The Financial Modeling Landscape Is Changing And So Are The Demands Put Upon Business Analysts.
Federal guidance, spurred by the great recession, enhanced IT capabilities, the need for greater security, an increased acceptance of financial theory and the overall pursuit of best practice have all elevated the level of detail and precision which financial modeling in the business world has pursued.
Learning Excel, a widely used and accepted computer application is one essential skill required of a strong financial modeler. But these days, the business world requires more:
1. An understanding of modern model governance standards and management,
2. The FP&A analyst gold standard of developing fully integrated forecast financial statements as opposed to simply ad hoc spreadsheet analysis, and
3. Knowledge of proper validation and monitoring techniques.
This article addresses those needs.
Many types of modeling approaches exist. This article focuses on forecasting an integrated set of financial statements, line item by line item, using what is called a “deterministic disaggregated component” approach.
To the corporate insider, this type of forecast model is invaluable. CEOs, CFOs, business planning, treasury and investor relations all rely heavily on forecast statements, line by line. Such forecasting is used to monitor departments and business units and key figures are often communicated to outside investors and the general public.
Industry analysts too use the same deterministic forecasting approach to model financial statements and subsequent valuations.
Large companies can forecast with remarkable accuracy. Often, tolerance levels from budget are as low as 3-5%. If we were simply buying a stock as in investor, other approaches may suffice with less effort. Here we are not – we are managing an entire company.
1.What is a Model?
2.Introduction to Model Governance
3.Best Practice Modeling for Excel
4.Forecast Statement Analysis and Design
5.Validation and Acceptance
6.Additional Resources
1. What is a Model?
Federal Reserve issued guidance to banks (SR 11-7 among other documents) defined a model for the purpose of model risk management after the wake of the great recession in 2008. It provides a framework for best in class financial model development, validation and monitoring.
SR 11-7 outlines six criteria a financial tool must possess to be a model.
1.The analysis must employ academic theory
2.Three components: inputs-calculation processes-outputs
3.The analysis must transform data into useful business information
4.Must be used repetitively
5.Output must be quantitative in nature
6.Subject matter expertise is required
7.Possible qualitative model inputs or output
Models are not limited to one programming language environment or application. The term “model” should be viewed “wing to wing” from initial data inputs to final model output. Numerous programming environments may be involved as with numerous data sources. “Downstream” models should be identified.
What Are Corporate Finance Models Used For?
Financial models inform decision makers and also assist in complying with regulatory requirement. The three primary corporate finance activities are:
1. Investment Decisions – estimating and comparing cash flows, risks and returns to requirements and expectations.
2. Financing Decisions – determining capital structure; what type of instruments will we use to finance the assets, what are the terms of this financing (e.g. length, terms) and to what degree can each asset be levered?
3. Dividend Policy – should we invest for growth and replacement or should we dividend cash flows to investors and what are investor’s expectations?
Influencing Model Factors
Models improve in integrity and effectiveness as the result of several guiding influences.
2. Introduction to Model Governance
Model Governance concerns itself with:
A.Identifying models
B.Developing internal policy and procedure
C.Developing and enforcing model standards
D.Interpreting and abiding by regulatory guidance and rule
E.Researching and identifying best practice
F.Ensuring proper documentation
G.Assisting in tracking models and ensuring proper remediation when required
H.Overseeing model testing
I.Risk management
J.Change management
Model Risk Management
Virtually each of the activities mentioned in this article relate to development and subsequent model risk management. Model risk refers to the chance of unintended consequences resulting from model development, inputs or outputs.
The Model Lifecycle
Models follow a what is called the “model lifecycle”. The model lifecycle has four components. In order, they are:
Model Participants
There are numerous players and business units involved through the model lifecycle. They include:
Model Developers. Those develop the model using systems, theories, formulas and data.
Model Owners. Those responsible for model use in “real time”.
Model Users. Those who implement the model and its output.
Model Testers. Usually IT, who test model development results and manage model change.
Model Validators. Those who confirm a model is working as intended, often using mathematical approaches.
Business Units. Business Units implementing the model and utilizing the model output.
Model Governance. Those who see that models are tracked, implementing monitored, inventoried and comply to standards.
Risk Management. Those who monitor and manage the risk element of a business’s activities.
Compliance. Those who confirm activities meet regulatory requirements.
Audit. A last internal line of defense.
Model Standards
Here is one set of standard topics for non-regulated corporate finance models. These ensure ownership, improves accuracy and reduces key person dependence. Regulatory requirement may come into play as well for certain financial institutions which ups the ante.
Model Documentation
Good documentation is a key component to model risk management and should be viewed as such and not simply a compliance and requirement exercise. Advisory bulletin 2013-07 supports this assertion and adds that documents should be the responsibility of the model developer (development) and owner (operating procedures, ongoing monitoring), thereby providing a first line of defense and control between model developers, users and model owners. Proper documentation is cumbersome and takes time, but it is critical to a model’s overall success by reducing risks and costs. Benefits include:
- Reduced key person dependence
- Mitigated transitional challenges as models pass from user to user and output need to output need as conditions and requirements change
- A more streamlined validation and audit process
- Reduction in potential user error
- Avoidance of potential misuse
- Effective communication of challenging theoretical concepts – “comfort”
The documentation of financial models is a critical element of model risk management. It behooves developers, users and owners to spend the time and effort to properly document a model through its life cycle. Documentation, though time consuming and cumbersome, aids in validation, monitoring and audit and provides a platform for model developers to effectively communicate their model and its conceptual soundness to all areas of an institution, from data downloaders to executive management. Federal agencies will look favorably upon models which have comprehensive and well-conceived documents to support their models.
Model Flow Charting
Flow charts are powerful tools. Flow charts can come in many forms and there is no one exact manner to flow a model. From my experience, two flow charts stand out as invaluable;
1. System flow chart “wing to wing” (input to output)
2. Development flow chart
A system flow chart will show, left to right, a model’s data inputs and IT/business unit environments, calculation processes, and model output and IT/business unit environments. A development flow chart will visualize the exact mathematical methodologies and techniques employed to transform input data into useful business information and will generally focus on only one business environment.
Flow charting financial statement builds provide the user a quick gauge on what data is at hand versus what is needed to complete a model. A flow chart will help identify the required builds and environments from which data will be acquired. Flow charting will also help visualize core components within and across models and are an ideal medium for identifying and communicating risk and control points. Flow charts will dramatically improve model buy-in and provide a path for solid structure. Dead ends, duplicity and unmanageable audit trails now become visual.
Model Definitions
Here are some great definitions to know:
Back-test. Use of historic data as a test to model output validity.
Benchmark. The comparison of model output to the output of an outside and independent source.
Emerging Risk. Unforeseeable risk arising further in time and model execution.
FAST. Set of rules for financial model design. Flexible, Appropriate, Structured and Transparent.
Impact Analysis. Assessment of cost, timing, scope and quality of a model-consequence.
In-Sample. Historical data used in model development.
Model. Quantitative method, system, or approach that applies statistical, economic, financial, or mathematical theories, techniques, and assumptions to process input data into quantitative estimates.
Out of Sample. Historical data not used in model development.
Outcomes Analysis. The comparison of model output to actual outcomes. Back-testing is one example.
Parameter. Numerical characteristic of a set or population of numbers.
Calibration. Adjustment of data and assumptions.
Residual Risk. Remaining risk after a risk mitigation action has been performed.
Risk Appetite. Largest tolerable degree of uncertainty acceptable.
Scenario. Multiple changes to inputs to reflect a given set of circumstances.
Secondary Risk. Risk arising from a risk response.
Sensitivity. Impact of a change to an input relative to the change in output.
Stress Test. Assessment of model stability by employing hypothetical data inputs or drivers.
Threshold. Measure of uncertainty or impact worthy of attention.
Tolerance. Degree of deviation within which a model still functions properly.
Validation. A set of processes and activities intended to verify that a model performs as intended and as expected.
3. Best Practice Modeling for Excel
Numerous organizations have developed modeling rules for the use of Excel. These rules provide structure and commonality among spreadsheet design and use. Many rules and guidelines can apply to other languages as well. Technically, Excel is an application and not a language. And it is only one of many possible languages and applications which may be incorporated into a model. Due to its wide-spread use and applicability to recent college graduates, this section focuses on best practice for Excel.
Excel Standards Organizations
- FAST (Flexible, Appropriate, Structured, Transparent)
- Smart Financial Modeling
- BPM (Best Practice Modeling)
Common Rule Categories
- Workbook Design
- Worksheet Design
- Line Item Design
- Cell Design and Use
- Assumption Design and Use
- Use of Features
- Formatting Rules
The purpose of these rules is to provide clarity, accuracy, safety and a clear audit trail. Only create work which you would gladly show on a blackboard in a court of law.
Color Use color protocol Use color sparingly Inputs are blue Calcs are black External links are green Trouble spots are red |
Saving and Printing Use naming protocol Use saving protocol Print format for all pages Print then proof, never off the screen only |
When creating a model, you are telling a story. As the FAST standards say, you are really writing sentences, paragraphs and chapters. That approach will help get your model “read” and more easily accepted. Models are not political tools.
Brevity Keep formulas short Assumptions shown on same page as calc Keep audit trails short Avoid daisy chains Never replicate math, except as proofs Avoid external links, use exported tabs instead Highlight external links when required to use Avoid circular references when possible |
Rows, Columns and Tabs One column, one purpose One row, one purpose No line or column breaks between calculations Avoid merging cells Forecast periods move left to right Statements move top to bottom No breaks between rolling forecast periods Don’t hide rows or columns Tabs flow left to right Use summary sheets, clean of build ups |
Formulas, Calculations and Functions Use statement accounting sign convention as you would read from a 10K Subtotals presented below Use balance and sanity checks Show all math Anchor repetitive calcs left to right and top to bottom Macros should be used sparingly Avoid named ranges Create dedicated calculation areas Anchor “drivers” |
Common Statement Rules
Here are some time tested financial statement techniques and rules of thumb:
Balance Sheet. Always balances.
Working Capital. A function of operations with few exceptions such as the current portion of long term debt.
Debt. Built from asset’s ability to obtain financing, possibly drawn down to zero or maintained at a certain debt to equity ratio.
Debt: Equity. Acts as the “keel” to the balance sheet.
Equity. Cash is not run up, dividends are used to balance assets and liabilities plus equity.
Cash flow. A function of balance sheet and income statement. Often produces a “hypothetical” dividend, as companies may choose retain excess cash as opposed to issue a dividend.
4. Forecast Statement Design and Analysis
Five key approaches to a sound forecast model:
1. Integrate Statements
2. Utilize Quantitative Forecast Techniques
3. Roll - Period to Period and Model to Model
4. Use Expected Values
5. Validate Results
Types of Models
Deterministic models use assumptions (independent variables) which are believed to be “known”, that is they are static. Run a deterministic model ten times and you will get the same result.
Stochastic models use assumptions (independent variables) which are only believed to be “known”, within a set of parameters or “boundaries”. Run a stochastic model ten times and you will get ten different results. Dependent variable output therefore has a random element to it. Models are often then run using Monte Carlo simulation for numerous results and an average of these results is used as the final model output.
Deterministic Forecast Modeling
Stochastic models have a distribution of potential outcomes since they allow for random variations in one or more inputs. The random variation is usually based on fluctuations observed in historical data for a chosen period using standard time-series techniques. In other words, assumptions are not precisely known and may vary through time.
If possible, see if your model can combine both types of models. Key assumptions developed through stochastic processes incorporated into a deterministic model could provide the best of both worlds.
Other Model Classifications
Extrapolative Modeling utilizes prior period results in time series fashion, year over year growth, for example.
Index Modeling utilizes outside data to estimate future internal results.
Disaggregated Modeling uses disparate data (line items on financial information, for example) to estimate future outcomes.
Forecast Statement Development
Forecast models do not produce guesses, but rather estimations. They are developed using real world observations (often actuals) and may be modified as necessary. Fortunately, fundamental statement analysis provides a perfect framework through the use of common financial statement metrics and observations. Some observations may be internal (last year’s sales, number of customer, and some may be external (GDP growth, housing starts, as examples).
Metrics will drive certain line items on each financial statement, which in turn, may drive other financial statement’s line items using integrated modeling techniques. Interest expense as a percentage of debt is an example.
Other ratios can be used to assess performance and judge the validity of forecast results.
Integrating Statements
Both conceptually and mechanically, integrated statements, by acknowledging the balance sheet-income statement-cash flow interdependency, demonstrate a core understanding of corporate finance synthesis and provide a foundation for both accounting and modeling structure. This is why the investment banks and Big Four consulting firms go to great lengths to develop this skill in their incoming analysts. Without integrated statements forecasts, accompanying DCFs are likely to not hold upon to scrutiny.
Integrated statements can create circular references such as this:
- Interest expense on the income statement is a function of an average debt balance on the balance sheet.
- Current net income is dependent upon interest expense.
- Balance sheet equity is dependent upon current period net income.
- Debt is dependent upon total capitalization
- Total capitalization is dependent upon equity.
Though conceptually correct, both modeling organizations FAST and Best Practice Modeling recommend avoiding circularity. Circular references within circular references can trip up Excel (yes, I have seen it happen). This can be solved by using a goal seek macro, for instance, maintaining a debt to equity level through all model periods.
However this is handled, the best integrating approach will reflect the actual management approach employed in the organization.
Common Quantitative Forecast Techniques
Budgeting and forecasting methods can be divided into two broad categories: qualitative and quantitative. Listed below are common quantitative forecast techniques for financial statement modeling.
1.Time series change (price, for example)
2.Causal relationships (cash and receivables as a % of sales)
3.Naïve - Beginning balances equaling prior period ending balances or flat-lined (fixed assets, debt)
Qualitative techniques may be used to adjust quantitative forecast output, based upon subject matter expertise.
Time Series
A time series is just a set of observations measured at successive points in time or over successive periods of time. Time series uses past trends of a variable.
Cyclical
Any recurring sequence of points above and below the trend line that last more than a year is considered to constitute the cyclical component of the time series. Example; while the trend line for gross domestic product (GDP) is upward sloping, the output growth displays a cyclical behavior around the trend line.
Seasonal
Seasonal components capture the regular pattern of variability in the time series within one-year periods. Many economic variables display seasonal patterns. Seasonal will require you to forecast by quarter and/or month.
Calendar
Similar to seasonal, but follows a calendar path, such as weeks or quarters (Q1, Q2, Q3, Q4).
Time Series Refinements
Moving
The term "moving" refers to the way averages are calculated—the forecaster moves through the time series to pick observations to calculate using an average of a fixed number of observations. In calculating moving averages to generate forecasts, the forecaster may experiment with different-length moving averages. She will choose the length that yields the highest accuracy for the forecasts generated. Weights may be assigned to time periods.
Casual
With causal relationships, analysts examine the cause-and-effect relationships of a variable with other relevant variables such as the level of consumer confidence, an income statement or balance sheet item. Below are examples of common causal relation calculations:
1. Position calculations represent a company's financial position regarding earnings, cash flow, assets or capitalization. Calculations can be expressed as a dollar amount, a percentage, or a comparison. Position calculations are often referred to as “common-sized” when it is uniformly applied to a whole statement.
- Cash % Assets
- Debt / Equity
2. Metric calculations assess financial position relative to a non-financial figure such as days, transactions or number of customers.
- Transaction Figures (Units Sold Per Day, Transaction Days, etc.)
- Utilization %
A widely-known causal method is regression analysis, a technique used to develop a mathematical model showing how a set of variables are related. Regression analysis that employs one dependent variable and approximates the relationship between these two by a straight line is called a simple linear regression. Regression analysis that uses two or more independent variables to forecast values of the dependent variable is called a multiple regression analysis.
Causal Metrics (Drivers)
Here are some well used financial statement metrics used to develop line items for forecasts:
Assets
- Cash % of revenue
- Cash % of assets
- Inventory % of sales
- Accounts receivable % of sales
- Accounts receivable based on days outstanding
- Short term securities % of debt
- Short term securities % of cash
- Depreciation and amortization function of first cost
Liabilities
- Payables % of cost of goods sold
- Debt function of D:E target
- Dividend result of D:E target
Income Statement
- Revenue = price x volume
- Cost of goods sold % of revenue
- Selling expenses % of revenue
- Depreciation function of first cost of asset
- Interest expense % of average debt balance
- Taxes at tax rate
Time Series Metrics (Drivers)
- Price
- Volume
- Cost of Goods Sold (PxV)
- SG&A
- Non-recurring and one-time expenses such as legal fees
Other considerations
Roll the Model Period to Period
Rolling the model means allowing time series drivers to work directly off of the prior period balances. Furthermore, ending balance sheet item balances should flow directly to the next period’s beginning balance. Try developing your model’s statements using these line items first, then move to causal line items. Never cut paste special unless importing data.
Use Expected Values
Though asset capacity and elasticity constraints (utilization rates) usually prevent symmetrical distribution of outcomes from occurring in real life, as a planning and forecast tool, forecasts most often assume a bell-shaped curve. In such a symmetrical distribution, the most likely outcome is equal to the probability weighted outcome. This will provide compatibility to most downstream models such as valuation models which most often are built upon the assumption of expectation. Expected values are not shaded by optimism, pessimism or hope.
Assumption Examples
Table 1
Table 2
Table 3
Table 4
Table 5
Table 6
5. Validation and Acceptance
Model Validation Techniques
Validation of model results can be performed several ways:
Back-testing – Use of historic data as a test to model output validity. This is also a great way to develop a model. Simply build your model using historic data and solve for a known answer.
Benchmarking – As previously discussed, this verifies your model’s output by comparing it to another source, either internal or external.
Scenario Analysis – Run your model using various inputs and assumptions to test for model integrity and reasonableness.
Sensitivity Analysis – Vary inputs and assumptions to compare against other input and assumption changes to see if a proper correlation exists in model output.
Use Test – Has the model stood the test of time?
Common Validation Ratios
These ratios provide sanity checks for validation. Is model output reasonable and does it follow accepted patterns? Ratios not only reflect the financial position of a firm but may also reflect a model’s calculation abilities and errors.
These metrics may also help verify inputs and assumptions and also validate a model’s results:
Validation Benchmarks
Model output can be compared to numerous benchmarks. They include:
- Prior performance
- Expectation
- Competitor results
- Industry standards
- Analyst forecasts
- Internal consensus
Sensitivity Analysis
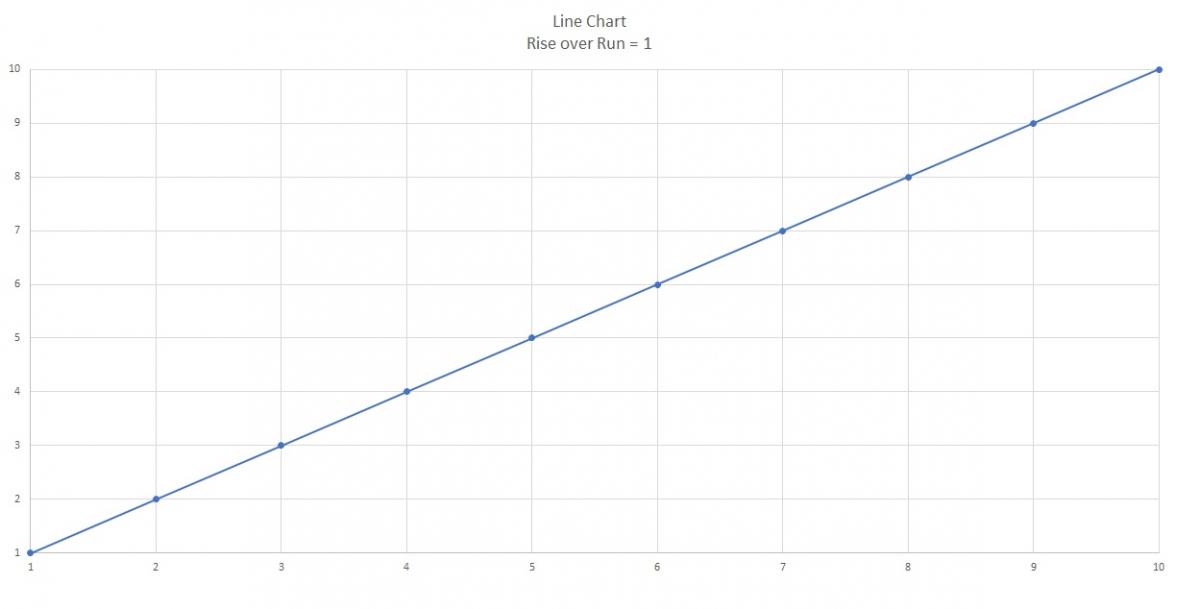
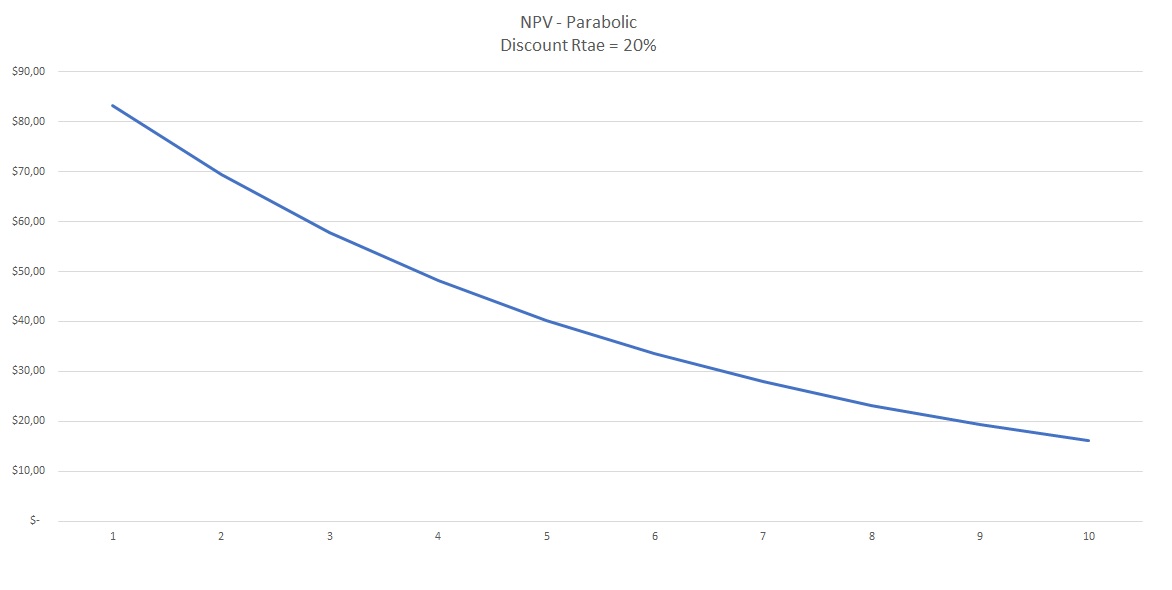
Back-testing
Assuming we use a three year average to forecast accounts receivable for the year 2018. Is our result consistent with out of sample actuals at a tolerable level? Here, it appears so.
Validation Trends
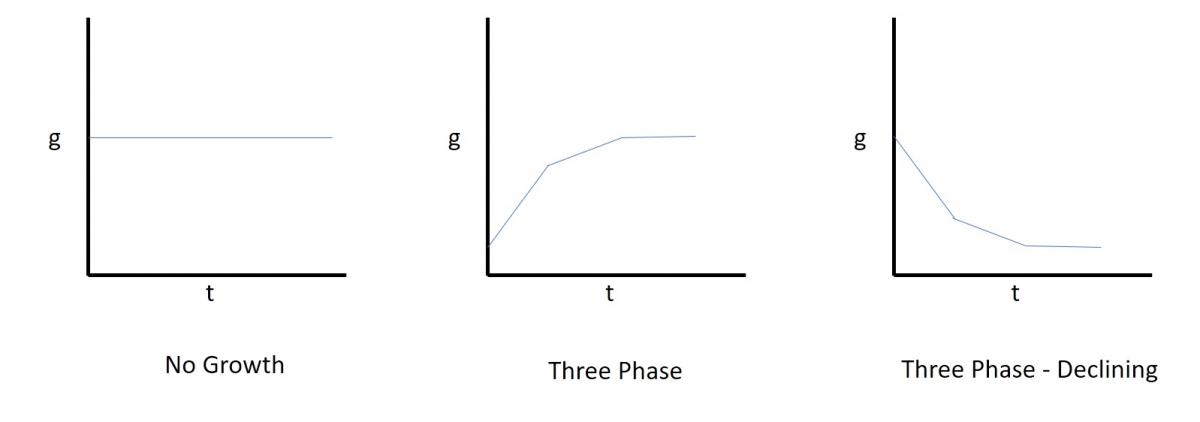
Ongoing Monitoring
Ongoing monitoring plays a vital role in model integrity. Are models bask-tested annually? What business conditions and/or data sources have changed? What external factors may have changed affecting the model’s relevancy? Is the regulatory environment adopting new rules and standards?
Model Acceptance
- Does your model comply to accepted model development or are they simply proprietary? (FAST standards)
- Was the model developed with “management buy-in?”
- Do key model users buy into the theory behind the model? If not, should the model attempt to better support the theory driving model calculations?
- Does model development theory still hold?
- Does model output “marry” to downstream use? If not, how can this be improved?
- What approval processes are in place for the ongoing use and approval of the model?
- Does the data input and model final output remain relevant?
6. Additional Resources
Here are links to model documentation and other guidance:
The Global Association of Risk Professional has numerous articles on model risk and validation.