In our earlier blogs we have discussed the role of data in your profession, your main...
According to a recent survey by Prophix and FP&A trends, 88% of companies claim they have data quality issues [1]. The aim of this blog is to sketch the main steps that you can take to ensure that your company belongs to the remaining 12%.
Think what quality of your data should be
In one of the previous blog, we concluded that ‘right’ data is data that fits your purpose. But is the data of good quality? And again, what is a ‘good’ of ‘acceptable’ level of data quality and how to measure it?
Each data might require its level of quality. You can’t organize online sales services without knowing a delivery address. In this case, each customer must have a delivery address. At the same time, you might survive without knowing their marital status. You manage getting required data by setting your data quality requirements. You do it by using different data quality dimensions. The most common dimensions are data accuracy, completeness, correctness, timeliness, etc. These dimensions allow you to build data quality checks and measure the quality of the data. You measure data quality by comparing the requirements set up by data with the real outcomes.
Step 1: Define your critical data
If you have read ‘Big and small steps to get the right data to right people at the right place and time’ [link blog 4] and followed my advice, you might have completed the analysis of your main reports and discovered your critical data elements. You should take into account that each department probably has their own vision on what is ‘critical’. For example, for a sales department it could be a customer or product profitability. Or you could recognize ROI as critical data. I once talked to specialists from a large international company about this. They told me they have counted more than 1500 critical elements. Now it is your turn!
Step 2: Identify and deliver your data quality requirements
When it comes to setting up your data requirements, you should:
- clarify data definitions;
- stipulate the purpose of data usage;
- define data quality requirements.
Now it is time to define your data quality requirements.
Being a data user, you are accountable for defining your data quality requirements and delivering them to data owners. Also, you need to check whether data quality checks have been set up and if so, whether they match your requirements. If all is done, from now on, you and your colleagues should never have to clean incoming data again.
Step 3: Recognize the difference between data cleansing and data quality framework
To avoid any misunderstandings, let us specify what unites and what separates these two terms. They both relate to the improvement of your data quality. They might even relate to the same techniques and tools for detecting problematic data.
These are the similarities. Now, what about the differences?
Data cleansing is mainly a one-off exercise to detect and to correct corrupt and incorrect data records. It can take some time, once I have heard about a cleansing project in a company that took almost two years.
Data quality framework is a preventive way to ensure data of good quality in long-term perspective.
You could choose to do both, but you might also simply concentrate your efforts on a long-term sustainable solution. Let’s see how to do it.
Step 4: Build a data quality framework
Finance and business planning departments are the main stakeholders with huge concerns about data. To implement the framework, you need to involve a lot of different departments. Financial top executives might take the role of a sponsor for this initiative. It is worth mentioning the main elements you need to foresee:
1. Designing a governance structure.
This structure includes setting up policies and procedures. Then you need to identify roles and their accountabilities regarding data quality. Incident management is a part of this frame.
2. Setting up a central issues log catalogue.
Here, all parties involved will need to register their data quality issues.
3. Solving the most important data quality issues.
Data quality issues can be of different nature and of different importance. The manner of how you will solve them depends on the size of your company and the preferred way of working. You can organize some projects around the most critical issues. You can create working groups that will include both business and IT professionals. You might also start a company-wide program.
4. Knowing and documenting the path that data travels from its origin to end-users.
Before you can start solving the issues, some solid preliminary work needs to be done. It includes gathering knowledge about what your data is, where it is located, and how it flows from its origin to the end-users. Without having clear answers to these questions, your efforts to improve data quality will be fruitless.
5. Organizing your master data, especially customer data.
One of the important preconditions of successful data quality management is bringing in order your master data. The most known examples of master data are customer and product data. Almost every company now is in the process of cleaning their customer data. In Europe, it is partly caused by the GDPR requirements set up by the European Union.
6. Identifying the ‘golden’ sources.
The next important precondition is the identification of the ‘golden sources’ of data. A ‘golden source’ is an application where trustworthy data has been initially administrated and maintained. All other systems use the same data from this one source. There are a lot of companies that might keep the same customer information in different systems simultaneously. Discrepancies in information are almost unavoidable in this situation. For example, different systems could have different information on a customer’s address, if they are not synchronized or updated with the same frequency.
7. Identifying and implementing data quality checks and controls.
My last tip is simply reminding you about the necessity to define and implement data quality checks and controls on the whole way of data flow.
You can get more detailed information on setting data management and data quality framework in The Data Management Cookbook. Now it is your turn to start a journey to bringing the quality of your data to the required level.
In my next blog will be devoted to new concepts around the usage of data as big data and data science and its application to finance and business planning function.
Notes
[1] ‘Defining the Evolution of FP&A: Benchmarks, Challenges and Opportunities‘, by Prophix and FP&A Trends, p.12.
The article was first published in Unit 4 Prevero Blog
Subscribe to
FP&A Trends Digest
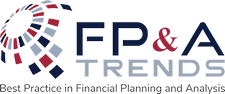
We will regularly update you on the latest trends and developments in FP&A. Take the opportunity to have articles written by finance thought leaders delivered directly to your inbox; watch compelling webinars; connect with like-minded professionals; and become a part of our global community.