML?
Budgeting and forecasting are leading areas of FP&A, where most of our respondents (42%) use AI or ML. Our panellists also see this as the area where FP&A can benefit most from implementing AI/ML. AI and ML also have great potential in scenario planning and business analytics.
Figure 5: Different Areas where FP&A Teams Can Use AI/ML
Practical Insights for Successful Implementation of AI/ML
Andreas Simon, Regional Director MEA at Jedox, has shared quite a practical 6-step approach to AI implementation with us.
Figure 6: Six Steps Approach To AI Implementation (according to Andreas Simon)
Step 1. Data Modeling & Specification involves:
- Data needs to be made available to the AI team.
- Specification of what exactly needs to be predicted (for instance, measures, periods).
- Kickoff with Customer Data Experts to analyse which data is available and which (additional) data is needed to reach specification goals.
Step 2. Data Analysis requires:
- Data Analysis of the available data regarding the prediction goals (e.g. missing values, grouped data, new/outdated data).
- Kickoff with Customer Data Experts to analyse if the data decision is valid.
Step 3. Design Decision means:
- Testing available data with different approaches (e.g. Time Series & Driver Based approach).
- Decide which approach to take for the specified data sets depending on the first results with given data sets. Possible re-iteration if data sources are not sufficient.
- Milestone: decision on which prediction model should be used in the Implementation phase.
Step 4. Design Implementation is focused on:
- Using a specified approach on all available data sets, adapt parameters.
- Adapt approaches to different data scenarios to reach maximum accuracy for each data set.
Step 5. Test & Refine involves:
- Test approaches in different scenarios.
- Refine approaches to reach the optimal output.
- Possible re-iteration if data sources or prediction models are not sufficient.
Step 6. Production means applying results to the production level.
In addition to providing us with a step-by-step guide, Andreas shared a practical case study of a hotel chain. This approach was implemented and resulted in a forecast accuracy of over 95%.
Conclusions
AI and ML technologies improve our budgeting, planning, and forecasting efficiency and accuracy. But before we can enjoy such a wonderful result, we must invest time and effort to build these models. This can only be achieved through a long process of trial and error.
ML implementation is a marathon, not a sprint. Don't quit this race midway.
We would like to thank our global sponsor, Jedox, for their great support with this FP&A Circle.