Forecasting new product launches are a tricky business with plenty of emotional baggage. They are also...
In a previous blog, I wrote about how forecasting the outcome of new product launch was almost a mission impossible and that Finance professionals would be better off focusing on creating a flexible model, able to cope with new and changing information. In this blog I take a broader view of new products and talk about how best to monitor progress post-launch when information is still a little sketchy, volumes are still very low and reporting mechanisms may not yet be fully in place.
Embrace imperfection
First and foremost, Finance need to accept that new product data may well be limited. Regardless of how the situation has come about, it is far better to have imperfect data than none at all. For example, consider using:
- Infrequent, irregular data, not necessarily monthly
- Manually sourced data. Whilst never ideal from a reporting perspective in the absence of system information a pragmatic approach is needed.
- Data that requires manual allocations, assumptions or adjustments
- Data sourced externally, not necessarily easily verifiable.
The best way to mitigate against any concerns or resistance to such an approach is to clearly communicate what you are doing and document key methodologies in arriving at your dataset.
Take a balanced scorecard approach. Whilst in a previous blog I focused on how to improve the scorecard process, here I want to emphasise the value of non-financial metrics, which should be embraced, particularly those that are considered to be lead indicators.
Often the most helpful lead indicators are used by the sales teams, so it is worth having a dialogue with them to understand what data could be extracted from their pipeline & CRM tools. The same applies in terms of qualitative feedback and how that trends over time.
Create a nursery area
Nurseries are safe environments where your kids learn and play – and you need the same for new products. You need a reporting mechanism outside of the regular monthly P&L management pack that focuses solely on new products and where different rules apply. By different rules I mean:
- There may not be a budget, instead, there may be a business case, or multiple business cases that mix OPEX and perhaps even still some CAPEX
- There will be a range of metrics, not necessarily consistent across new products. It will depend on what is readily available.
- Mistakes with the data happen. If data is scarce, difficult to produce and subject to manual intervention it's inevitable there will be mistakes and a need for restatements.
Remind everyone of the benefits of Finance
Given the points above you could be forgiven for thinking that I am advocating a ‘slap-dash’ approach to the management reporting of new products. I’m not! I’m advocating that Finance own the reporting ‘space’ for new products because of the inherent discipline that is part of our professional training.
It is therefore important to remind the organisation that whilst a highly pragmatic approach is being taken to source data & insight, Finance is
- Still an impartial user of data, with no hidden agenda (and most likely an impartial repository of business cases)
- Accustomed to bringing discipline to a reporting process
- Skilled at interrogating data, including its veracity
- Able to leverage insights from the broader monthly P&L and scorecard packs.
Concluding takeaway – A beg, borrow, steal mindset is needed
Understanding the progress of a new product launch is critical to shaping its success and often data to help with that can be thin on the ground. Finance have a key role to play here, and can add value if they are prepared to take a slightly different approach from the one used to deliver the usual monthly management information pack.
Being creative about where data is sourced is key, covering both financial and non-financial aspects of the product. Also creating a dedicated medium for delivering insights is a helpful way in managing expectations about how ‘imperfect’ data should be used and interpreted.
The article was first published in Unit 4 Prevero Blog
Subscribe to
FP&A Trends Digest
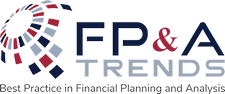
We will regularly update you on the latest trends and developments in FP&A. Take the opportunity to have articles written by finance thought leaders delivered directly to your inbox; watch compelling webinars; connect with like-minded professionals; and become a part of our global community.